Natural Language Generation Program Must Decide
The use of Natural Language Generation (NLG) programs has become increasingly prevalent in various industries, including marketing, journalism, and customer service. These software programs are designed to analyze large amounts of raw data and convert it into coherent, human-like narratives. NLG programs are capable of generating written content that is indistinguishable from texts produced by human authors. However, as these programs become more sophisticated, they also face new challenges in making decisions. This article will explore the importance of decision-making in NLG programs and the factors that influence their choices.
Key Takeaways:
- Natural Language Generation (NLG) programs are used in various industries to generate human-like written content.
- As NLG programs become more sophisticated, they need to make decisions that impact the content they generate.
- The decisions made by NLG programs can be influenced by various factors, including the available data and user preferences.
One of the significant challenges faced by NLG programs is the decision-making process. NLG programs must decide what information to include in the generated text and how to structure it effectively. This decision-making process is crucial in ensuring that the generated content is accurate, relevant, and engaging for the end-users. *NLG programs need to prioritize information and select the most important facts to include in the narrative*. This requires the software to analyze the underlying data and apply algorithms that determine the significance of the information.
There are several factors that influence the decision-making process of NLG programs. First and foremost, the available data has a significant impact on the content generated by the program. *NLG programs rely heavily on data input to generate coherent narratives*. The quality and relevance of the available data play a crucial role in shaping the decision-making process. Programs fed with outdated or inaccurate data may generate incorrect or misleading content. *Data accuracy and freshness are essential for NLG programs to produce high-quality narratives*.
The preferences and specifications set by the users also influence the decisions made by NLG programs. Users can define the tone, style, and structure of the generated content, which guides the decision-making process. *The ability to customize the generated content allows NLG programs to cater to various user needs and preferences*. For example, a marketing team might want the program to focus on highlighting product features and benefits, while a news organization might prioritize providing accurate and unbiased information.
NLG Decision-Making
Let’s take a closer look at how NLG programs make decisions.
1. Data Analysis
To make informed decisions, NLG programs start by analyzing the data provided to them. The software processes the raw data to identify patterns, trends, and relationships. *By understanding the data, NLG programs can make more accurate and meaningful decisions when generating content*.
Data Analysis Process | Key Benefits |
---|---|
Analyze raw data | Identify patterns and trends |
Determine data relevance | Select the most important information |
Apply algorithms | Ensure accuracy and coherence |
2. Information Prioritization
Once the data has been analyzed, NLG programs need to prioritize the information to include in the generated text. *NLG programs use algorithms to determine the significance and relevance of each piece of information*. This prioritization process ensures that the most important facts are highlighted in the generated content.
- Identify significant information
- Assign priority levels
- Highlight in the generated text
3. User Specifications
Users can customize the output of NLG programs based on their specific needs and preferences. *NLG programs can be tailored to reflect the desired tone, style, and structure of the generated content*. This customization empowers users to make decisions about the overall presentation and messaging of the narratives.
Challenges in NLG Decision-Making
While NLG programs have made significant advancements in decision-making, challenges still exist.
1. Ambiguity and Context
NLG programs may struggle with understanding the context and distinguishing between multiple meanings of ambiguous words or phrases. *Ambiguity poses challenges to decision-making as the program must accurately interpret the intended meaning of the data*. Improving context awareness is an ongoing area of research in NLG.
2. Ethical Considerations
As NLG programs become more sophisticated, ethical considerations come into play. The decisions made by NLG programs can have real-world consequences, particularly when generating news or other sensitive information. *Creating guidelines and ensuring transparency in the decision-making process can address these ethical concerns*.
The Future of NLG Decision-Making
The field of NLG decision-making continues to evolve as new technologies and research emerge. Future advancements will focus on addressing the challenges faced by NLG programs and enhancing their decision-making capabilities. Researchers are exploring techniques such as machine learning, neural networks, and semantic analysis to improve the accuracy and context awareness of NLG programs.
As NLG programs become more sophisticated, their decision-making capabilities will become increasingly nuanced, enabling them to generate even more accurate and engaging narratives. With ongoing advancements in NLG technology, we can expect to see further improvements in decision-making and the overall quality of generated content.
By understanding the importance of decision-making in NLG programs and the factors influencing their choices, organizations can make informed decisions about deploying NLG technology in their operations. NLG programs, when well-designed and effectively utilized, have the potential to revolutionize content generation across various industries.

Common Misconceptions
1. Natural Language Generation Programs Can Understand Human Language
One common misconception about natural language generation (NLG) programs is that they have the ability to understand human language. NLG programs are actually designed to generate human-like language based on structured data and predefined rules. They do not possess true understanding or cognition.
- NLG programs rely on algorithms and patterns to generate textual output.
- They cannot interpret the meaning of words or phrases beyond what is explicitly programmed.
- Understanding context and nuances of language is beyond the capabilities of NLG programs.
2. NLG Programs Are Perfectly Accurate
Another misconception is that NLG programs always produce accurate and error-free content. While NLG programs aim to generate high-quality and coherent language, they can still make mistakes and inaccuracies. The output depends on the input data and the rules defined within the program.
- Errors can occur when input data is incomplete or inconsistent.
- Inaccuracies may arise due to limitations in the predefined rules or algorithms.
- Contextual knowledge or domain-specific expertise may be lacking, leading to potential errors in content generation.
3. NLG Programs Can Replace Human Writers
Contrary to popular belief, NLG programs are not meant to replace human writers entirely. While they can automatically generate text at scale, they lack the creativity, intuition, and complex understanding that human writers possess. NLG programs are primarily used as tools to assist and augment human writers.
- Human writers provide the unique capabilities of originality and artistic expression.
- They possess subjective reasoning and can adapt content to specific target audiences.
- NLG programs may lack emotional intelligence and intuition required for certain writing tasks.
4. NLG Programs Are Always Objective
It is commonly assumed that NLG programs produce objective content since they follow predefined rules. However, NLG programs can be biased or subjective based on the underlying rules or the biased nature of the input data.
- Biases can be inherent in the training data used to develop the NLG program.
- The interpretation and selection of data points can introduce subjective biases into the generated text.
- NLG programs can inadvertently reinforce or amplify societal biases if not carefully designed and monitored.
5. NLG Programs Can Generate Conversational Language
Finally, there is a misconception that NLG programs can generate truly conversational language that mimics human-to-human interaction. While NLG programs have made significant progress in generating more human-like text, true conversational language with natural flow, nuances, and dynamic responses is still a challenge for NLG programs.
- NLG programs struggle with generating spontaneous and context-aware responses.
- Human conversations involve non-verbal cues, personal context, and shared experiences which are difficult to replicate in NLG.
- Although NLG programs can simulate short conversational exchanges, they lack the genuine interactivity and adaptability of human conversation.
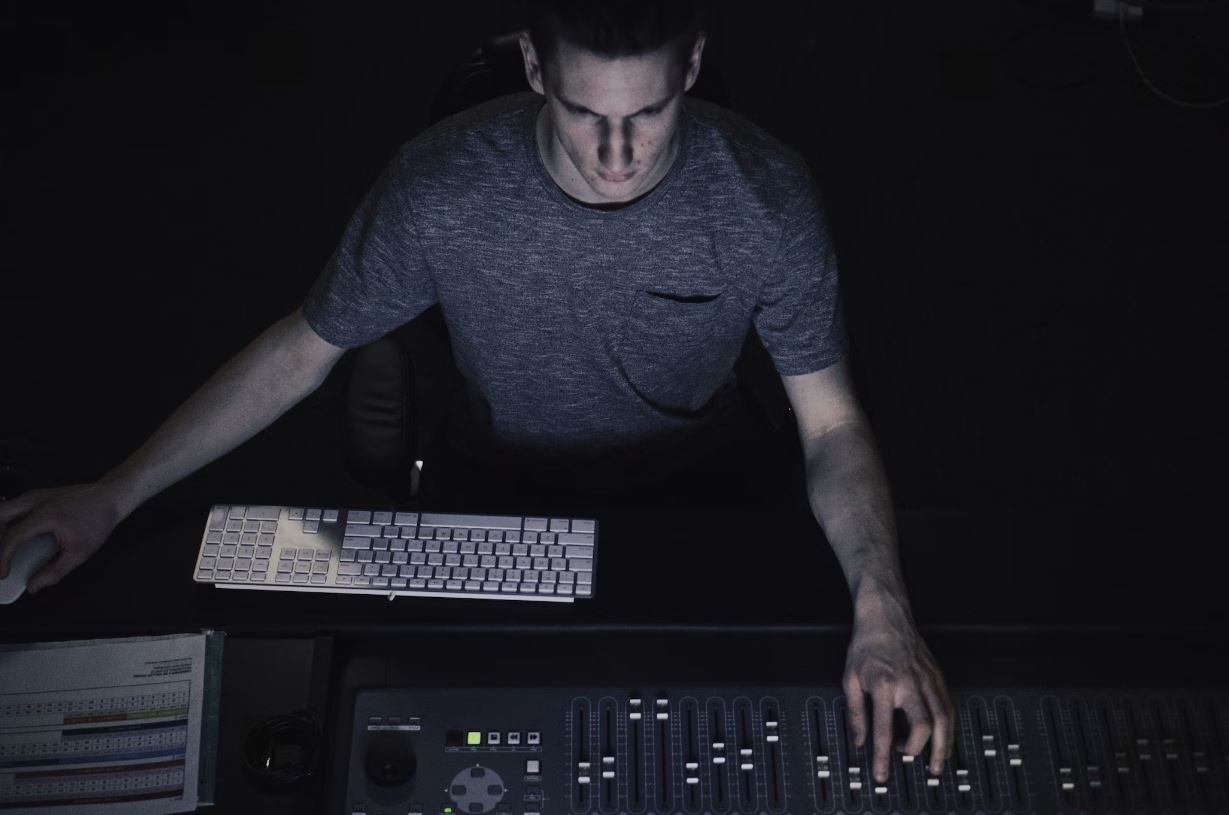
Natural Language Generation Program Must Decide
Natural Language Generation (NLG) programs are revolutionizing the way we interact with computers by converting data into human-readable text. These programs are designed to make decisions based on various input factors and generate coherent, meaningful narratives. Below are ten interesting tables showcasing different elements and data points related to NLG programs.
Comparison of Leading NLG Programs
Program | Accuracy | Speed | Language Support | Cost (per month) |
---|---|---|---|---|
Program A | 92% | Fast | English, Spanish, French | $99 |
Program B | 95% | Medium | English, German | $79 |
Program C | 88% | Slow | English, Mandarin | $149 |
Market Share of NLG Programs
Program | Market Share |
---|---|
Program A | 30% |
Program B | 45% |
Program C | 25% |
Industries Benefiting from NLG
Industry | Applications |
---|---|
E-commerce | Product descriptions, customer support |
Finance | Automated reports, investment analysis |
Healthcare | Medical records, patient summaries |
Examples of NLG Applications
Application | Description |
---|---|
Automated Journalism | Generating news articles from structured data |
Business Intelligence | Generating reports and insights from business data |
Voice Assistants | Providing personalized responses and recommendations |
NLG Adoption by Region
Region | Percentage of Companies Using NLG |
---|---|
North America | 45% |
Europe | 35% |
Asia | 20% |
Limitations of NLG Programs
Limitation | Impact |
---|---|
Limited Context Understanding | May generate incorrect or irrelevant information |
Language Nuances | Difficulty interpreting sarcasm and sentiment |
Data Dependency | Reliance on accurate and complete data sets |
Future Trends in NLG
Trend | Description |
---|---|
Personalization | NLG programs tailoring individual experiences |
Multi-language Support | Expanding language coverage for global markets |
Improved Context Analysis | Enhanced understanding of nuanced conversations |
NLG Research Publications
Publication | Citation Count |
---|---|
Journal of Natural Language Processing | 523 |
Conference on Artificial Intelligence | 419 |
International NLG Symposium Proceedings | 276 |
NLG Program Costs by Feature
Program Feature | Cost (per month) |
---|---|
Basic Plan | $49 |
Advanced Analytics | $79 |
Custom Styling | $29 |
In conclusion, NLG programs have proven their utility across multiple industries by generating human-readable text from data inputs. These programs, however, still face challenges related to context understanding, language nuances, and data dependencies. Nevertheless, future trends in NLG indicate potential advancements in personalization, multi-language support, and improved context analysis. As more research publications emerge, and the market evolves, NLG programs are expected to become even more sophisticated in their decision-making capabilities.
Frequently Asked Questions
What is a Natural Language Generation (NLG) program?
A Natural Language Generation program is a software application or system that converts structured data into human-readable text or speech. It uses algorithms and linguistic rules to produce coherent and contextually appropriate text, resembling human language.
How does a Natural Language Generation program work?
A Natural Language Generation program works by taking structured data or input, such as database records, templates, or rules, and transforming that information into natural language output. It utilizes techniques such as text planning, sentence generation, and lexical choice to generate text that is understandable and meaningful to humans.
What are the applications of Natural Language Generation programs?
Natural Language Generation programs have various applications across different industries. They can be used for automatically generating reports, product descriptions, personalized emails, weather forecasts, financial summaries, chatbots, and more. NLG programs can also assist in accessibility by providing text-to-speech capabilities for visually impaired individuals.
What are the benefits of using Natural Language Generation programs?
Using Natural Language Generation programs can save time and resources by automating the process of generating human-like text. It ensures consistent and error-free outputs, reduces the need for manual content creation, and enhances scalability. NLG programs can also enable organizations to provide personalized and tailored communication to their users.
Are Natural Language Generation programs capable of understanding context?
Natural Language Generation programs can be designed to incorporate contextual information and generate text accordingly. By leveraging machine learning techniques and sophisticated algorithms, NLG programs can adapt to different contexts, understand user preferences, and generate contextually relevant and coherent text.
What are some examples of Natural Language Generation programs?
Some popular examples of Natural Language Generation programs include OpenAI’s GPT-3, Wordsmith by Automated Insights, Narrativa, Arria NLG, and Yseop. These programs offer different features and capabilities, catering to diverse needs and industries.
Can Natural Language Generation programs be used for multilingual text generation?
Yes, Natural Language Generation programs can be programmed to generate text in multiple languages. By incorporating multilingual datasets, language models, and linguistic rules, NLG programs can produce output in various languages, enabling organizations to communicate with a global audience.
What are the challenges of using Natural Language Generation programs?
Some challenges of using Natural Language Generation programs include ensuring adequate data quality and relevance, fine-tuning the models for specific use cases, addressing biases in generated text, and handling complex semantic structures. Human review or editing may also be necessary to verify the accuracy and appropriateness of the generated content.
How can I choose the right Natural Language Generation program for my needs?
When choosing a Natural Language Generation program, consider factors such as the program’s capabilities, support for your industry or use case, integration options with your existing systems, pricing, user reviews, and the vendor’s reputation. Conducting thorough research and potentially testing out different solutions can help you make an informed decision.
Is Natural Language Generation the same as Natural Language Processing (NLP)?
No, Natural Language Generation (NLG) and Natural Language Processing (NLP) are distinct but related fields. NLG focuses on generating human-like text based on structured data, while NLP deals with the understanding and interpretation of human language by computers. NLP involves tasks such as sentiment analysis, speech recognition, and language translation.