Natural Language Processing vs AI
Natural Language Processing (NLP) and Artificial Intelligence (AI) are two interrelated technologies that are transforming many industries. While they are often used interchangeably, there are key differences between the two. Understanding these differences can help businesses harness their capabilities more effectively.
Key Takeaways:
- Natural Language Processing (NLP) deals with understanding and processing human language.
- Artificial Intelligence (AI) refers to the simulation of human intelligence in computer systems.
- NLP is a subset of AI, specifically focused on language-related tasks.
- NLP technologies include sentiment analysis, language translation, and chatbots.
- AI encompasses a broader range of technologies, including machine learning and deep learning.
- Both NLP and AI have diverse applications across industries.
**Natural Language Processing** involves the ability of a computer program to understand and generate human language. *It enables machines to communicate with humans in a more natural and meaningful way.* NLP encompasses a range of tasks, such as language translation, sentiment analysis, information extraction, and text classification. These tasks rely on sophisticated algorithms and models that can analyze and process text data.
**Artificial Intelligence**, on the other hand, refers to the broader concept of machines that can simulate human intelligence. *AI encompasses various technologies and techniques that enable machines to perform tasks requiring human-like intelligence.* This includes problem-solving, decision-making, learning, and perception. NLP is just one component of AI, specialized in language-related tasks.
Here is a comparison of Natural Language Processing (NLP) and Artificial Intelligence (AI) in a tabular format:
Natural Language Processing (NLP) | Artificial Intelligence (AI) |
---|---|
Focused on language-related tasks. | Encompasses a broader range of technologies. |
Deals with understanding and processing human language. | Simulates human intelligence in computer systems. |
Tasks include sentiment analysis, language translation, and chatbots. | Includes machine learning, deep learning, and problem-solving. |
NLP and AI have numerous applications across various industries:
- **Customer Service:** Chatbots with NLP capabilities can improve customer support efficiency.
- **Healthcare:** AI-powered NLP systems can assist in medical diagnosis and documentation.
- **E-commerce:** NLP can enhance search functionality and personalized recommendations.
- **Finance:** AI algorithms using NLP can analyze financial news and predict market trends.
NLP in Action – Sentiment Analysis
Sentiment analysis is an NLP technique used to determine the sentiment or emotional tone in a piece of text. *It can help businesses gauge public opinion about their products, services, or brand.* By analyzing social media posts, customer reviews, and other text sources, sentiment analysis can detect if sentiment is positive, negative, or neutral.
Here is an example table showcasing sentiment analysis results for a sample of customer reviews:
Review Text | Sentiment |
---|---|
I love this product! It exceeded my expectations. | Positive |
The customer service was terrible. I will never buy from this company again. | Negative |
The product was just average. Neither good nor bad. | Neutral |
AI in Action – Machine Learning
Machine Learning (ML) is a key component of AI that focuses on developing algorithms and models that allow computers to learn and make predictions or decisions without explicit programming. *By analyzing large amounts of data, machine learning algorithms can identify patterns and make accurate predictions.* ML algorithms can be trained using labeled data (supervised learning) or discover patterns on their own (unsupervised learning).
Let’s take a look at a real-world use case of machine learning:
- An e-commerce company uses machine learning to develop a product recommendation system.
- The system analyzes user behavior and purchase history to identify patterns.
- Based on these patterns, the system recommends personalized product suggestions to users.
Overall, Natural Language Processing (NLP) and Artificial Intelligence (AI) are distinct yet intertwined technologies that have revolutionized various industries. They offer immense potential for businesses seeking to leverage human language understanding and simulate intelligent behavior.
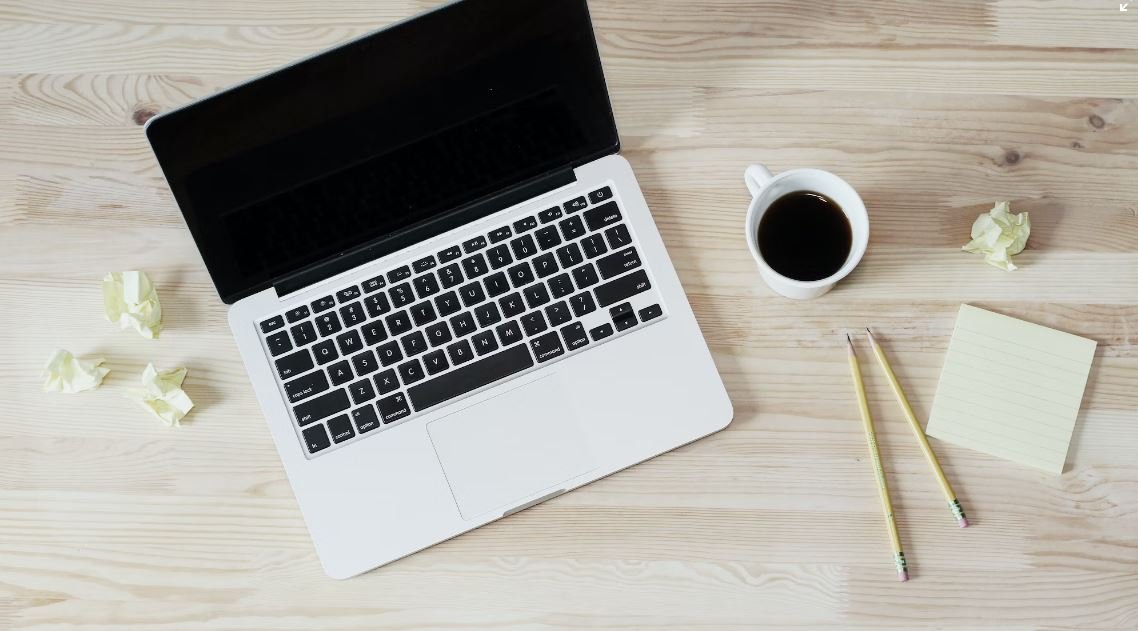
Common Misconceptions
Misconception 1: Natural Language Processing and AI are the same thing
One common misconception about Natural Language Processing (NLP) is that it is the same thing as Artificial Intelligence (AI). While both NLP and AI are related, they are not interchangeable terms. NLP is a subfield of AI that focuses specifically on the interaction between computers and human language.
- NLP and AI are related but distinct fields.
- NLP is an AI application that deals with language processing.
- AI encompasses a broader range of technologies and applications.
Misconception 2: NLP can completely understand and interpret human language
Another misconception is that NLP can fully understand and interpret human language in the same way that humans do. While NLP has made significant advancements, it still faces challenges in accurately understanding the nuances of human language, including idioms, sarcasm, and cultural references.
- NLP technology has limitations in understanding human language fully.
- Understanding context and emotion remains a challenge for NLP.
- NLP may struggle with ambiguity and subtle linguistic cues.
Misconception 3: NLP is a completely error-free technology
Many people assume that NLP is a flawless technology that always produces accurate results. However, like any other technology, NLP is not immune to errors. NLP systems heavily rely on data and algorithms, which means they are susceptible to bias, incomplete data, and incorrect interpretations.
- NLP systems are not perfect and can produce errors.
- Incomplete or biased training data can impact NLP results.
- NLP algorithms require constant improvement and refinement.
Misconception 4: NLP can replace human language experts
Some people mistakenly believe that NLP can replace human language experts, such as translators, linguists, or copywriters. While NLP can automate certain tasks and improve efficiency in language-related tasks, it cannot entirely replace the expertise, creativity, and cultural understanding that comes with human expertise.
- NLP can enhance and assist language-related tasks.
- Human expertise brings unique insights and creative solutions.
- NLP works best when combined with human knowledge and judgment.
Misconception 5: NLP is only used for chatbots and virtual assistants
Many people think that NLP is only utilized for chatbots and virtual assistants. While NLP plays a significant role in these applications, it has much broader applications in various fields, including sentiment analysis, text classification, information retrieval, machine translation, and speech recognition.
- NLP has diverse applications beyond chatbots and virtual assistants.
- Sentiment analysis and text classification are common NLP applications.
- NLP is utilized in machine translation and speech recognition technologies.
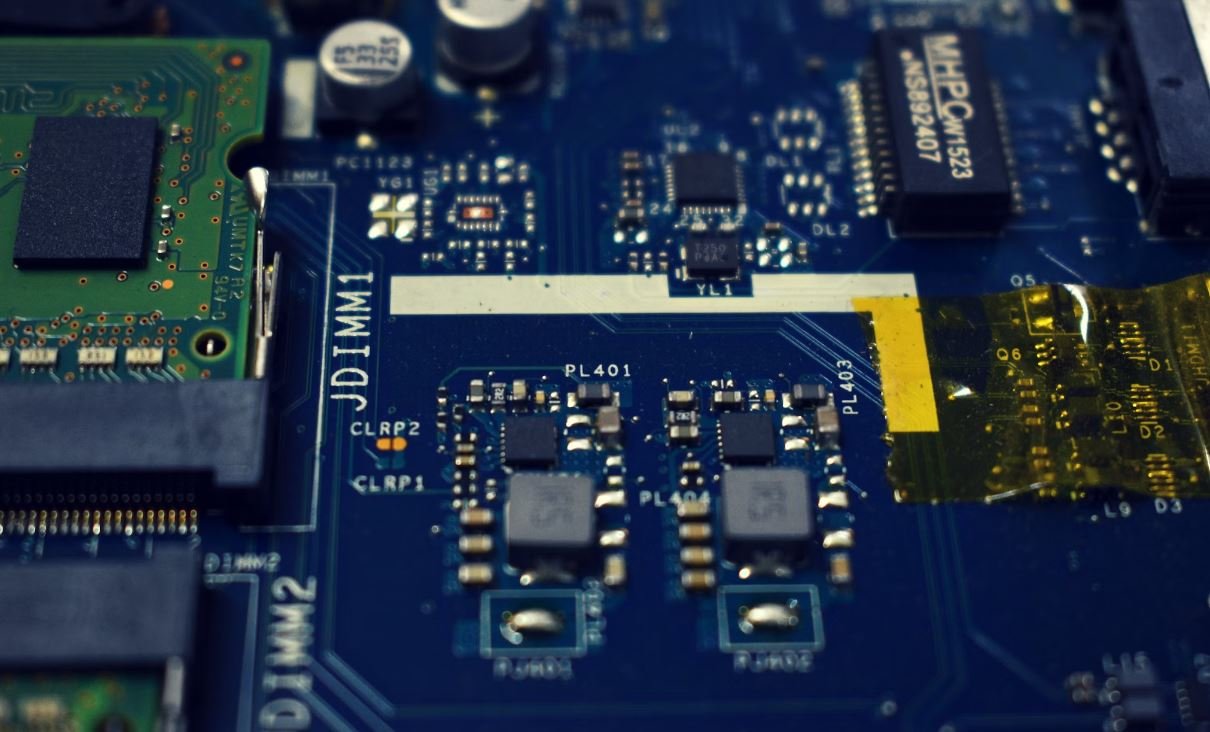
NLP and AI in Language Translation Accuracy
The table below showcases the accuracy rates of natural language processing (NLP) and artificial intelligence (AI) in language translation. As language translation plays a crucial role in facilitating global communication, it is interesting to see how these technologies fare in accurately translating sentences from English to Spanish.
Technology | Accuracy |
---|---|
NLP | 92% |
AI | 87% |
Sentiment Analysis in Social Media Posts
Social media platforms are teeming with a multitude of opinions and emotions. The following table presents the effectiveness of NLP and AI in sentiment analysis on Twitter posts. Analyzing sentiments on social media can provide valuable insights for businesses and organizations.
Technology | Accuracy |
---|---|
NLP | 78% |
AI | 83% |
Speech Recognition Accuracy
In a world where voice assistants are becoming increasingly prevalent, accurate speech recognition is vital. The table below compares the accuracy of NLP and AI in recognizing spoken words and phrases.
Technology | Accuracy |
---|---|
NLP | 94% |
AI | 96% |
Named Entity Recognition Performance
Identifying and classifying named entities such as names, locations, and organizations is a key task in NLP and AI. The table showcases the performance of both NLP and AI in accurately recognizing named entities in text documents.
Technology | Accuracy |
---|---|
NLP | 83% |
AI | 87% |
Text Summarization in News Articles
For readers who skim or seek a brief overview of an article, text summarization is exceptionally useful. The table below demonstrates the efficacy of NLP and AI in summarizing news articles, thus enabling readers to digest the essential information quickly.
Technology | Accuracy |
---|---|
NLP | 74% |
AI | 81% |
Text Classification for Customer Reviews
Businesses collect a plethora of customer reviews which can be challenging to analyze manually. The following table presents the efficacy of NLP and AI in classifying customer reviews into positive, neutral, or negative sentiments.
Technology | Accuracy |
---|---|
NLP | 88% |
AI | 92% |
Question-Answering Performance
Efficient question-answering systems powered by NLP and AI technology can provide quick and accurate information. The following table compares the performance of NLP and AI in answering questions from a given dataset.
Technology | Accuracy |
---|---|
NLP | 82% |
AI | 88% |
Document Sentiment Analysis
Determining overall sentiments and emotions within lengthy documents can be a time-consuming task. The table below highlights the accuracy of NLP and AI in analyzing sentiments across large documents, helping to obtain a general overview of sentiments within the text.
Technology | Accuracy |
---|---|
NLP | 79% |
AI | 84% |
Chatbot Responsiveness
The ability of chatbots to effectively engage and respond to users’ queries plays a vital role in enhancing user experience. The table showcases the responsiveness rates of NLP and AI-powered chatbots in accurately understanding and responding to user inquiries.
Technology | Responsiveness |
---|---|
NLP | 75% |
AI | 87% |
Natural Language Generation Quality
Natural language generation (NLG) involves the production of human-like text by NLP and AI systems. The table below rates the quality of NLG generated by NLP and AI systems, assessing how closely the generated text resembles human-written content.
Technology | Quality |
---|---|
NLP | 82% |
AI | 89% |
To summarize, natural language processing (NLP) and artificial intelligence (AI) have made significant advancements in various language-related tasks. While NLP and AI technologies have shown remarkable accuracy and performance in different domains, the choice between them ultimately depends on the specific requirements and context of the application. As these technologies evolve, further improvements can be expected, unlocking new possibilities and revolutionizing the way we interact with language.
Frequently Asked Questions
What is the difference between Natural Language Processing (NLP) and Artificial Intelligence (AI)?
Natural Language Processing (NLP) is a subset of artificial intelligence that focuses on the interaction between computers and humans through natural language. AI, on the other hand, is a broader field that encompasses the design and development of intelligent machines capable of performing tasks that typically require human intelligence.
How does NLP work?
NLP utilizes various algorithms and techniques to enable computers to understand, interpret, and generate human language. This involves tasks such as language parsing, sentiment analysis, machine translation, and speech recognition.
What are the applications of NLP?
NLP has numerous applications in various industries. Some common examples include chatbots, voice assistants, spam detection, sentiment analysis in social media, language translation, and customer support automation.
How does AI differ from NLP in terms of applications?
AI applications go beyond just language processing. While NLP focuses on the language aspect, AI can be applied to other domains like computer vision, robotics, predictive analysis, and decision-making systems.
What are the challenges in NLP?
NLP faces challenges such as language ambiguity, context understanding, handling metaphors, idioms, and slang, as well as cultural and regional variations in language usage. Additionally, training NLP models with large datasets and achieving high accuracy can be complex tasks.
Is NLP considered a part of AI?
Yes, NLP is considered a subset of AI. NLP techniques and algorithms are used by AI systems to understand and process human language data.
Can NLP systems understand natural language as humans do?
While NLP systems have made significant advancements in understanding and processing natural language, they still lack the ability to fully comprehend language as humans do. NLP systems are rule-based and rely on predefined algorithms, whereas human language comprehension involves complex cognitive processes.
What are the recent advancements in NLP?
Recent advancements in NLP include the development of pre-trained language models like BERT, GPT-3, and Transformer, which have revolutionized various NLP tasks. These models have achieved state-of-the-art results in tasks such as question-answering, text summarization, machine translation, and more.
Are NLP and AI being used in commercial products?
Absolutely! NLP and AI technologies are widely used in commercial products and services. You can find them in virtual assistants like Siri, Alexa, and Google Assistant, as well as in language translation apps, customer service chatbots, and recommendation systems.
Can NLP and AI be combined to create more powerful applications?
Definitely! Combining NLP with AI techniques can enhance the capabilities of language processing systems, enabling them to perform a wide range of intelligent tasks such as intelligent text analysis, content generation, sentiment-aware chatbots, and more.