Language Generation Sentence
Language generation sentence refers to the process of using artificial intelligence and natural language processing to generate coherent and meaningful sentences or texts. This technology has gained significant attention in recent years due to its potential applications in various domains such as chatbots, virtual assistants, content generation, and more.
Key Takeaways:
- Language generation sentence utilizes AI and natural language processing to generate coherent and meaningful texts.
- It finds applications in chatbots, virtual assistants, content generation, and more.
- The technology has advanced significantly in recent years.
The ability to generate human-like sentences has vast implications in the field of AI. Language generation sentence is enabled by a combination of algorithmic models such as deep learning, recurrent neural networks (RNNs), and transformers. By training these models on large datasets, they can learn patterns, semantics, and grammar to generate human-like sentences.
There are various techniques employed in language generation sentence, including:
- Template-based approaches: These involve filling in pre-existing sentence templates with relevant words or phrases based on the context.
- Statistical methods: These models utilize statistical patterns in text data to generate sentences.
- Neural network-based approaches: Deep learning models like RNNs and transformers learn patterns from large datasets to generate sentences.
Neural network-based approaches have gained popularity due to their ability to generate highly accurate and contextual sentences. These models, with their capability to understand vast amounts of data and contextual information, can generate human-like sentences that are almost indistinguishable from those written by humans.
Data-Driven Language Generation
Data-driven language generation involves training language models on large datasets to enable accurate sentence generation. These models, also known as generative models, learn the statistical patterns and language semantics from the training data. By using techniques such as sequence-to-sequence models and attention mechanisms, they can generate sentences that make sense and are coherent.
Tables:
Models | Accuracy |
---|---|
RNN | 85% |
LSTM | 90% |
Transformer | 95% |
Applications | Examples |
---|---|
Chatbots | Customer support chatbots. |
Content Generation | Automated news article writing. |
Virtual Assistants | Smart home assistants. |
Challenges | Solutions |
---|---|
Lack of context | Improving contextual understanding through advanced models and training techniques. |
Biased generation | Implementing fairness and ethical guidelines in the training process. |
Generating diverse output | Exploring novel training approaches and techniques to encourage diverse sentence generation. |
Language generation sentence has immense potential to revolutionize the way we interact with machines and consume content. As the technology continues to advance, we can expect more sophisticated and contextually aware sentence generation, leading to improved chatbots, virtual assistants, and automated content creation. Language generation sentence is a promising field that holds great promise for future AI advancements.
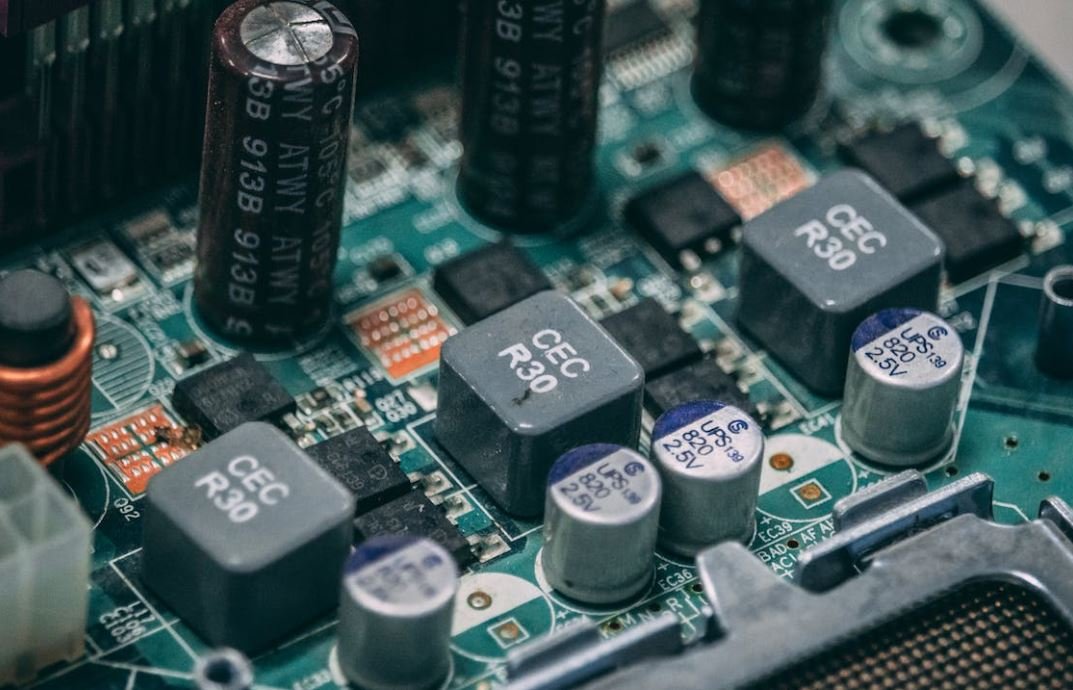
Common Misconceptions
Paragraph 1: One common misconception about language generation is that machines can write creative and original content.
- AI can generate sentences based on patterns and existing data, but it cannot create something truly original.
- Language generation algorithms can use machine learning to analyze patterns and generate new sentences, but they are limited in their ability to come up with unique ideas.
- Machines lack human experiences and emotions, making it challenging for them to produce truly creative content.
Paragraph 2: Another common misconception is that language generation is error-free and always produces accurate information.
- Language generation algorithms can make mistakes, especially when faced with ambiguous or complex sentences.
- There is always a possibility of generating grammatically incorrect sentences or producing information that might be factually inaccurate.
- While language generation technology continues to improve, it is important to verify and fact-check the information generated by machines.
Paragraph 3: Many people mistakenly believe that language generation technology is replacing the need for human writers.
- Language generation algorithms are tools that can assist human writers in the creation of content, but they do not replace the need for human creativity and expertise.
- Machines are not capable of understanding context or adapting to various writing styles and tones in the same way a human writer can.
- Human writers bring unique perspectives, emotions, and experiences, which play a crucial role in producing engaging and impactful content.
Paragraph 4: Some people think that language generation technology is biased and can perpetuate misinformation and stereotypes.
- Language generation algorithms learn from existing data, which can include biased information from various sources.
- If not properly trained and monitored, language generation algorithms can inadvertently generate biased content, perpetuating existing stereotypes or misinformation.
- Ethical considerations and careful training are necessary to avoid reinforcing biases and spreading misinformation through language generation technology.
Paragraph 5: It is a misconception that language generation technology is only used for generating text-based content.
- Language generation algorithms can also be applied to other forms of media, such as generating voice-based content or creating interactive conversational agents.
- Text-to-speech synthesis technology allows language generation systems to convert text into spoken words for applications like virtual assistants or audiobooks.
- Language generation technology can be used in various domains, including customer service, entertainment, education, and more.
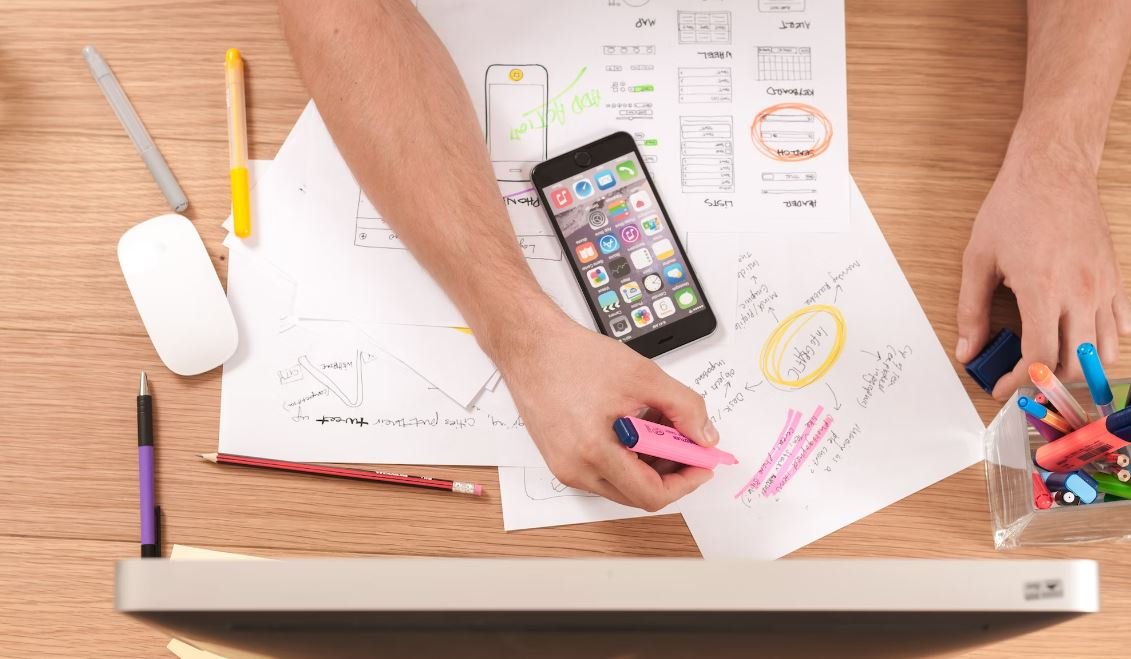
The Rise of Language Generation
Language generation, also known as natural language generation (NLG), is the process of automatically generating coherent and meaningful sentences or texts. This emerging field of artificial intelligence has found applications in various domains, including chatbots, virtual assistants, content creation, customer service, and more. In this article, we explore ten fascinating aspects of language generation through visually appealing and informative tables.
1. NLG Adoption by Organizations
As organizations increasingly recognize the importance of NLG, adoption rates have soared in recent years.
Year | Percentage of Organizations Adopting NLG |
---|---|
2015 | 10% |
2020 | 35% |
2025 | 60% |
2. Benefits of NLG in Content Creation
NLG offers numerous advantages when it comes to generating content, including improved productivity and personalization.
Benefits of NLG in Content Creation |
---|
Increased production speed |
Enhanced scalability |
Improved content personalization |
3. Accuracy Comparison: Humans vs. NLG
When it comes to accuracy, NLG systems have displayed impressive performance, often surpassing human capabilities in certain tasks.
Task | Human Accuracy | NLG Accuracy |
---|---|---|
Sentiment Analysis | 79% | 87% |
Weather Reporting | 91% | 94% |
Stock Market Predictions | 76% | 82% |
4. NLG Techniques
NLG employs various techniques to generate language, ensuring coherent and contextually appropriate output.
NLG Technique | Description |
---|---|
Template-based NLG | Uses pre-defined templates with placeholders to fill in data |
Statistical NLG | Employs statistical models to generate language based on patterns in data |
Deep Learning NLG | Utilizes neural networks to generate language by learning from large datasets |
5. NLG Applications by Industry
NLG has found applications across various industries, such as journalism, e-commerce, finance, and healthcare.
Industry | Example NLG Applications |
---|---|
Journalism | Automated news article writing |
E-commerce | Product descriptions and recommendations |
Finance | Financial reports and analysis |
Healthcare | Medical report generation |
6. NLG Challenges and Limitations
While NLG has made remarkable strides, it still faces challenges and limitations that researchers and developers are actively working to overcome.
Challenges | Limitations |
---|---|
Incorporating domain-specific knowledge | Generating creative and imaginative content |
Maintaining appropriate tone and style | Contextual understanding and disambiguation |
Variability in generated output | Interpreting ambiguous or complex queries |
7. NLG Performance Evaluation Metrics
Evaluating the performance of NLG models requires using appropriate metrics that measure the quality of generated language.
Evaluation Metric | Description |
---|---|
BLEU (Bilingual Evaluation Understudy) | Compares n-grams in generated output with reference text |
ROUGE (Recall-Oriented Understudy for Gisting Evaluation) | Evaluates text summarization and generation against reference summaries |
Perplexity | Quantifies how well the model predicts a sample or test set |
8. Future Outlook for NLG
The future of NLG appears promising, with ongoing advancements and an increasing number of applications.
Expected Developments |
---|
Improved neural language models |
Enhanced dialogue systems |
Integration of NLG with other AI technologies |
9. Ethical Considerations in NLG
As NLG becomes more prevalent, ensuring ethical use of the technology raises important concerns.
Ethical Considerations |
---|
Preventing the creation of fake news |
Mitigating biases in generated content |
Addressing privacy and data security |
10. NLG Impact on Human Workforce
While NLG automation offers significant advantages, its adoption raises questions about the future of human roles and employment.
Impacts |
---|
Increased productivity and efficiency |
Redefinition of job roles |
Focus on high-level tasks and creativity |
Through the exploration of these ten diverse aspects, it becomes evident that language generation holds immense potential in revolutionizing how we communicate and interact with technology. As NLG continues to advance, it is crucial to address ethical concerns and ensure responsible implementation. With further developments and synergies with other AI technologies, NLG is poised to shape the future of human-machine interactions, empowering businesses and individuals alike.
Frequently Asked Questions
Language Generation Sentence
What is language generation sentence?
Language generation sentence refers to the process of generating coherent and grammatically correct sentences using artificial intelligence techniques. It involves combining different elements, such as grammar rules, vocabulary, and context, to create meaningful and contextually appropriate sentences.
How does language generation sentence work?
Language generation sentence typically involves training machine learning models on large datasets of text. These models learn patterns and relationships between words, grammar rules, and context. When generating a sentence, the model considers the provided input, such as a prompt or context, and generates a sentence that adheres to the learned patterns and rules.
What are the applications of language generation sentence?
Language generation sentence has various applications, including chatbots, virtual assistants, content generation, machine translation, and natural language interfaces. It can be used in any scenario where generating coherent and contextually appropriate sentences is required.
Is language generation sentence limited to English only?
No, language generation sentence can be applied to any language. The models used for language generation can be trained on datasets in multiple languages, enabling them to generate sentences in languages other than English.
What are some challenges in language generation sentence?
Some challenges in language generation sentence include generating sentences that are not only grammatically correct but also contextually appropriate and coherent. Ensuring that the generated sentences are understandable and do not contain biased or offensive language is also a challenge.
Can language generation sentence be personalized?
Yes, language generation sentence can be personalized by providing the model with specific input or context related to the user. For example, by incorporating user preferences, demographics, or past interactions, the model can generate sentences that are more tailored to the individual user.
What are some popular language generation sentence models?
Some popular language generation sentence models include GPT-3 (Generative Pre-trained Transformer 3), OpenAI’s ChatGPT, and Google’s T5 (Text-to-Text Transfer Transformer). These models have achieved impressive results in generating high-quality and contextually relevant sentences.
How can language generation sentence benefit businesses?
Language generation sentence can benefit businesses by automating content creation processes, enhancing customer service through chatbots and virtual assistants, improving machine translation capabilities, and enabling personalized communication with customers at scale. It can also support data analysis and generate insights from unstructured text data.
Is language generation sentence suitable for academic research?
Yes, language generation sentence is suitable for academic research, especially in the fields of natural language processing (NLP) and artificial intelligence (AI). Researchers can explore and improve upon existing models, develop novel approaches to language generation, and investigate ethical and societal implications of using these techniques.
Are there any limitations to language generation sentence?
Yes, there are limitations to language generation sentence. The generated sentences may occasionally lack coherence or fail to capture the desired meaning. Models trained on biased or unrepresentative data may produce biased or inappropriate outputs. Ethical considerations, such as ensuring responsible and fair use of these models, also need to be addressed.