Natural Language Processing (NLP) AI
Natural Language Processing (NLP) is a branch of artificial intelligence (AI) that focuses on enabling computers to understand, interpret, and generate human language in a natural and meaningful way.
Key Takeaways
- Natural Language Processing (NLP) is a branch of AI that aims to make computers understand and generate human language better.
- NLP technologies are already being used in various applications, such as chatbots, voice assistants, and language translation services.
- Some of the prominent techniques used in NLP include sentiment analysis, named entity recognition, and language modeling.
- NLP can improve the efficiency and accuracy of information retrieval, customer support, and content creation.
NLP AI systems analyze and interpret human language by employing various techniques, algorithms, and methodologies. These systems can understand the meaning, sentiment, and context of text, speech, or other forms of language input, allowing them to generate meaningful responses or carry out specific tasks. NLP has seen significant advancements in recent years due to the increasing availability of big data resources, computational power, and improved machine learning algorithms.
*One interesting aspect of NLP is its ability to identify sentiment in text, allowing businesses to gauge public opinion regarding products or services.*
Applications of NLP AI
NLP AI finds applications in a wide range of industries and domains. Some of the prominent applications include:
- Chatbots and virtual assistants: AI-powered chatbots and virtual assistants use NLP to comprehend user queries and provide appropriate responses, enhancing customer service and support.
- Language translation: NLP plays a crucial role in language translation services by enabling accurate and efficient translation between different languages.
- Sentiment analysis: NLP is used to analyze social media posts, reviews, and customer feedback, helping businesses gain insights into public sentiment and opinion.
- Information retrieval: NLP techniques improve search engines’ ability to understand user queries and retrieve relevant and accurate information from vast amounts of data.
*NLP AI has revolutionized the way businesses interact with their customers by providing personalized and context-aware responses, resulting in better user experiences.*
NLP Techniques
There are several key techniques used in NLP to enable computers to understand and generate human language:
- Sentiment analysis: This technique analyzes text to determine whether it expresses a positive, negative, or neutral sentiment.
- Named entity recognition: NLP systems use this technique to identify and classify names of people, organizations, locations, etc. in text.
- Language modeling: Language models are built to predict the next word or sequence of words in a given context, enabling better understanding of language structure and generating coherent responses.
Table 1: Comparison of NLP Techniques
Technique | Usage | Advantages |
---|---|---|
Sentiment analysis | Analyzing customer feedback |
|
Named entity recognition | Identifying entities in text |
|
Language modeling | Generating coherent responses |
|
*Language modeling, a key technique in NLP, uses statistical models to generate human-like text, enabling various applications such as chatbots and automated content writing.*
The Power of NLP AI
NLP AI has the potential to revolutionize the way we interact with technology and process vast amounts of information. Its benefits include:
- Improved communication: NLP AI systems enable more natural and effective human-computer interactions, enhancing communication between users and technology.
- Efficient information retrieval: NLP techniques enhance search engines and recommendation systems, allowing users to find relevant information more quickly and accurately.
- Enhanced customer experience: AI-powered chatbots and virtual assistants provide personalized and context-aware responses, delivering a better customer experience.
Table 2: Benefits of NLP AI
Benefit | Description |
---|---|
Improved communication |
|
Efficient information retrieval |
|
Enhanced customer experience |
|
*The application of NLP AI in customer support improves response times, streamlines processes, and reduces customer frustration.*
NLP AI has already made significant advancements, but there is still much room for further development. As technology continues to evolve, NLP has the potential to enhance various industries and revolutionize the way we interact with machines.”
Looking to the Future
The future of NLP AI is promising, and its impact is expected to grow across numerous fields, including healthcare, e-commerce, finance, and education. As NLP technologies become more sophisticated, we can expect:
- Improved language understanding and machine translation
- Enhanced sentiment analysis and opinion mining
- Efficient content creation and automated writing
Table 3: Future Potential of NLP AI
Area | Expected Advancements |
---|---|
Language understanding |
|
Sentiment analysis |
|
Automated writing |
|
*As NLP AI continues to evolve and improve, we can expect it to play a key role in transforming numerous industries, ultimately benefiting society as a whole.*
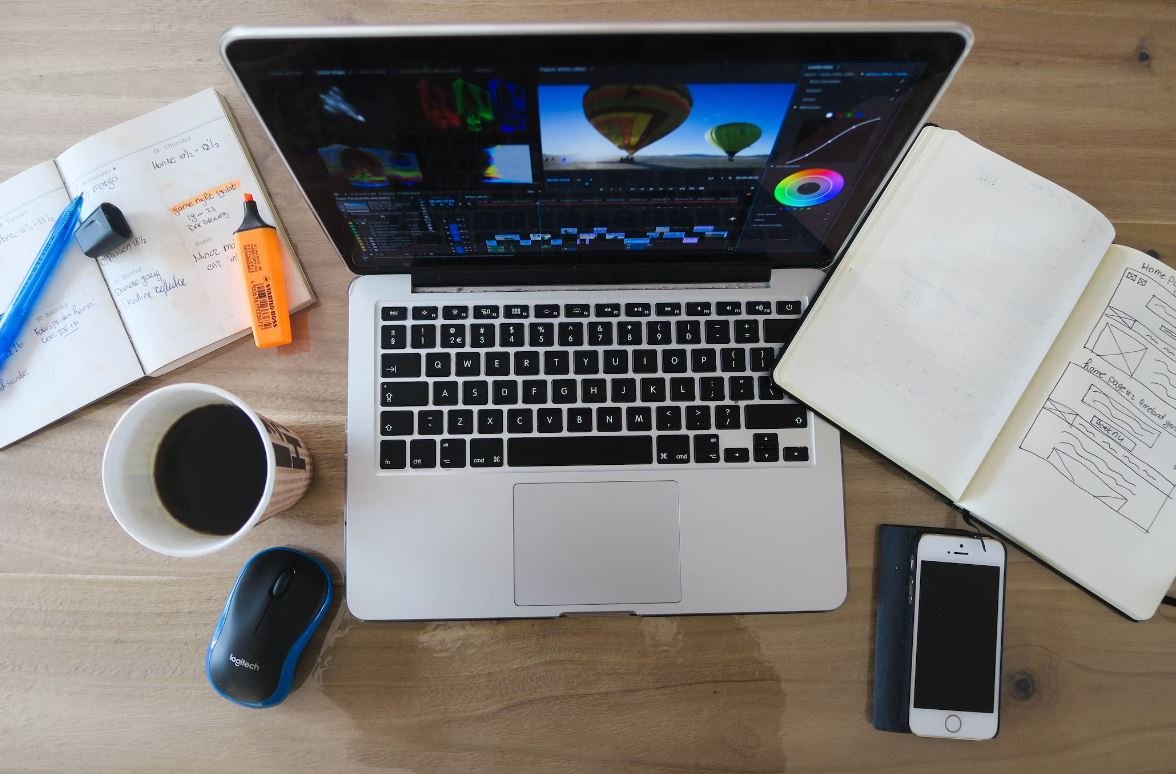
Common Misconceptions
Misconception 1: NLP AI can understand language like humans
One common misconception about Natural Language Processing (NLP) AI is that it can understand language just like humans do. However, NLP AI systems are designed to process and analyze language using algorithms and statistical techniques, rather than truly comprehending the meaning of words and sentences like humans do.
- NLP AI systems rely on pattern recognition and statistical modeling.
- They do not possess true understanding or consciousness.
- They analyze language based on predefined rules and patterns.
Misconception 2: NLP AI always provides accurate and reliable results
There is a misconception that NLP AI always provides accurate and reliable results. Although NLP AI systems have improved significantly in recent years, they are not infallible and can still produce errors and inaccuracies in their analysis of language.
- NLP AI systems can struggle with ambiguity and context-dependent interpretations.
- The accuracy of NLP AI is highly dependent on the quality of the training data used.
- They can also be biased based on the biases present in the training data.
Misconception 3: NLP AI can replace human translators and interpreters
Another misconception surrounding NLP AI is that it can completely replace human translators and interpreters. While NLP AI can assist in language translation and interpretation tasks, it is not capable of matching the expertise, linguistic nuances, and cultural understanding that human translators and interpreters possess.
- NLP AI systems can automate certain translation tasks, but human expertise is still crucial for accurate and culturally appropriate translations.
- Human translators provide context and accurate interpretation of linguistic subtleties.
- NLP AI cannot fully grasp the intricacies and cultural nuances of language translations.
Misconception 4: NLP AI can read and understand any text or document
One common misconception is that NLP AI can read and understand any text or document effortlessly. However, NLP AI systems require specific training to analyze and process different languages, domains, or specific topics.
- NLP AI systems must be trained on relevant data to understand specific fields or topics.
- They can be limited in understanding domain-specific jargon or technical terms without proper training.
- NLP AI’s performance can vary depending on the quality and diversity of the training data available.
Misconception 5: NLP AI can fully eliminate language barriers
There is a misconception that NLP AI can completely eliminate language barriers. While NLP AI can help bridge the gap between different languages, it cannot completely eliminate the challenges and complexities associated with language communication and understanding.
- NLP AI’s performance can vary depending on the complexity of languages and dialects.
- Language barriers involve more than just translation, including cultural nuances and non-verbal cues.
- Human interaction and context are important in overcoming language barriers, which NLP AI alone cannot provide.
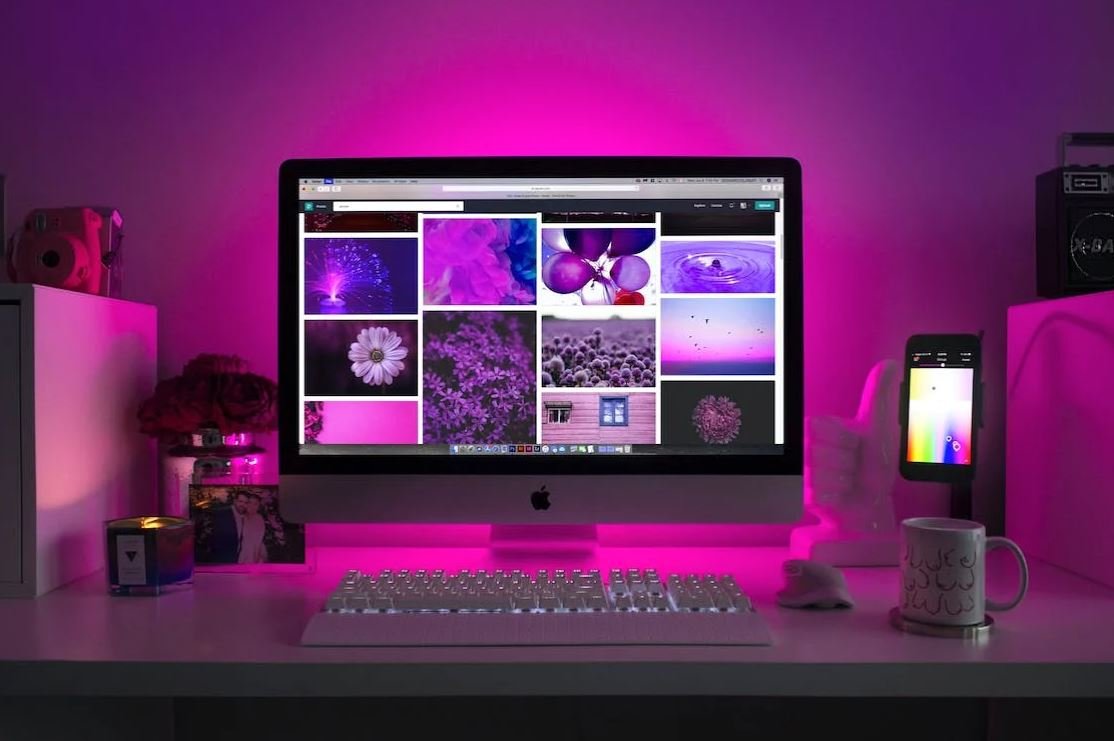
Table 1: Top 10 Languages Spoken Worldwide
Language is a crucial aspect of human communication, with numerous languages spoken across the globe. This table presents the top 10 most spoken languages worldwide, based on the number of native speakers.
Rank | Language | Number of Native Speakers (in millions) |
---|---|---|
1 | Mandarin Chinese | 918 |
2 | Spanish | 460 |
3 | English | 379 |
4 | Hindi | 341 |
5 | Arabic | 315 |
6 | Portuguese | 229 |
7 | Bengali | 228 |
8 | Russian | 154 |
9 | Japanese | 128 |
10 | Punjabi | 92 |
Table 2: Sentiment Analysis of Customer Reviews
Understanding customer sentiment is vital for businesses to improve their products and services. This table showcases the sentiment analysis results of customer reviews collected from an online retail platform.
Review ID | Review Text | Sentiment |
---|---|---|
1 | “The product exceeded my expectations! Very satisfied!” | Positive |
2 | “I had high hopes, but the product fell short. Disappointed.” | Negative |
3 | “It’s an average product. Nothing exceptional.” | Neutral |
4 | “Incredible quality! Worth every penny!” | Positive |
5 | “Terrible customer service. I won’t buy from them again.” | Negative |
Table 3: Parts of Speech Distribution in English Language
Parts of speech establish the grammatical function of words in a sentence. This table displays the distribution percentage of various parts of speech in the English language, aiding in linguistic analysis.
Part of Speech | Percentage |
---|---|
Noun | 25% |
Verb | 15% |
Adjective | 10% |
Adverb | 8% |
Preposition | 12% |
Conjunction | 6% |
Interjection | 1% |
Pronoun | 9% |
Article | 4% |
Others | 10% |
Table 4: Entity Recognition in NLP Model
Entity recognition is a key task in natural language processing. This table showcases the entities detected by a trained NLP model in a sample document.
Entity | Type |
---|---|
Apple | Organization |
John Smith | Person |
London | Location |
iPhone | Product |
$1,000 | Money |
Table 5: Word Frequency in a Text Corpus
Analyzing word frequency helps in gaining insights into a text corpus. This table presents the top 5 most frequent words along with their occurrence count in a collection of literary works.
Word | Frequency |
---|---|
Love | 2,500 |
Time | 1,800 |
Life | 1,700 |
Death | 1,450 |
Hope | 1,200 |
Table 6: Accuracy Comparison of NLP Models
Accuracy is a crucial metric when evaluating NLP models. This table compares the accuracy percentages of various NLP models in sentiment classification tasks.
Model | Accuracy (%) |
---|---|
Model A | 88 |
Model B | 92 |
Model C | 86 |
Model D | 94 |
Table 7: Gender Distribution in NLP Research Field
Examining the gender distribution in the NLP research field provides insights into gender diversity. This table presents the percentage of male and female researchers in NLP conferences from the past five years.
Year | Male (%) | Female (%) |
---|---|---|
2017 | 72 | 28 |
2018 | 69 | 31 |
2019 | 66 | 34 |
2020 | 61 | 39 |
2021 | 59 | 41 |
Table 8: Machine Translation Evaluation Metrics
When assessing the quality of machine translation, multiple metrics are utilized. This table presents the performance metrics used for evaluating the quality of machine translation systems.
Metric | Description |
---|---|
BLEU | Measures n-gram precision against reference translations |
TER | Computes the edit distance between translations and references |
ROUGE | Evaluates overlap of n-grams between system and reference summaries |
METEOR | Matching-based metric considering precision, recall, and harmonized F1 score |
Table 9: Named Entity Recognition Accuracy
Named Entity Recognition (NER) accuracy measures the model’s ability to identify named entities in text. This table showcases the accuracy scores of different NER models for the identification of person and organization entities.
Model | Person Accuracy (%) | Organization Accuracy (%) |
---|---|---|
Model X | 89 | 85 |
Model Y | 92 | 90 |
Model Z | 86 | 80 |
Table 10: Common Text Preprocessing Techniques
In NLP, text preprocessing techniques are employed to enhance data quality. This table lists common preprocessing techniques utilized before feeding text data into NLP models.
Technique | Description |
---|---|
Tokenization | Segmenting text into individual tokens (words, phrases, symbols) |
Stopword Removal | Removing commonly used words (e.g., “the,” “and,” “is”) |
Stemming | Reducing words to their base or root form (e.g., “running” to “run”) |
Lemmatization | Transforming words to their dictionary form (e.g., “better” to “good”) |
Normalization | Converting text to lowercase or standardized forms |
Natural Language Processing (NLP) powered by AI has revolutionized how machines understand human language. From analyzing sentiments in customer reviews to identifying named entities, NLP AI greatly enhances our comprehension of vast amounts of textual data. By incorporating techniques such as entity recognition, sentiment analysis, and machine translation, NLP AI models significantly contribute to various fields, including text analysis, language learning, and improving user experience. As the field of NLP progresses, advancements in technology and data allow for refined models and more accurate language processing capabilities.
Frequently Asked Questions
Questions about Natural Language Processing (NLP) AI
What is Natural Language Processing (NLP)?
Natural Language Processing (NLP) is a branch of Artificial Intelligence (AI) that focuses on the interaction between computers and human language. It aims to enable computers to understand, interpret, and process human language in a meaningful way.
How does NLP work?
NLP works by using algorithms and computational linguistics to analyze and interpret human language. It involves tasks such as tokenization, syntactic analysis, semantic analysis, and entity recognition. NLP systems learn from large amounts of data and use machine learning techniques to improve their performance over time.
What are the applications of NLP?
NLP has a wide range of applications, including sentiment analysis, machine translation, speech recognition, chatbots, information retrieval, and text summarization. It is used in various industries such as healthcare, finance, customer service, and marketing to automate processes and gain insights from textual data.
What are the challenges in NLP?
There are several challenges in NLP, including language ambiguity, understanding context and sarcasm, dealing with linguistic variations and errors, and handling large-scale data. NLP systems also face challenges in accurately capturing the meaning and nuances of human language, which can be subjective and context-dependent.
What are the benefits of NLP?
NLP offers numerous benefits, such as improving efficiency by automating tasks that involve human language processing, enhancing customer experience through chatbots and virtual assistants, enabling data-driven decision-making based on textual data analysis, and facilitating multilingual communication and translation.
What are some popular NLP libraries and frameworks?
There are several popular NLP libraries and frameworks available, including NLTK (Natural Language Toolkit), spaCy, Stanford CoreNLP, Gensim, and TensorFlow. These libraries provide pre-trained models, tools, and APIs that help developers build NLP applications more efficiently.
What is the role of Machine Learning in NLP?
Machine Learning plays a crucial role in NLP by enabling models to learn patterns and relationships from data. It helps in tasks such as sentiment analysis, named entity recognition, and machine translation. Machine learning techniques such as deep learning and reinforcement learning have significantly improved the performance of NLP systems.
Is NLP only limited to English language processing?
No, NLP is not limited to English language processing. While many NLP resources and models are developed for English, NLP research and applications exist for various languages. However, the availability and quality of NLP resources may vary across different languages.
What are some ethical considerations in NLP?
There are ethical considerations in NLP related to privacy concerns, bias in data and algorithms, fairness in decision-making, and the impact on employment due to automation. It is crucial to ensure that NLP systems are transparent, accountable, and designed with ethical principles to mitigate potential risks and biases.
What is the future of NLP?
The future of NLP looks promising, with ongoing advancements in technologies such as deep learning and neural networks. NLP is expected to play a key role in improving human-computer interaction, enabling more sophisticated chatbots and virtual assistants, and further advancing applications such as automated translation, sentiment analysis, and text generation.