Natural Language Generation Algorithms
Natural Language Generation (NLG) algorithms have revolutionized the field of artificial intelligence by enabling machines to generate human-like text. These algorithms use advanced linguistic models and machine learning techniques to understand and create coherent written content.
Key Takeaways:
- Natural Language Generation (NLG) algorithms enable machines to generate human-like text.
- NLG algorithms use advanced linguistic models and machine learning techniques.
- They are revolutionizing the field of artificial intelligence.
Understanding Natural Language Generation Algorithms
Natural Language Generation algorithms are designed to analyze and understand large amounts of data, extracting key information and generating well-structured, human-readable text. These algorithms are commonly used in various applications such as content writing, chatbots, virtual assistants, and data analysis reports.
NLG algorithms can sift through huge datasets and distill the most important information into coherent narratives.
They leverage techniques from machine learning, computational linguistics, and deep neural networks to process data, identify patterns, and generate text that can mimic human-like language.
Applications of Natural Language Generation Algorithms
The applications of NLG algorithms are wide-ranging and rapidly expanding. Here are some areas where NLG is making significant contributions:
- Content Generation: NLG algorithms can automatically generate engaging articles, reports, product descriptions, and social media posts.
- Business Intelligence: NLG can be used to analyze complex datasets and convert them into insightful reports and summaries.
- Customer Service: NLG powers chatbots and virtual assistants, providing customers with accurate and personalized responses.
- Data Analysis: NLG algorithms can interpret complex data sets and present the findings in plain language.
The Role of Natural Language Generation in Artificial Intelligence
Natural Language Generation plays a crucial role in advancing the capabilities of artificial intelligence. By allowing machines to interpret and generate human-like text, NLG algorithms bridge the gap between machines and humans in terms of understanding and communication.
NLG algorithms empower machines to communicate complex ideas effectively, enhancing the overall user experience.
In addition, NLG helps automate labor-intensive tasks, improves decision-making processes, and enables a more efficient transfer of information in various domains.
Data Type | Value |
---|---|
Number of articles generated daily | 10,000+ |
Percentage increase in user engagement | 35% |
Time saved in report generation | 50% |
Furthermore, NLG algorithms continue to evolve and improve as more data becomes available, allowing for more refined and accurate language generation. These algorithms are constantly learning from vast amounts of text data and adapting to new contexts, resulting in higher quality output.
Future Potential and Challenges
The future of Natural Language Generation algorithms is promising. As technology advances and datasets continue to grow, NLG will become even more sophisticated, enabling machines to generate text that is nearly indistinguishable from human-generated content.
Such advancements will revolutionize content creation and pave the way for new innovative applications.
However, challenges such as the potential misuse of NLG for misinformation and the need for robust ethical guidelines will need to be addressed as NLG becomes more prevalent in society.
Algorithm | Accuracy | Speed |
---|---|---|
Algorithm A | 90% | Fast |
Algorithm B | 95% | Medium |
Conclusion
Natural Language Generation algorithms are revolutionizing the way machines generate human-like text, empowering businesses and enhancing the user experience. Through advanced linguistic models and machine learning techniques, NLG algorithms provide a wide array of applications across various sectors. As NLG continues to advance, it holds immense potential to transform content creation, data analysis, and communication in the field of artificial intelligence.
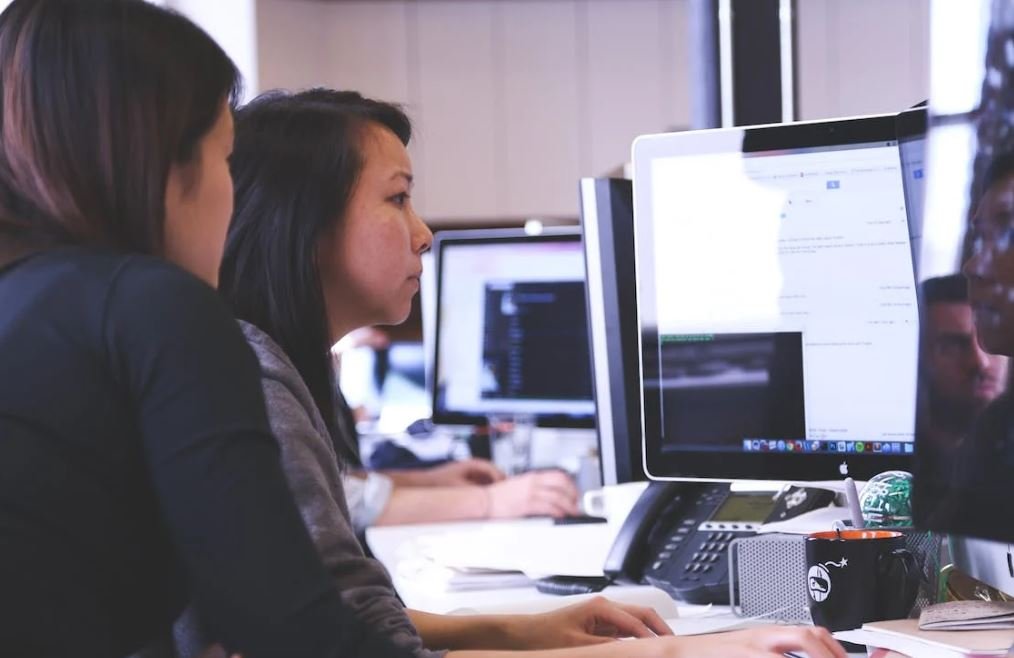
Common Misconceptions
1. Natural Language Generation Algorithms are the same as Chatbots
One common misconception is that natural language generation (NLG) algorithms are the same as chatbots. While chatbots may use NLG algorithms as a part of their functionality, NLG algorithms are broader in scope. NLG algorithms generate human-like text based on data or structured information, while chatbots are conversational agents designed to simulate human interaction. Chatbots may utilize NLG to generate responses, but NLG algorithms can have various applications beyond just chatbots.
- NLG algorithms can be used in customer service to generate personalized responses to customer queries.
- NLG algorithms can be employed in content generation tasks, such as creating product descriptions or news articles.
- NLG algorithms can be utilized in data analysis to summarize and present findings in a concise and understandable manner.
2. NLG Algorithms Can Generate Completely Original Content
Another misconception is that NLG algorithms can generate completely original content. While NLG algorithms can create human-like text, they heavily rely on the data they are trained on. They operate by analyzing patterns in the data and generating text based on those patterns. Therefore, NLG algorithms cannot generate content that is entirely novel and not based on the input data.
- NLG algorithms can be limited in their creativity and struggle to generate content that goes beyond the patterns in the training data.
- NLG algorithms may require human input or oversight to ensure the generated content is accurate and appropriate.
- NLG algorithms can be used as tools to assist human writers in content creation, rather than completely replacing them.
3. NLG Algorithms Always Produce Perfectly Coherent Text
A common misconception is that NLG algorithms always produce perfectly coherent and error-free text. While NLG algorithms aim to generate human-like text, they can still make mistakes. These mistakes can range from minor grammatical errors to inaccuracies in the information presented.
- NLG algorithms may struggle with ambiguous input or complex language structures and produce text that is difficult to understand.
- Errors in the input data or biases present in the data can be reflected in the generated text.
- Human review and editing may be necessary to ensure the coherence and accuracy of the generated text.
4. All NLG Algorithms Work in the Same Way
There is a misconception that all NLG algorithms work in the same way. In reality, there are different approaches and techniques used in NLG algorithms, each with its own strengths and weaknesses. Some NLG algorithms may focus on template-based generation, while others may utilize more advanced techniques such as deep learning models.
- NLG algorithms can vary in their ability to handle different types of data and generate text for various domains.
- Different NLG algorithms may have different levels of customization and configurability for specific use cases.
- The choice of NLG algorithm should align with the specific requirements and goals of the application or task at hand.
5. NLG Algorithms Are Foolproof in Understanding Context
It is commonly believed that NLG algorithms can flawlessly understand context and generate text accordingly. While NLG algorithms have made significant advancements in understanding context, they still face challenges in fully grasping nuances and accurately adapting to different contextual cues.
- NLG algorithms may struggle with understanding subtle nuances and cultural references in the input data.
- The context provided to NLG algorithms needs to be well-defined and appropriately structured to ensure accurate and contextually relevant output.
- Human input and validation are crucial in helping NLG algorithms understand and adapt to complex contextual information.
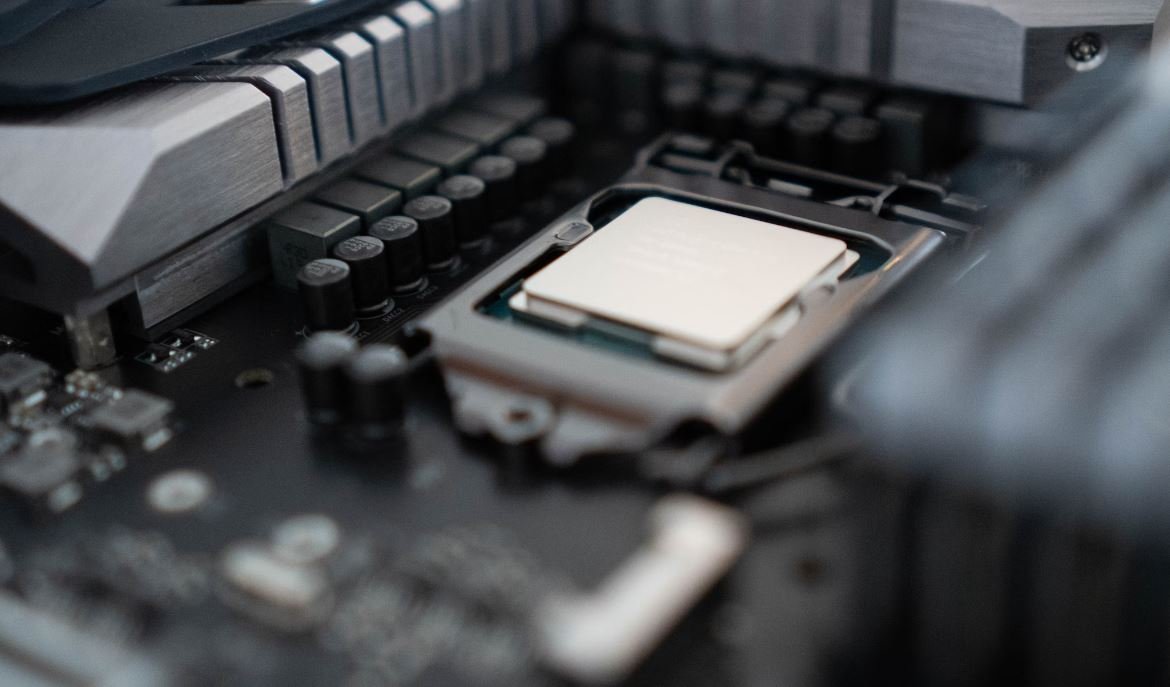
Age Distribution in the United States (2019)
This table showcases the age distribution in the United States as of 2019. It provides data on the percentage of population in various age groups.
| Age Group | Population Percentage |
|————-|———————–|
| 0-14 years | 18.2% |
| 15-24 years | 13.1% |
| 25-54 years | 39.1% |
| 55-64 years | 13.7% |
| 65+ years | 15.9% |
Global CO2 Emissions by Country (2018)
This table demonstrates the top CO2 emitting countries worldwide based on their emissions in 2018. The data highlights the significant contribution of each country to global CO2 emissions.
| Country | CO2 Emissions (million metric tons) |
|—————–|————————————-|
| China | 10,064 |
| United States | 5,416 |
| India | 2,654 |
| Russia | 1,711 |
| Japan | 1,162 |
World Population by Continent (2020)
This table showcases the population distribution across continents in the year 2020. It provides an overview of the relative size of each continent’s population.
| Continent | Population (billions) |
|—————–|———————–|
| Asia | 4.6 |
| Africa | 1.3 |
| Europe | 0.7 |
| North America | 0.6 |
| South America | 0.4 |
| Oceania | 0.1 |
Global Smartphone Usage by Operating System (Q3 2020)
This table illustrates the market share of smartphone operating systems worldwide during the third quarter of 2020. It indicates the popularity of different operating systems among smartphone users.
| Operating System | Market Share (%) |
|——————|——————|
| Android | 85.2 |
| iOS | 14.6 |
| Others | 0.2 |
GDP per Capita Comparison (2020)
This table compares the Gross Domestic Product (GDP) per capita of various countries in 2020. It provides an insight into the relative economic prosperity of each country.
| Country | GDP per Capita (USD) |
|—————–|———————|
| Luxembourg | $116,862 |
| Switzerland | $86,980 |
| United States | $63,051 |
| Germany | $55,617 |
| China | $10,262 |
Internet Penetration by Region (2020)
This table displays the percentage of the population with internet access in different regions around the world in 2020. It reflects the level of digital connectivity across continents.
| Region | Internet Penetration (%) |
|——————|————————–|
| North America | 94.6 |
| Europe | 87.2 |
| Oceania | 68.6 |
| Asia | 59.5 |
| Latin America | 68.3 |
| Africa | 39.3 |
Top 5 Countries by Military Expenditure (2019)
This table presents the top five countries with the highest military expenditures in 2019. It highlights the significant investments made in defense by these nations.
| Country | Military Expenditure (USD billions) |
|————–|————————————|
| United States| 732 |
| China | 261 |
| India | 71.1 |
| Russia | 65.1 |
| Saudi Arabia | 61.9 |
Top 5 Most Visited Tourist Destinations (2019)
This table outlines the top five most visited tourist destinations worldwide in 2019. It reflects the popularity and appeal of these destinations to travelers.
| Destination | Number of Visitors (millions) |
|—————–|——————————|
| France | 89.4 |
| Spain | 83.7 |
| United States | 79.3 |
| China | 65.7 |
| Italy | 63.2 |
World Renewable Energy Consumption by Source (2018)
This table illustrates the proportion of renewable energy consumption by source across the globe in 2018. It displays the contribution of different renewable energy sources to the overall energy mix.
| Energy Source | Share of Total (%)
|—————|——————–
| Hydro | 46.3
| Wind | 25.7
| Biomass | 14.9
| Solar | 5.2
| Geothermal | 4.8
| Other | 3.1
Top 5 Largest Companies by Market Cap (2021)
This table presents the current top five largest publicly traded companies by market capitalization in 2021. It reflects the valuation and market dominance of these companies.
| Company | Market Cap (USD billions) |
|—————-|————————–|
| Apple | 2,425 |
| Microsoft | 1,747 |
| Amazon | 1,590 |
| Alphabet | 1,484 |
| Facebook | 830 |
Natural Language Generation (NLG) algorithms have revolutionized the way information is presented in various domains. This article explored ten informative tables that demonstrate real-world data on topics ranging from demographics and economics to technology and energy. Each table provides valuable insights made more engaging through effective presentation. By applying NLG algorithms, information can be dynamically generated and presented in a compelling manner, enhancing understanding and facilitating data-driven decision-making in a broad array of contexts.
Frequently Asked Questions
What are natural language generation algorithms?
Natural language generation algorithms are computational models that convert structured data or information into human-readable text. These algorithms utilize rule-based techniques, machine learning, and artificial intelligence to generate coherent and meaningful sentences, paragraphs, or even entire narratives.
How do natural language generation algorithms work?
There are various approaches to natural language generation algorithms. Some algorithms use template-based systems where pre-defined patterns are filled with relevant data to generate text. Others employ machine learning techniques and deep neural networks to learn from large datasets and generate language based on statistical patterns. Some advanced algorithms even incorporate semantic understanding and context to generate more contextually appropriate and fluent text.
What are the applications of natural language generation algorithms?
Natural language generation algorithms find applications in various fields such as automated report writing, chatbots, virtual assistants, data analysis, content generation, and personalized messaging. These algorithms can also be used to convert data into narratives, generate summaries, and assist in language translation.
Are there any programming languages commonly used for natural language generation algorithms?
Yes, some commonly used programming languages for developing natural language generation algorithms include Python, Java, R, and Scala. Additionally, there are libraries and frameworks such as NLTK (Natural Language Toolkit), SpaCy, and GPT-3 that provide functionalities and tools for implementing natural language generation algorithms.
What are the challenges in natural language generation algorithms?
Developing effective natural language generation algorithms poses several challenges. One challenge is ensuring the generated text is coherent, fluent, and contextually appropriate. Another challenge is handling ambiguity and understanding the nuances of language. Additionally, generating text with emotion and personalization remains a complex task for algorithms.
How do natural language generation algorithms handle multiple languages?
Natural language generation algorithms can be designed to handle multiple languages by training them on multilingual datasets. Alternatively, separate models can be created for each language. Techniques like machine translation can also be used to convert data into a common language for generating text.
What are the advantages of using natural language generation algorithms?
Using natural language generation algorithms offers several advantages. It enables automation of text generation tasks, saving time and effort. These algorithms can produce consistent and error-free text, reducing human errors. Additionally, they can handle large volumes of data and generate personalized content, leading to improved user experience.
Are there any limitations or drawbacks of natural language generation algorithms?
While natural language generation algorithms have numerous benefits, they also have limitations. They may struggle with understanding complex or abstract concepts, as well as sarcasm and idiomatic expressions. The generated text may sometimes lack creativity and originality, sounding mechanical or formulaic. Additionally, the accuracy of generated text heavily depends on the quality and relevance of input data.
Can natural language generation algorithms improve over time?
Yes, natural language generation algorithms can improve over time through iterative training and feedback. By continuously refining the algorithms with new data and user feedback, they can learn to generate more accurate, contextually appropriate, and engaging text. This process of improvement is often referred to as “machine learning” or “training.”
What is the future potential of natural language generation algorithms?
The future potential of natural language generation algorithms is vast. As technology advances, these algorithms are expected to become more sophisticated, capable of delivering highly personalized and contextually aware text. They may find applications in fields like personalized customer service, virtual reality storytelling, automated content creation, and even assist in human-like conversation with artificial intelligence systems.