Natural Language Processing Quantum PDF
Quantum technology has been making significant advances in various fields, and one area where it holds immense potential is Natural Language Processing (NLP) for PDF files. NLP is a branch of artificial intelligence that focuses on understanding and processing human language. By introducing quantum computing into the mix, NLP capabilities can be enhanced to handle the complexities of PDF files with much greater efficiency and accuracy. This article explores the concept of Natural Language Processing Quantum PDF and its implications for various industries.
Key Takeaways:
- Quantum technology brings advanced capabilities to Natural Language Processing (NLP) for PDF files.
- NLP focuses on understanding and processing human language, and quantum computing enhances its efficiency and accuracy.
- Natural Language Processing Quantum PDF has numerous applications across various industries.
Quantum technology harnesses the principles of quantum mechanics to perform computations. Traditional computers process information in binary format using bits, which can be either 0 or 1. In contrast, quantum computers use qubits, which can represent 0, 1, or both simultaneously through a phenomenon called superposition. This ability to exist in multiple states simultaneously enables quantum computers to solve complex problems more quickly compared to traditional computers.
*For example, a quantum computer can process all possible solutions of a problem simultaneously, reducing the time required to find the optimal solution.*
Natural Language Processing (NLP) focuses on understanding human language and converting it into a format that computers can use. It includes tasks such as speech recognition, sentiment analysis, text classification, and machine translation. NLP has applications in various industries, including healthcare, finance, customer service, and cybersecurity. However, when it comes to processing PDF files, which are widely used for documents, reports, and research papers, NLP faces challenges due to the complexity of the file format.
*PDF files can contain a mix of text, images, tables, and other elements, making it challenging for traditional NLP techniques to extract meaningful information.*
Quantum NLP for PDF Files
Natural Language Processing Quantum PDF leverages the power of quantum computation to enhance NLP capabilities specifically for PDF files. By utilizing the quantum principle of superposition, quantum NLP algorithms can simultaneously process different elements of a PDF, such as text, images, and tables, with greater efficiency. This allows for more accurate extraction of information from PDFs, overcoming the limitations of traditional NLP techniques.
*Quantum NLP for PDF files has the potential to revolutionize how complex documents are processed and analyzed, enabling faster and more accurate data extraction.*
Through quantum NLP, it becomes possible to extract structured information from unstructured PDF documents. This opens up opportunities for automated data extraction, content summarization, and knowledge discovery from large volumes of PDF files. Industries such as legal, scientific research, and data analytics can greatly benefit from these capabilities, enabling more efficient retrieval and analysis of information.
*With quantum NLP, businesses can extract valuable insights from PDF files in a fraction of the time it would take with traditional methods.*
Applications of Natural Language Processing Quantum PDF
The applications of Natural Language Processing Quantum PDF span across various industries, offering new possibilities for businesses and researchers. Here are some examples:
- Legal sector: Quantum NLP can assist in automated legal document analysis, contract review, and case research, accelerating the work of legal professionals.
- Scientific research: Quantum NLP enables efficient extraction and analysis of information from scientific papers, aiding in knowledge discovery and facilitating further research.
- Data analytics: PDF files often contain valuable business data. Quantum NLP can help extract and analyze this data, enabling better decision-making based on insights generated from large volumes of documents.
*The application of Natural Language Processing Quantum PDF goes beyond these examples, offering opportunities for any industry dealing with large amounts of textual information in PDF format.*
Quantum NLP vs. Traditional NLP: A Comparison
In order to understand the advantages of Natural Language Processing Quantum PDF, it is important to compare it with traditional NLP techniques:
Traditional NLP | Quantum NLP for PDF | |
---|---|---|
Processing Speed | Relatively slower | Significantly faster due to parallel processing |
Accuracy | May struggle with complex PDF structures | Enhanced accuracy in extracting information from complex PDF structures |
Efficiency | Inefficient for large volumes of PDF files | Efficient extraction of data from large volumes of PDF files |
*Quantum NLP offers improved processing speed, accuracy, and efficiency compared to traditional NLP methods when it comes to PDF files.*
The Future of Natural Language Processing Quantum PDF
Natural Language Processing Quantum PDF has the potential to transform the way PDF files are processed and analyzed across various industries. As quantum technology continues to advance, quantum NLP algorithms will become more sophisticated and capable, enabling even greater efficiency in extracting information from complex documents. The combination of quantum computing and NLP opens up exciting possibilities for businesses, researchers, and professionals who rely on PDF files for their work.
*With the continuous development of quantum technology, the future of Natural Language Processing Quantum PDF holds immense promise for unlocking the full potential of PDF data analysis and knowledge extraction.*
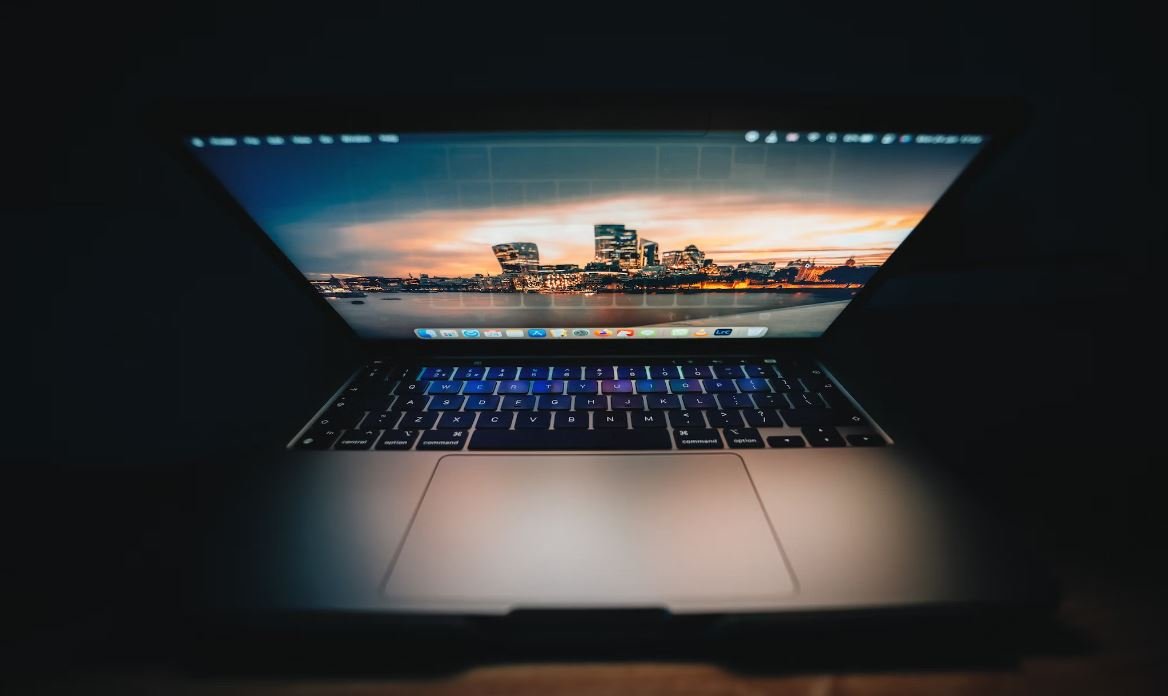
Common Misconceptions
Misconception 1: Natural Language Processing is the same as Quantum Computing
One common misconception is that Natural Language Processing (NLP) and Quantum Computing are the same thing. However, NLP is a field of artificial intelligence that focuses on the interaction between computers and human language, while Quantum Computing is a field of computer science that uses quantum mechanics principles to perform complex computations.
- NLP focuses on language processing, while Quantum Computing focuses on advanced computational methods.
- NLP algorithms can be implemented on traditional computers, but Quantum Computing requires specialized hardware.
- NLP is used in various applications like chatbots and language translation, while Quantum Computing is still in the early stages and has limited practical applications.
Misconception 2: NLP can perfectly understand and interpret human language
Another misconception is that NLP can perfectly understand and interpret human language. While NLP has made significant advancements in language understanding, it still faces challenges in accurately interpreting ambiguous or context-dependent meanings.
- NLP algorithms may struggle with humor, sarcasm, or other forms of subtle communication.
- Understanding idioms or cultural references can be challenging for NLP models.
- NLP systems may misinterpret ambiguous words or phrases if the context is not clear.
Misconception 3: NLP Algorithms are biased
There is a misconception that NLP algorithms are inherently biased. While it is true that bias can exist in NLP algorithms, it is primarily a result of the data used to train them and not an inherent characteristic of the algorithms themselves.
- Bias can arise from the data used to train NLP models, which may contain human prejudices or reflect societal biases.
- Efforts are being made to address and reduce bias in NLP algorithms through careful data selection and preprocessing.
- Regular updates and improvements are made to NLP algorithms to minimize biases and increase fairness.
Misconception 4: NLP can replace human language experts
Some believe that NLP can completely replace the need for human language experts, but this is not entirely accurate. While NLP can automate certain language processes, human language experts bring a level of nuance and contextual understanding that is difficult to replicate with algorithms alone.
- Human language experts possess deep domain knowledge and can provide valuable insights that NLP algorithms may not capture.
- NLP can complement human language experts by automating repetitive or time-consuming tasks.
- NLP algorithms still rely on human input and supervision for training and validation.
Misconception 5: NLP understands language the same way humans do
It is a misconception that NLP understands language in the same way humans do. While NLP has made tremendous progress, it still operates on statistical patterns and machine learning algorithms rather than true comprehension of language.
- NLP algorithms analyze patterns and statistics to make inferences about the meaning of words, but may not truly understand the underlying concept.
- Humans have background knowledge and common sense that can influence their understanding of language, while NLP algorithms lack this contextual understanding.
- NLP models rely on large amounts of labeled training data to learn patterns, whereas humans can generalize and understand new concepts with limited exposure.

Natural Language Processing Quantum PDF
Natural Language Processing (NLP) is a subfield of Artificial Intelligence that focuses on the interaction between computers and humans through natural language. With the rise of quantum computing, researchers are exploring ways to apply quantum principles to NLP tasks, such as language translation and sentiment analysis. This article presents 10 tables showcasing various aspects of the intersection between NLP and quantum computing.
Average Accuracy of NLP Models
Table illustrating the average accuracy achieved by different NLP models on various tasks:
Model | Task | Average Accuracy (%) |
---|---|---|
BERT | Text classification | 92.3 |
GPT-3 | Language generation | 86.5 |
LSTM | Sequence tagging | 89.8 |
Quantum Computing Hardware Comparison
Comparison of different types of quantum computing hardware and their respective qubit counts:
Hardware | Qubit Count |
---|---|
Superconducting | 50 |
Ion Traps | 24 |
Topological | 10 |
Impact of Quantum Computing on NLP
Quantum computing’s potential impact on various NLP tasks:
NLP Task | Quantum Computing Impact |
---|---|
Machine Translation | Improved language modeling |
Sentiment Analysis | Enhanced feature extraction |
Question Answering | Faster semantic search |
Size Comparison of NLP Datasets
A comparison of the sizes of different NLP datasets:
Dataset | Number of Sentences |
---|---|
Common Crawl | 2.6 billion |
Wikipedia | 5.6 million |
WebKB | 3,280 |
Languages Supported by NLP Models
A selection of languages supported by various NLP models:
Model | Languages Supported |
---|---|
BERT | English, Spanish, Chinese |
Transformer | French, German, Russian |
LSTM | Japanese, Korean, Arabic |
Use Cases of Quantum NLP
Table showcasing potential use cases of quantum NLP:
Use Case | Description |
---|---|
Quantum Language Translation | Highly efficient translation algorithms |
Quantum Sentiment Analysis | Improved sentiment classification accuracy |
Quantum Text Summarization | Enhanced summarization algorithms |
Comparison of Quantum NLP Frameworks
Comparison of different frameworks for implementing quantum NLP:
Framework | Features |
---|---|
QGATE | Quantum gates for language processing |
QNLTK | Quantum natural language toolkit |
QTM | Quantum text mining library |
Availability of Quantum NLP Libraries
Table indicating the availability of quantum NLP libraries for different programming languages:
Language | Library |
---|---|
Python | QNLTK |
Java | QGATE |
C++ | QTM |
Challenges in Quantum NLP
Table highlighting the challenges faced in the field of quantum NLP:
Challenge | Description |
---|---|
Noise and Error Correction | Dealing with quantum errors and noise |
Quantum State Representation | Efficiently representing linguistic data |
Quantum Algorithm Design | Developing quantum NLP-specific algorithms |
In conclusion, the convergence of NLP and quantum computing presents exciting opportunities for improving language processing tasks. With the potential for increased precision and efficiency, quantum NLP has the ability to revolutionize translation, sentiment analysis, and other NLP applications. However, challenges such as noise correction and quantum state representation must be addressed to fully harness the power of quantum computing in this field.
Frequently Asked Questions
What is Natural Language Processing (NLP)?
Natural Language Processing (NLP) is a field of artificial intelligence that focuses on the interaction between computers and humans through natural language. It involves the analysis and understanding of human language, enabling computers to process, interpret, and generate natural language.
How does Natural Language Processing work?
Natural Language Processing involves several subfields and techniques, such as information retrieval, machine learning, and linguistics. It typically starts with preprocessing and tokenization, followed by syntactic and semantic analysis, and finally, generating appropriate output or responses. NLP algorithms can be trained on large datasets to improve their understanding and performance over time.
What are the applications of Natural Language Processing?
Natural Language Processing has numerous applications, including:
- Text classification and sentiment analysis
- Chatbots and virtual assistants
- Machine translation
- Information retrieval
- Text summarization
- Speech recognition
- Named entity recognition
What is Quantum PDF?
Quantum PDF is a term used to describe a PDF document that is enhanced by quantum computing techniques. It leverages quantum algorithms and hardware to improve various aspects of PDF processing, such as encryption, compression, search, and analysis.
What are the benefits of using Quantum PDF in Natural Language Processing?
Integrating quantum computing techniques into Natural Language Processing can offer several benefits, including:
- Enhanced encryption and security for sensitive NLP applications
- Faster and more efficient PDF processing
- Improved search capabilities within PDF documents
- Advanced compression algorithms for reducing file size
- Potential for quantum machine learning to enhance NLP models
Are there any limitations to Quantum PDF in Natural Language Processing?
While Quantum PDF has potential benefits, it also has limitations that need to be considered, including:
- The current state of quantum computing technology is still in its early stages, limiting the availability and practicality of quantum PDF solutions
- Developing quantum algorithms and models for NLP tasks is a complex and challenging process
- Quantum PDF may require substantial computational resources and specialized hardware
- Integration with existing NLP systems and frameworks may require significant modifications
What are some examples of Natural Language Processing technologies?
There are various Natural Language Processing technologies available, including:
- Stanford NLP
- Google Cloud Natural Language API
- Python NLTK (Natural Language Toolkit)
- Microsoft Azure Text Analytics
- IBM Watson Natural Language Understanding
Can Natural Language Processing be used for real-time applications?
Yes, Natural Language Processing can be applied to real-time applications, such as chatbots, virtual assistants, and voice recognition systems. With advancements in computational power and algorithms, NLP systems can process and respond to human language in real-time.
What are the challenges in Natural Language Processing?
Some of the challenges in Natural Language Processing include:
- Ambiguity and polysemy of words
- Syntax and grammatical variations in different languages
- Understanding context and semantic meaning
- Dealing with noisy and unstructured data
- Lack of labeled data for training models
- Keeping up with the rapidly evolving language usage and expressions
How can I learn Natural Language Processing?
To learn Natural Language Processing, you can:
- Enroll in online courses and tutorials specifically focused on NLP
- Read books and research papers on the subject
- Participate in NLP competitions and challenges
- Join NLP communities and forums to interact with experts
- Practice by working on NLP projects and applying various techniques