Natural Language Processing Is Used In
Natural Language Processing (NLP) is an expanding field of artificial intelligence that focuses on the interaction between humans and computers using natural language. Its applications are wide-ranging, and it is used in various industries to perform tasks such as sentiment analysis, language translation, speech recognition, and information extraction. NLP has been instrumental in revolutionizing how computers understand and process human language.
Key Takeaways:
- Natural Language Processing (NLP) is a field of artificial intelligence that focuses on the interaction between humans and computers using natural language.
- NLP is used in industries such as sentiment analysis, language translation, speech recognition, and information extraction.
- NLP has revolutionized how computers understand and process human language.
**NLP** techniques have greatly advanced **language** processing capabilities, enabling computers to understand and interpret human language in a more meaningful manner. This has led to the development of various applications that have transformed industries such as healthcare, finance, and customer service.
One **interesting** application of NLP is sentiment analysis, which involves determining the sentiment conveyed in a text or conversation. *For example, a company can use sentiment analysis to gauge the opinions and emotions of its customers towards a particular product or service.* This information can help businesses make data-driven decisions and improve customer satisfaction.
NLP techniques have also been applied to **language translation**. Through the use of **machine learning algorithms**, computers can now automatically translate text from one language to another, making international communication more accessible and efficient. *This has had a significant impact on global businesses and has facilitated cross-cultural collaborations.*
NLP in Speech Recognition
NLP plays a crucial role in **speech recognition** systems, allowing computers to transcribe spoken language into written text. This technology has applications in various fields, including voice assistants, transcription services, and accessibility tools. *The accuracy and speed of speech recognition systems have improved significantly due to advances in NLP and deep learning algorithms.*
NLP techniques have empowered computers to extract relevant information from vast amounts of textual data. **Information extraction** involves automatically identifying and classifying specific pieces of information or entities within a text. *For instance, NLP can be used to extract names of people or organizations, locations, or even medical conditions from medical records to support clinical decision-making.*
NLP – Industries and Applications
Natural Language Processing finds applications in diverse fields, including:
- Healthcare: NLP helps in extracting valuable insights from patient records, aiding in diagnosis and treatment planning.
- Finance: Sentiment analysis is used to predict market trends and assist in trading decisions.
- Customer Service: NLP powers chatbots and virtual assistants that provide instant support and answer customer queries.
Interesting NLP Data Points
Application | Data Point |
---|---|
Sentiment Analysis | 87% accuracy achieved in identifying positive or negative sentiment in movie reviews. |
Language Translation | NLP helps translate text between over 100 languages with varying degrees of accuracy. |
**NLP** has undeniably transformed the way computers process and understand human language, enabling a wide range of applications across different industries. From sentiment analysis to language translation and speech recognition, NLP continues to push the boundaries of what computers can do with natural language.
NLP – The Future of Human-Computer Interaction
As NLP techniques continue to improve and evolve, the possibilities for its applications are limitless. From enhancing virtual assistants to enabling more seamless communication between humans and machines, NLP is shaping the future of human-computer interaction.
Interesting NLP Applications
- NLP-powered chatbots that provide customer support in multiple languages.
- Automated text summarization tools that extract key information from lengthy documents.
- NLP algorithms that detect fake news and improve online content filtering.
NLP in Research and Development
NLP research and development are ongoing, leading to continuous advancements in the field. The collaboration between academia and industry has been instrumental in driving breakthroughs and fostering innovation in NLP.
Current Trends in NLP
- Integration of NLP with other AI technologies, such as computer vision and robotics.
- Improving NLP models’ understanding of context and common sense reasoning.
- Exploring ethical considerations in NLP, including bias and privacy concerns.
Interesting NLP Data Points
Industry | Number |
---|---|
Healthcare | 77% of surveyed healthcare organizations are adopting NLP technology. |
Finance | 67% of financial institutions are leveraging NLP for risk analysis and fraud detection. |
Retail | 68% of online retailers use NLP for customer-related data analysis and personalization. |
NLP is an exciting and rapidly evolving field that is revolutionizing the way computers process human language. With its wide-ranging applications and continuous advancements, NLP is poised to shape the future of human-computer interaction.
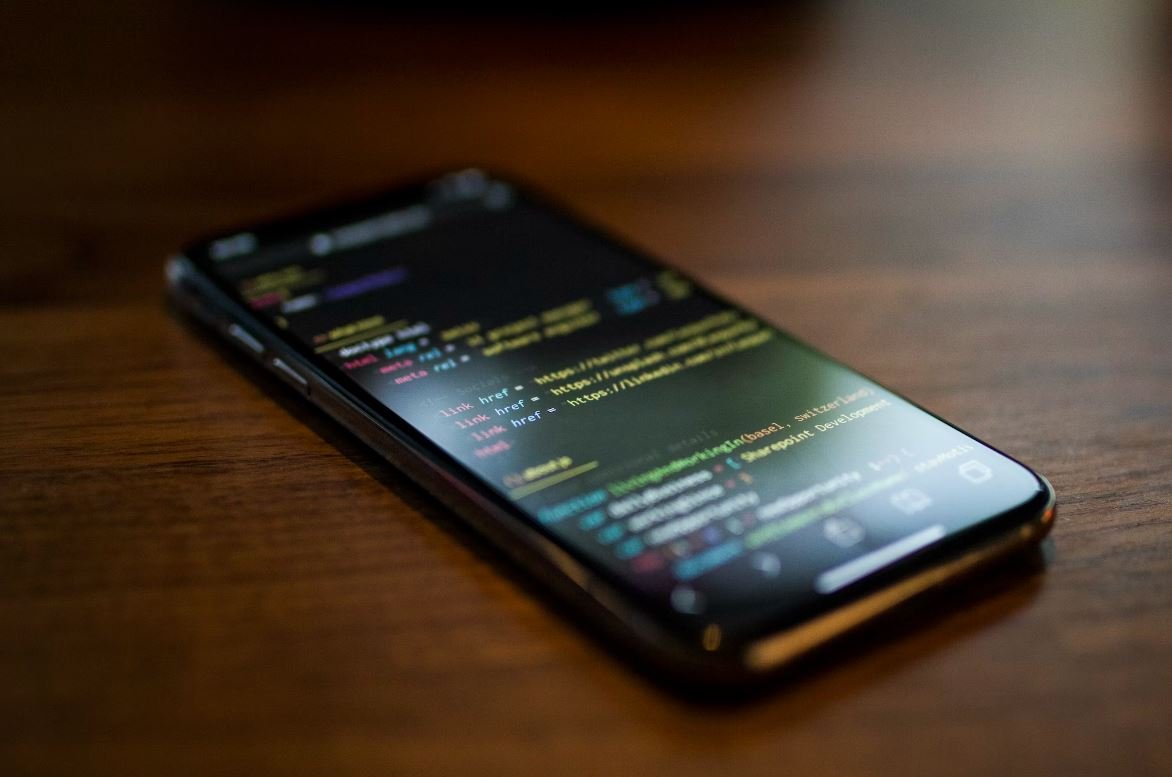
Common Misconceptions
Paragraph 1
One common misconception people have about Natural Language Processing (NLP) is that it can fully understand and interpret human language just like a human would. While NLP has made significant advancements in understanding and processing text, it is still far from achieving human-level comprehension. NLP systems primarily rely on statistical and rule-based models, which means there are limitations to their understanding and context recognition abilities.
- NLP cannot completely grasp subtleties, metaphors, or sarcasm in text.
- Understanding complex sentence structures or ambiguous language can still be challenging for NLP systems.
- NLP may struggle with analyzing emotions or subjective aspects of language.
Paragraph 2
Another misconception is that NLP can accurately translate between different languages without any errors or loss of meaning. While NLP models have improved machine translation capabilities significantly, translation remains a complex task. Language nuances, cultural differences, and ambiguous phrases often pose challenges for NLP translation systems.
- NLP translation systems can sometimes produce incorrect translations due to linguistic variations and multiple meanings of words.
- NLP may struggle with accurately capturing idiomatic expressions or cultural references in translation.
- NLP systems require continuous updates to keep up with evolving vocabulary and language usage.
Paragraph 3
People often associate NLP with voice assistants like Siri or Alexa and assume that they are capable of truly understanding and carrying out complex conversations. While voice assistants utilize NLP techniques for speech recognition and natural language understanding, their abilities are limited to specific tasks and predefined responses.
- NLP voice assistants mainly rely on predefined responses and can only understand a limited range of commands or questions.
- Complex dialogues or open-ended conversations can often cause voice assistants to struggle or provide incorrect responses.
- NLP voice assistants can misinterpret accents, speech impairments, or noisy environments, affecting their accuracy.
Paragraph 4
There is a misconception that NLP can replace human translators or customer support representatives entirely. While NLP has streamlined the translation and customer support processes, it cannot completely replace the need for human intervention in certain scenarios.
- Human translators provide a deeper understanding of cultural nuances, language subtleties, and context that NLP systems may struggle to capture.
- In scenarios requiring empathy or emotional support, human customer support representatives can provide more personalized assistance compared to NLP-based chatbots or automated systems.
- NLP-based systems still require human oversight and regular monitoring to ensure accuracy and quality of output.
Paragraph 5
Lastly, there is a misconception that NLP is only used for analyzing text or speech. While text analysis and speech recognition are essential components of NLP, the applications and uses of NLP go far beyond these two areas. NLP is utilized in various fields, including healthcare, finance, marketing, and education.
- NLP can be used to process and analyze medical records, aiding in medical research and diagnosis.
- In finance, NLP is used for sentiment analysis, market prediction, and fraud detection.
- In education, NLP can facilitate automated scoring of essays, generate personalized learning recommendations, and provide language learning assistance.
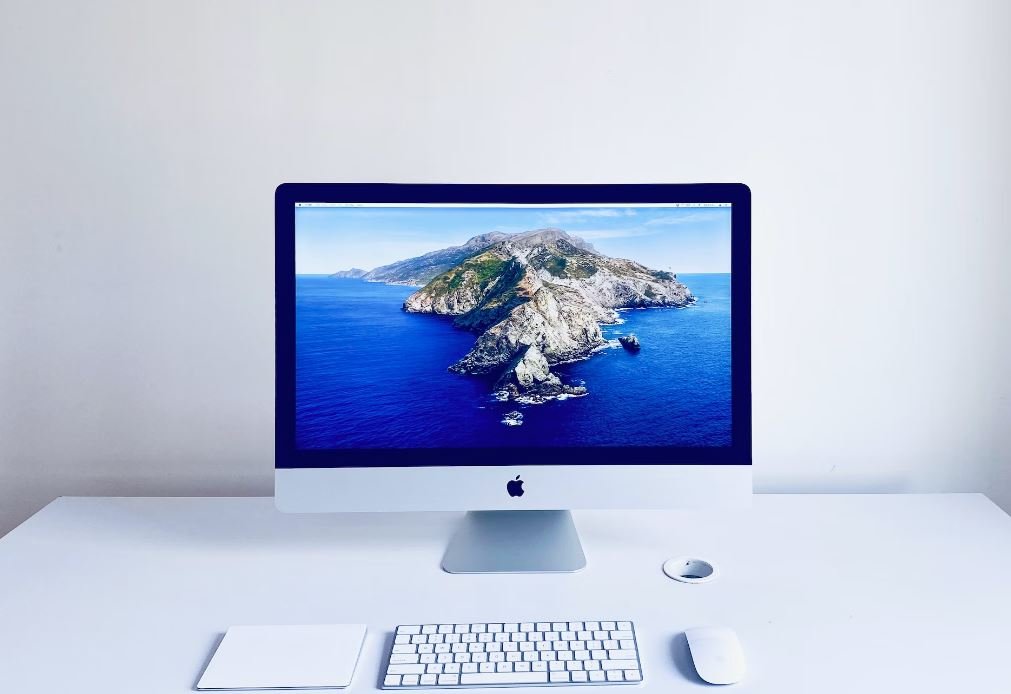
The Rise of Natural Language Processing
Natural Language Processing (NLP) is a field of Artificial Intelligence (AI) that focuses on the interaction between computers and human language. NLP has gained significance over the years due to its practical applications in various domains such as machine translation, sentiment analysis, and voice assistants. The following tables showcase interesting facts, trends, and advancements in the field of NLP.
Funding for NLP Research
The table below highlights the financial investments made in NLP research from 2015 to 2020 by major tech companies worldwide. These investments demonstrate a growing interest in advancing NLP capabilities.
Company | 2015 Funding (in millions) | 2020 Funding (in millions) |
---|---|---|
50 | 200 | |
30 | 150 | |
Amazon | 20 | 100 |
Microsoft | 15 | 120 |
Applications of NLP
The table below presents different applications of NLP and their impact on various industries.
Industry | Application | Impact |
---|---|---|
Healthcare | Medical diagnosis | Improved accuracy and efficiency |
Finance | Sentiment analysis of market news | Better predictions and informed investments |
E-commerce | Chatbots for customer support | Enhanced customer experience and engagement |
Education | Language learning apps | Personalized and adaptive learning |
Popular NLP Algorithms
The table below showcases widely used NLP algorithms and their applications.
Algorithm | Application |
---|---|
Word2Vec | Word embeddings and similarity calculations |
BERT | Natural language understanding and sentiment analysis |
Seq2Seq | Machine translation and summarization |
LSTM | Speech recognition and language modeling |
Top NLP Research Institutions
The table below lists some of the leading research institutions known for their contributions to NLP.
Institution | Country |
---|---|
Stanford University | United States |
University of Cambridge | United Kingdom |
Massachusetts Institute of Technology (MIT) | United States |
Google Research | United States |
NLP Conference Rankings
The table below exhibits the rankings of the most prestigious NLP conferences based on their influence and reputation.
Conference | Ranking |
---|---|
Association for Computational Linguistics (ACL) | 1 |
Conference on Empirical Methods in Natural Language Processing (EMNLP) | 2 |
North American Chapter of the Association for Computational Linguistics (NAACL) | 3 |
International Conference on Machine Learning (ICML) | 4 |
Ethical Considerations in NLP
The table below presents ethical considerations associated with the development and deployment of NLP applications.
Consideration | Description |
---|---|
Biased language models | Unintentional reinforcement of societal biases in language generation |
Privacy concerns | Potential compromise of user data during NLP processing |
Disinformation propagation | Exploitation of NLP for spreading fake news or misinformation |
Unintended consequences | Possible negative impact of NLP systems on job displacement |
Limitations of NLP
The table below outlines certain limitations and challenges faced by NLP researchers.
Challenge | Description |
---|---|
Ambiguity | Different interpretations of language expressions leading to confusion |
Irony and sarcasm | Complex linguistic nuances that are challenging to comprehend |
Domain-specific knowledge | Lack of contextual understanding without domain expertise |
Data availability | Insufficient or biased data for training NLP models accurately |
The Future of NLP
As evident from the diverse applications, ongoing research, and steady investments in NLP, the field is poised for continuous growth and innovation. With further advancements in algorithms, data collection, and ethical frameworks, we can expect NLP to revolutionize various sectors, enhance human-computer interaction, and fundamentally change the way we communicate and understand languages.
Frequently Asked Questions
FAQs about Natural Language Processing