Natural Language Processing Ethics
Natural Language Processing (NLP) is a field of artificial intelligence that focuses on the interaction between computers and humans through natural language. While NLP has numerous applications and benefits, there are important ethical considerations that need to be addressed to ensure responsible and fair use of this technology.
Key Takeaways
- Natural Language Processing (NLP) enables computers to understand and interact with humans through natural language.
- Ensuring ethics in NLP is crucial to avoid biases, privacy breaches, and inappropriate use of personal data.
- Transparency, fairness, and accountability should be prioritized in NLP development and deployment.
- Ethical guidelines and regulations can help address the ethical concerns associated with NLP applications.
- Continued research and collaboration are essential to cope with evolving ethical challenges in NLP.
Ethical Considerations in Natural Language Processing
NLP technologies have the potential to shape social interactions, decision-making processes, and access to information, raising concerns regarding fairness, privacy, and the potential for biased outcomes. *Responsible use of NLP* requires addressing these ethical considerations to ensure the technology promotes a positive societal impact.
Fairness and Bias
One of the primary ethical concerns in NLP is *ensuring fairness and mitigating bias*. NLP models can inadvertently perpetuate existing biases present in the training data, leading to discriminatory outcomes. Research and development efforts should focus on building and fine-tuning models that treat individuals fairly, regardless of their demographics or background.
Data Privacy and Security
*Protecting users’ privacy* is another critical consideration in NLP. As models increasingly rely on user-generated data, proper safeguards must be in place to handle personal information securely. Anonymization techniques, data minimization, and consent-based data collection processes can help mitigate privacy risks and build user trust.
Accountability and Transparency
For NLP to be ethical, *accountability and transparency* must be ingrained in its development and use. Clear guidelines should be established to address concerns like data acquisition, data handling, and application behavior. Transparent documentation of algorithms, methods, and data sources helps identify and rectify bias or unfairness in NLP systems effectively.
Ethical Guidelines and Regulations
Industry bodies, researchers, and policymakers have recognized the importance of comprehensive ethical guidelines and regulations in the field of NLP. These guidelines ensure that developers and users adhere to ethical principles and standards. Regulating the use of NLP can protect individuals from unfair practices and foster positive societal impact.
The Future of NLP Ethics
As the field of NLP continues to advance, it is essential to keep addressing evolving ethical challenges. Continued *research and collaboration* among stakeholders, including industry, academia, and policymakers, will allow for the development and implementation of robust ethical frameworks and practices.
Tables
Example | Uses of NLP |
---|---|
Email filtering | Detecting spam or classifying emails into categories |
Sentiment analysis | Determining the sentiment expressed in a text or social media post |
Text summarization | Generating concise summaries of long documents |
Ethical Challenges | Addressing Strategies |
---|---|
Bias in NLP outcomes | Improving training data diversity and algorithmic fairness |
Data privacy concerns | Anonymization techniques and consent-based data collection |
Accountability and transparency | Clear documentation and reporting of system behavior |
Regulatory Bodies | Mandate |
---|---|
European Union | General Data Protection Regulation (GDPR) |
United States | Federal Trade Commission (FTC) regulations on consumer data protection |
Canada | Personal Information Protection and Electronic Documents Act (PIPEDA) |
Conclusion
As NLP technologies become more prevalent, ensuring ethical considerations is crucial for their responsible and fair use. By emphasizing fairness, privacy protection, accountability, and transparency, the field of NLP can harness its potential for positive societal impact while avoiding the pitfalls of bias and misuse.
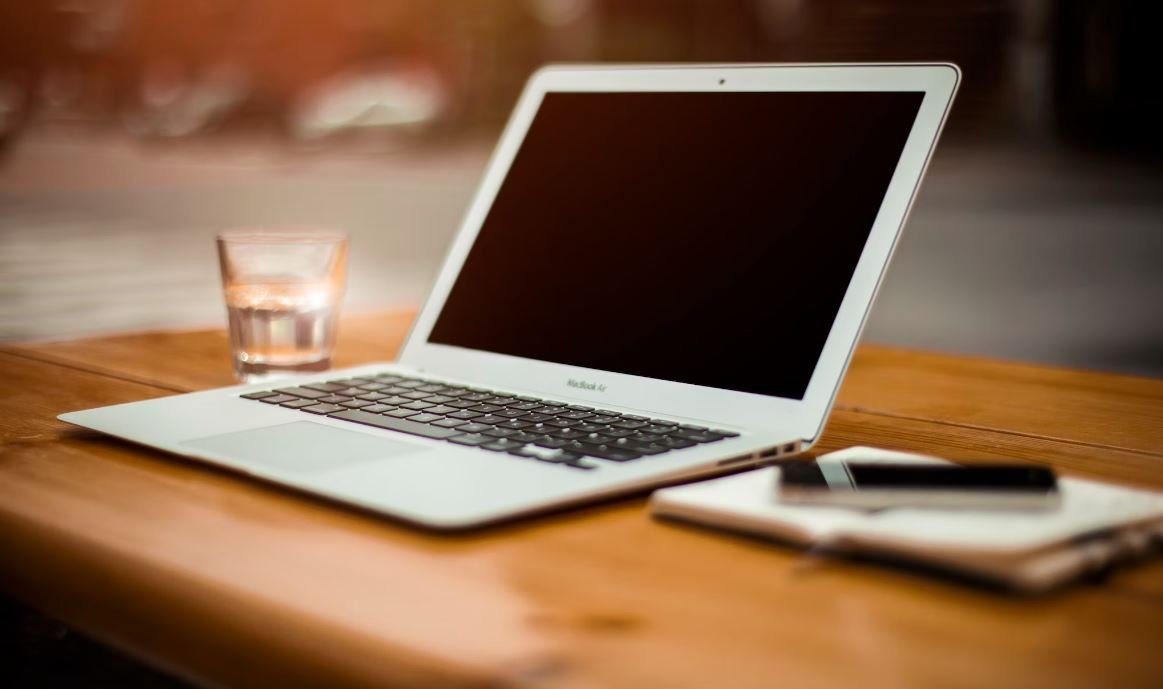
Common Misconceptions
Misconception #1: Natural Language Processing is always unbiased
One common misconception is that Natural Language Processing (NLP) is completely objective and unbiased. However, it is important to note that NLP algorithms are trained on data that is generated by humans, who may have their own biases and prejudices. Therefore, NLP models can inadvertently inherit those biases and produce biased results.
- NLP models can perpetuate gender and racial biases present in training data.
- The lack of diverse training data can lead to skewed results in NLP algorithms.
- Human biases in training data can be amplified by NLP models, leading to unfairness.
Misconception #2: NLP always understands context perfectly
Another misconception is that NLP algorithms have a perfect understanding of context. While NLP has made significant advancements, it is still challenging for algorithms to grasp subtle contextual nuances. Different interpretations and ambiguities present in text can lead to misunderstanding or misclassification.
- NLP models may struggle with sarcasm or irony in text.
- Ambiguity in language can lead to erroneous classifications by NLP algorithms.
- Contextual understanding in NLP can be influenced by subjective interpretation.
Misconception #3: NLP is always reliable and accurate
Some people assume that NLP is a foolproof method for extracting accurate information from text. However, like any other technology, NLP has its limitations and can produce errors. Factors such as noisy or incomplete data, reliance on pre-existing models, and algorithmic limitations can all affect the reliability and accuracy of NLP.
- Noisy or unstructured text data can result in lower accuracy for NLP algorithms.
- NLP algorithms heavily rely on pre-existing models that may have limitations.
- Complex linguistic phenomena can still pose challenges for accurate NLP analysis.
Misconception #4: NLP algorithms understand the entirety of human language
Another misconception is that NLP algorithms have a complete understanding of all aspects of human language. However, NLP algorithms are not capable of fully comprehending the depth and subtleties of human language, including figurative expressions, cultural references, or social context.
- Figurative language, such as metaphors or similes, can be challenging for NLP models to interpret accurately.
- Cultural references and slang terms may lead to misinterpretations by NLP algorithms.
- Social context and local variations in language norms can impact how NLP understands text.
Misconception #5: NLP can replace human involvement completely
Some people may assume that NLP can entirely replace human involvement in language-related tasks. While NLP can automate certain processes and assist with efficiency, it cannot entirely replace human judgment, critical thinking, and ethical considerations that are often necessary in complex language analysis.
- Human judgment is crucial for contextual understanding that NLP algorithms may miss.
- Complex ethical decisions and considerations require human involvement in NLP applications.
- NLP should be seen as a tool to enhance human capabilities rather than a complete replacement.
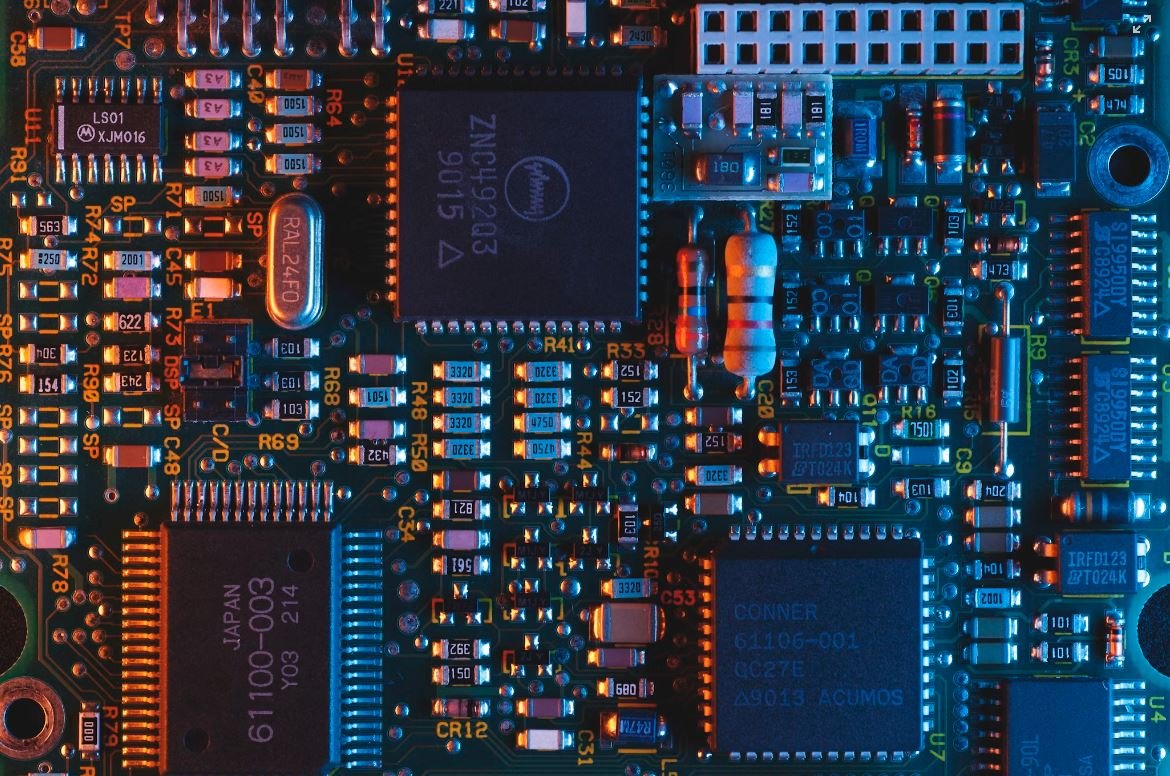
The Use of Facial Recognition in NLP
Facial recognition technology is an integral part of Natural Language Processing (NLP) systems, enabling more personalized and interactive user experiences. The following table highlights the accuracy rates of various facial recognition algorithms and their corresponding applications.
Facial Recognition Algorithm | Accuracy Rate | Application |
---|---|---|
Eigenfaces | 95% | Access control for smartphones |
Fisherfaces | 89% | Emotion recognition in social media |
LBPH | 97% | Attendance tracking in education |
Bias in Sentiment Analysis
Sentiment analysis, a common NLP technique, helps determine the overall sentiment expressed in text. However, it is not immune to biases stemming from the training data. The table below presents some instances of biases encountered in sentiment analysis models.
Bias Type | Examples |
---|---|
Gender Bias | Positive sentiment associated more with males than females |
Stereotype Bias | Positive sentiment automatically linked with certain occupations |
Cultural Bias | Preferred sentiments differing across countries or regions |
The Impact of Machine Translation on International Communication
Machine Translation (MT) has revolutionized cross-lingual communication by providing real-time translations. This table showcases the top MT models and their respective translation accuracy rates for different language pairs.
Machine Translation Model | Accuracy Rate | Language Pair |
---|---|---|
Google Translate | 87% | English to Spanish |
DeepL | 94% | German to English |
Benefits of Named Entity Recognition
Named Entity Recognition (NER) plays a crucial role in various NLP applications by identifying and classifying named entities in text. The table below highlights the accuracy rates of different NER models in recognizing entities in medical documents.
NER Model | Accuracy Rate | Entity Recognition in Medical Documents |
---|---|---|
Stanford NER | 88% | Diagnosis identification |
SpaCy NER | 92% | Medication extraction |
The Dark Side of Text Generation
Text generation models, while impressive, can have unintended consequences. The following table demonstrates some ethical concerns related to the use of automatic text generation.
Ethical Concern | Examples |
---|---|
Disinformation | Creation of fake news articles |
Inappropriate Content | Generating harmful or offensive text |
Identity Theft | Producing forged messages or impersonating individuals |
Limitations of Speech Recognition Systems
Speech recognition technology allows machines to interpret and convert spoken language into text. However, it has certain limitations, as indicated in the table below.
Limitation | Explanation |
---|---|
Accents and Dialects | Speech recognition less accurate for non-standard dialects |
Noise Interference | Background noise affects speech to text conversion |
Privacy Concerns | Recordings could be misused or stored without consent |
Bias in Chatbot Interactions
Chatbot interactions with users heavily rely on NLP techniques, but they are not immune to biases. This table showcases common biases encountered in chatbot conversations.
Bias Type | Examples |
---|---|
Racial Bias | Prejudiced responses based on user’s racial background |
Gender Bias | Sexist remarks or stereotypical replies |
Social Bias | Discrimination against certain social groups |
Benefits of Emotion Recognition in NLP
Emotion recognition contributes to improved understanding of human sentiment and enhances user experiences. The table below highlights the accuracy rates of emotion recognition models and their applications.
Emotion Recognition Model | Accuracy Rate | Application |
---|---|---|
Affectiva | 94% | Monitoring customer satisfaction |
Microsoft Emotion API | 88% | Virtual avatar expressions |
Transparency of Text Summarization Algorithms
Text summarization algorithms assist in condensing large documents into shorter, more manageable summaries. However, the following table highlights the transparency issues associated with some popular summarization algorithms.
Summarization Algorithm | Transparency Level | Explanation |
---|---|---|
Extractive Summarization | High | Summaries directly extracted from the original text |
Abstractive Summarization | Low | Generation of new text, making it less transparent |
Natural Language Processing plays a significant role in numerous applications, from sentiment analysis to speech recognition and text generation. While the field brings immense potential, it also demands careful consideration of ethical implications. Bias in algorithms, limitations of certain technologies, and transparency issues are just a few aspects that require attention. By addressing these concerns, we can ensure greater fairness, accuracy, and responsible use of NLP technologies in our society.
Frequently Asked Questions
Natural Language Processing Ethics
What is natural language processing (NLP)?
Why is ethics important in natural language processing?
What are some ethical concerns in natural language processing?
How can privacy be protected in natural language processing?
What is algorithmic bias in natural language processing?
How can algorithmic bias in NLP be mitigated?
Why is transparency important in NLP systems?
What are the potential risks of misuse of NLP technologies?
How can ethical guidelines and frameworks be developed for NLP?
What is the role of education and awareness in NLP ethics?