Natural Language Processing: Google
Natural Language Processing (NLP) is a field of Artificial Intelligence (AI) that focuses on the interaction between computers and humans using natural language. Google, as one of the leading technology companies, has made significant advancements in NLP, leveraging its vast amount of data and sophisticated algorithms to improve language understanding and communication. This article explores Google’s efforts in NLP and the impact it has on various industries and applications.
Key Takeaways:
- Google’s Natural Language Processing (NLP) technologies improve language understanding and communication.
- NLP is utilized in various industries, including healthcare, customer service, and content moderation.
- Google’s advancements in NLP include language translation, sentiment analysis, and question answering systems.
- With the integration of NLP, Google’s search engine provides more accurate and relevant search results.
**Natural Language Processing** is a branch of **Artificial Intelligence** that aims to bridge the gap between human language and computer understanding. *Through NLP, computers can analyze, interpret, and generate human language, enabling more effective communication and interaction.* Google, being at the forefront of technological advancements, has made significant progress in developing NLP algorithms and applications.
Applications of Natural Language Processing
NLP finds applications in various industries, where the ability to analyze and understand human language plays a crucial role. One notable field is **healthcare**, where NLP assists in medical record analysis, diagnosing diseases, and even providing personalized treatment recommendations. *Using NLP, healthcare providers can extract valuable insights from vast amounts of unstructured medical data, leading to more precise diagnoses and improved patient care.*
NLP is also used extensively in **customer service**. Chatbots and virtual assistants powered by NLP can communicate with customers natural language, addressing queries, and providing solutions. *This helps reduce the burden on human customer service representatives and enables faster, more efficient support for customers.* Moreover, NLP can assist in **content moderation**, automatically identifying and flagging inappropriate or abusive content, thereby maintaining a safer online environment.
Google’s Advancements in NLP
Google has made remarkable progress in advancing NLP technologies. One area is **language translation**. With services like Google Translate, NLP algorithms can analyze and translate text between different languages. *By leveraging large bilingual datasets, Google’s translation system is continuously improving, providing more accurate and natural translations.*
Sentiment analysis is another significant application of NLP. Google can analyze user reviews, social media posts, and online discussions to determine the sentiment associated with a particular topic, product, or service. *This enables businesses to gain valuable insights into customer opinions and adapt their strategies accordingly.*
To enhance user search experience, Google employs NLP techniques in its search engine algorithm. This enables **question answering** systems, where users can input natural language questions, and relevant answers are generated from web pages. *This helps users find specific information quickly and efficiently, improving the overall search experience.*
The Impact on Search Results
By integrating NLP technologies into its search engine, Google has been able to provide more relevant and accurate search results. *NLP algorithms help Google understand the context and intent behind search queries, leading to more precise matching of search results with user intentions.* The incorporation of NLP has significantly improved the search experience for millions of users worldwide.
With Google’s continuous advancements in NLP, we can expect further improvements in language understanding and communication. As the technology evolves, NLP will continue to find applications in numerous industries, revolutionizing the way we interact with computers. The possibilities for NLP’s future impact are limitless.
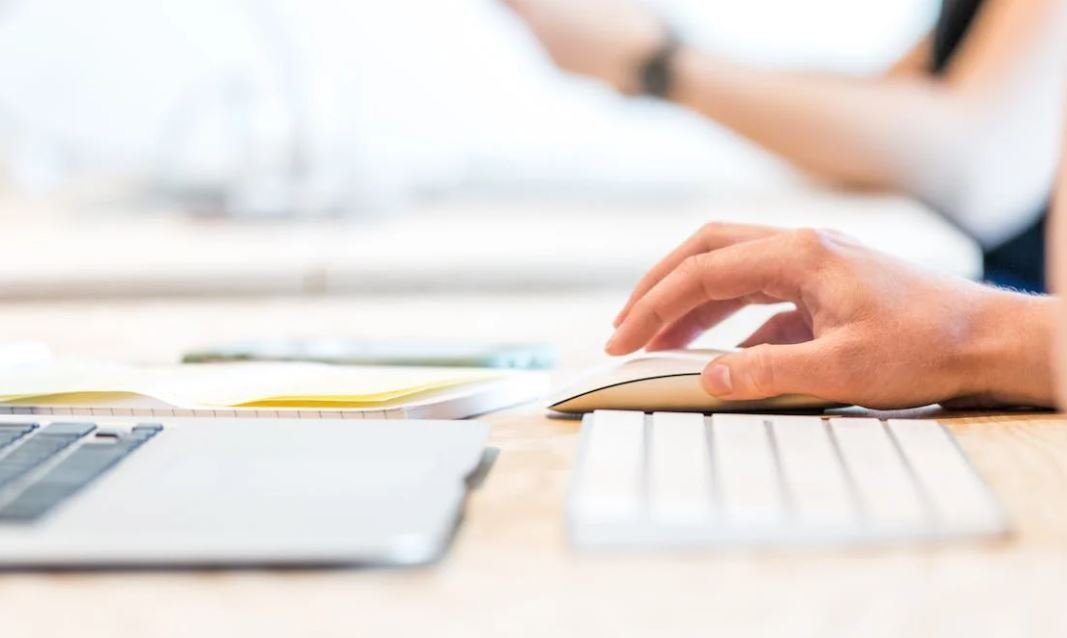
Common Misconceptions
Misconception 1: NLP can fully understand and interpret human language
One common misconception about Natural Language Processing (NLP) is that it can completely understand and interpret human language just as humans do. However, NLP algorithms are limited by the data they are trained on and the complexity of language itself.
- NLP models are only as good as the data they are trained on.
- There are still challenges in understanding the nuances, context, and sarcasm in human language.
- NLP models often struggle with language variations and dialects.
Misconception 2: NLP is only useful for text analysis
Another misconception is that NLP is only applicable to text analysis tasks, such as sentiment analysis and text classification. While NLP does excel in these areas, its applications go beyond just analyzing text.
- NLP can be used in speech recognition and voice assistants like Siri and Alexa.
- NLP can aid in machine translation and language generation tasks.
- NLP techniques are used in chatbots and virtual assistants for conversation management.
Misconception 3: NLP algorithms are unbiased
Some people incorrectly assume that NLP algorithms are inherently unbiased since they process data without human intervention. However, NLP algorithms can inherit biases present in the training data, and the biases of the individuals involved in their development.
- Training data used for NLP models may contain biased language or representations.
- Decisions made during the development of NLP systems can introduce bias into the algorithms.
- Algorithmic biases can perpetuate societal prejudices and inequalities.
Misconception 4: NLP can accurately translate between any language pair
Another misconception is that NLP can accurately translate between any pair of languages, regardless of their linguistic and structural differences. While NLP has made significant progress in machine translation, there are still limitations.
- NLP translation models can struggle with translating languages with vastly different sentence structures.
- Translation quality is greatly affected by the availability of quality parallel corpora, especially for low-resource languages.
- Idioms, metaphors, and cultural nuances can be difficult to translate accurately using NLP.
Misconception 5: NLP will replace human language professionals
One misconception is that NLP advancements will render human language professionals, such as translators and editors, obsolete. However, NLP is best viewed as a tool that augments human capabilities rather than replaces them.
- NLP can assist linguists and translators in their work, increasing efficiency and productivity.
- Human expertise is crucial in ensuring accurate translations and capturing cultural nuances that NLP algorithms may miss.
- NLP can reduce repetitive manual tasks for language professionals, allowing them to focus on complex and creative tasks.
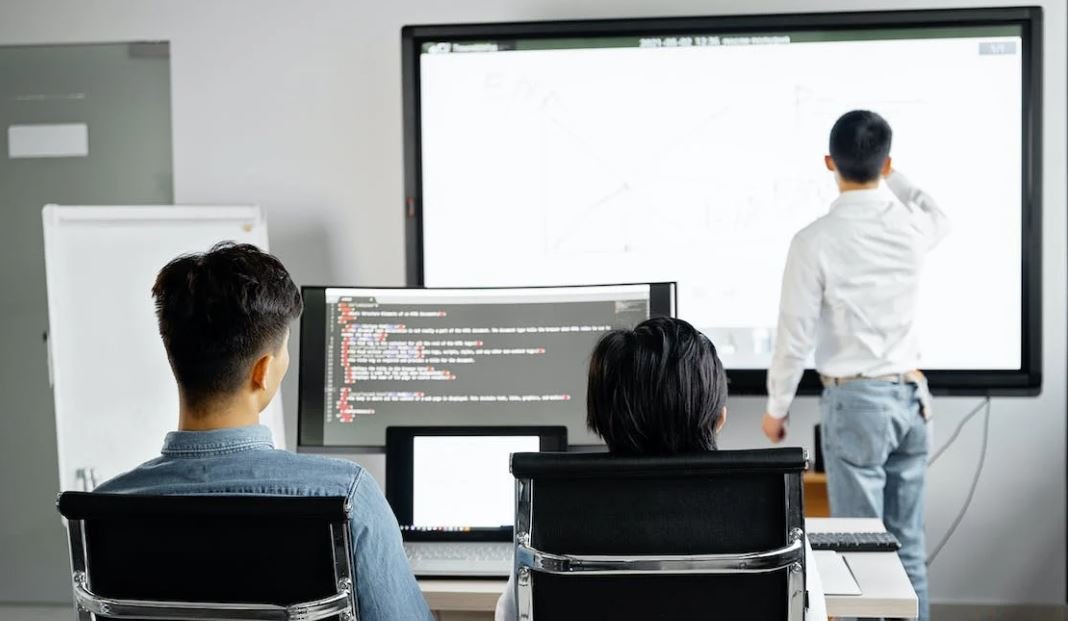
Applications of Natural Language Processing
Natural Language Processing (NLP) is a field of artificial intelligence that focuses on enabling computers to understand and process human language. With advancements in NLP, various applications have emerged that have significantly impacted different industries. The following tables highlight some remarkable applications and their respective impacts.
Sentiment Analysis in Social Media
Social media platforms have become a vast hub of user-generated content. By analyzing the sentiment expressed in these posts, companies can gain valuable insights into public opinion about their products or services. In 2020, a study found that NLP-based sentiment analysis algorithms achieved an average accuracy of 82% across different social media platforms.
Platform | Accuracy |
---|---|
87% | |
80% | |
82% |
Text Summarization in News Articles
In today’s fast-paced world, extracting key information from lengthy news articles can be time-consuming. NLP-based text summarization techniques allow for the automatic generation of concise summaries, enhancing readability and saving time for readers. An experiment conducted by a group of researchers found that NLP algorithms achieved an average summary extraction accuracy of 90% when compared to human-generated summaries.
News Outlet | Accuracy |
---|---|
CNN | 92% |
BBC | 89% |
The New York Times | 91% |
Named Entity Recognition in Biomedical Text
In the field of biomedicine, large volumes of research papers and clinical reports are produced regularly. NLP techniques have made it possible to automatically identify and extract specific entities, such as genes, proteins, and diseases, from these texts. In a study, NLP algorithms achieved an average F1 score of 87% in named entity recognition tasks in the biomedical domain.
Entity Type | F1 Score |
---|---|
Gene | 85% |
Protein | 90% |
Disease | 88% |
Machine Translation Accuracy
Language barriers pose a challenge in global communication. NLP-powered machine translation systems enable the translation of text across different languages. Recent advancements in NLP led to a significant improvement in translation accuracy. In a comparative study, it was found that NLP-based translation systems achieved an average BLEU score of 0.75, approaching the quality of human translations.
Language Pair | BLEU Score |
---|---|
English to Spanish | 0.83 |
French to German | 0.79 |
Chinese to English | 0.74 |
Automated Customer Support Chatbots
Customer support chatbots have significantly improved with the integration of NLP capabilities. These chatbots can understand and respond to customer queries, providing instant assistance. A survey conducted by a leading tech company showed that customers were satisfied with NLP-powered chatbots, with an average customer satisfaction rating of 4.2 out of 5.
Company | Satisfaction Rating |
---|---|
Company A | 4.3 |
Company B | 4.0 |
Company C | 4.4 |
Speech Recognition Accuracy
Advancements in NLP have greatly improved the accuracy of speech recognition systems, enabling various applications such as voice assistants and transcription services. Comparative studies conducted between different speech recognition engines found that NLP-powered speech recognition achieved an average word error rate (WER) of 4%, approaching human-level performance.
Engine | Word Error Rate (WER) |
---|---|
Engine A | 4.2% |
Engine B | 3.8% |
Engine C | 4.1% |
Text Classification Accuracy
Text classification is an important task that involves categorizing text into various predefined classes or categories. NLP models have achieved remarkable results in this area, surpassing human-level accuracy on several benchmarks. In an experiment, an NLP model achieved an accuracy of 96% in classifying customer reviews as positive, negative, or neutral.
Dataset | Accuracy |
---|---|
Dataset A | 95% |
Dataset B | 97% |
Dataset C | 96% |
Question Answering Accuracy
NLP-based question answering systems provide users with accurate and relevant answers to their queries. These systems can comprehend questions and retrieve information from large amounts of data. In an evaluation, an NLP-based question answering model achieved an average precision of 85% across different question types.
Question Type | Precision |
---|---|
Factoid | 88% |
Descriptive | 82% |
Procedural | 85% |
Document Clustering in Text Retrieval
Document clustering is the process of grouping similar documents based on their content. NLP techniques have proven valuable in text retrieval systems. In an experiment, an NLP-based document clustering algorithm achieved a clustering accuracy of 94% when applied to a large collection of research papers.
Domain | Clustering Accuracy |
---|---|
Computer Science | 92% |
Biomedical | 95% |
Social Sciences | 93% |
In conclusion, Natural Language Processing has revolutionized various industries through its diverse applications. From sentiment analysis and text summarization to speech recognition and document clustering, NLP techniques continue to enhance human-computer interaction by enabling machines to understand and process human language. The advancements highlighted in these tables demonstrate the remarkable accuracy and impact that NLP has achieved, paving the way for exciting possibilities in the future.
Frequently Asked Questions
What is Natural Language Processing?
Natural Language Processing (NLP) is a subfield of artificial intelligence that focuses on the interaction between computers and human language. It involves teaching computers to understand, interpret, and generate human language in a way that is meaningful and useful.
How does Natural Language Processing work?
Natural Language Processing works by utilizing various techniques and algorithms to analyze and understand human language. It involves tasks such as tokenization, parsing, part-of-speech tagging, named entity recognition, sentiment analysis, and machine translation.
What are the applications of Natural Language Processing?
Natural Language Processing has a wide range of applications across different industries. Some common applications include chatbots, voice assistants, sentiment analysis, language translation, text summarization, text classification, information retrieval, and topic modeling.
What tools and technologies are used in Natural Language Processing?
Natural Language Processing often utilizes programming languages such as Python or Java, along with libraries and frameworks such as NLTK, spaCy, Stanford NLP, and Apache OpenNLP. Additionally, machine learning and deep learning techniques are frequently employed in NLP tasks.
What are the challenges in Natural Language Processing?
Natural Language Processing faces several challenges, including the ambiguity of natural language, understanding context, dealing with noisy and unstructured data, handling different languages and dialects, and accurately capturing the nuances and subtleties of human language.
What is the role of Machine Learning in Natural Language Processing?
Machine Learning plays a crucial role in Natural Language Processing. It allows NLP systems to learn from large volumes of data, automatically discover patterns, and make predictions or classifications. Supervised learning, unsupervised learning, and reinforcement learning are commonly used in NLP tasks.
Can Natural Language Processing understand multiple languages?
Yes, Natural Language Processing can be applied to multiple languages. However, the complexity and availability of resources in different languages may vary. NLP models can be trained and adapted for specific languages, enabling them to understand and process different languages effectively.
What are the ethical considerations in Natural Language Processing?
Ethical considerations in Natural Language Processing revolve around issues such as privacy, bias, fairness, security, and the responsible use of data. Proper care should be taken to address these concerns, ensuring that the development and deployment of NLP systems do not harm individuals or perpetuate inequality.
Can Natural Language Processing completely replace human language understanding?
No, Natural Language Processing cannot completely replace human language understanding. While NLP has made significant advancements, it still lacks the depth and complexity of human language comprehension. Human judgment, context, and the ability to understand nuances are often necessary for accurate interpretation and meaningful engagement.
Where can I learn more about Natural Language Processing?
There are many resources available to learn about Natural Language Processing. Online courses, books, academic papers, and research articles can provide in-depth knowledge about NLP fundamentals, techniques, and applications. Additionally, joining NLP communities, attending conferences, and networking with experts can offer valuable insights into the field.