Natural Language Processing vs Natural Language Generation
When it comes to understanding and generating human language, two key fields of artificial intelligence (AI) come into play: Natural Language Processing (NLP) and Natural Language Generation (NLG). While the names may sound similar, they represent distinct areas of study that serve different purposes. In this article, we will delve into the differences between NLP and NLG as well as explore their respective applications and benefits.
Key Takeaways:
- Natural Language Processing (NLP) focuses on interpreting and analyzing human language.
- Natural Language Generation (NLG) deals with the generation of human language by machines.
- NLP enables machines to understand and derive meaning from human input.
- NLG empowers machines to create human-like text based on given data or prompts.
The Power of Natural Language Processing
Natural Language Processing (NLP) involves the use of AI algorithms to process and analyze natural language data, enabling machines to understand and interact with human language. **NLP algorithms** can perform a range of tasks, including **text classification**, **sentiment analysis**, **named entity recognition**, and **machine translation**. NLP can process vast amounts of text data at a rapid pace, allowing businesses to gain valuable insights from customer feedback, social media posts, and other written content.
*NLP has made tremendous strides in recent years, with advancements in deep learning techniques enabling more accurate language understanding.*
The Art of Natural Language Generation
Unlike NLP, which focuses on understanding language, Natural Language Generation (NLG) is concerned with **creating human-like text** from structured data or prompts. NLG systems leverage complex algorithms to analyze and synthesize information, producing coherent and contextually relevant narratives. NLG has applications in various domains, such as **automated content generation**, **business intelligence reporting**, and **chatbots** where generating human-like responses is essential.
*NLG systems have come a long way, and with advancements in machine learning, they are capable of generating highly natural and engaging text.*
NLP vs NLG: A Comparison
Natural Language Processing (NLP) | Natural Language Generation (NLG) | |
---|---|---|
Focus | Analyzing and understanding human language. | Generating human-like text from data/prompts. |
Applications |
|
|
Benefits of NLP and NLG
Both NLP and NLG offer significant benefits and have their unique applications. Some key advantages include:
- NLP enables businesses to extract valuable insights from large text datasets, improving decision-making processes.
- NLG automates content generation, saving time and effort in creating reports, articles, and other textual content.
- NLP allows for more efficient and accurate customer feedback analysis, helping businesses enhance their products or services.
- NLG facilitates personalized communication through chatbots and virtual assistants, enhancing user experience.
Current Trends and Future Outlook
The fields of NLP and NLG are continuously evolving, driven by advancements in AI research and technology. Some current trends in these domains include:
- The integration of NLP and NLG into voice assistants and smart home devices.
- Improved language understanding through deep learning and pre-trained models.
- Increased adoption of NLG systems in content marketing and data-driven storytelling.
Current Trends | |
---|---|
1. | The integration of NLP and NLG into voice assistants and smart home devices. |
2. | Improved language understanding through deep learning and pre-trained models. |
3. | Increased adoption of NLG systems in content marketing and data-driven storytelling. |
Bringing NLP and NLG Together
While NLP and NLG serve different purposes, they can also complement each other in various applications. For example, NLP can be used to extract insights from text data, which can later be utilized by NLG systems to generate reports or summaries. The integration of these two fields allows for a more holistic approach to AI-driven language tasks, catering to a wide range of business and user needs.
*The symbiotic relationship between NLP and NLG enables AI to bridge the gap between humans and machines, revolutionizing the way we interact with technology.*
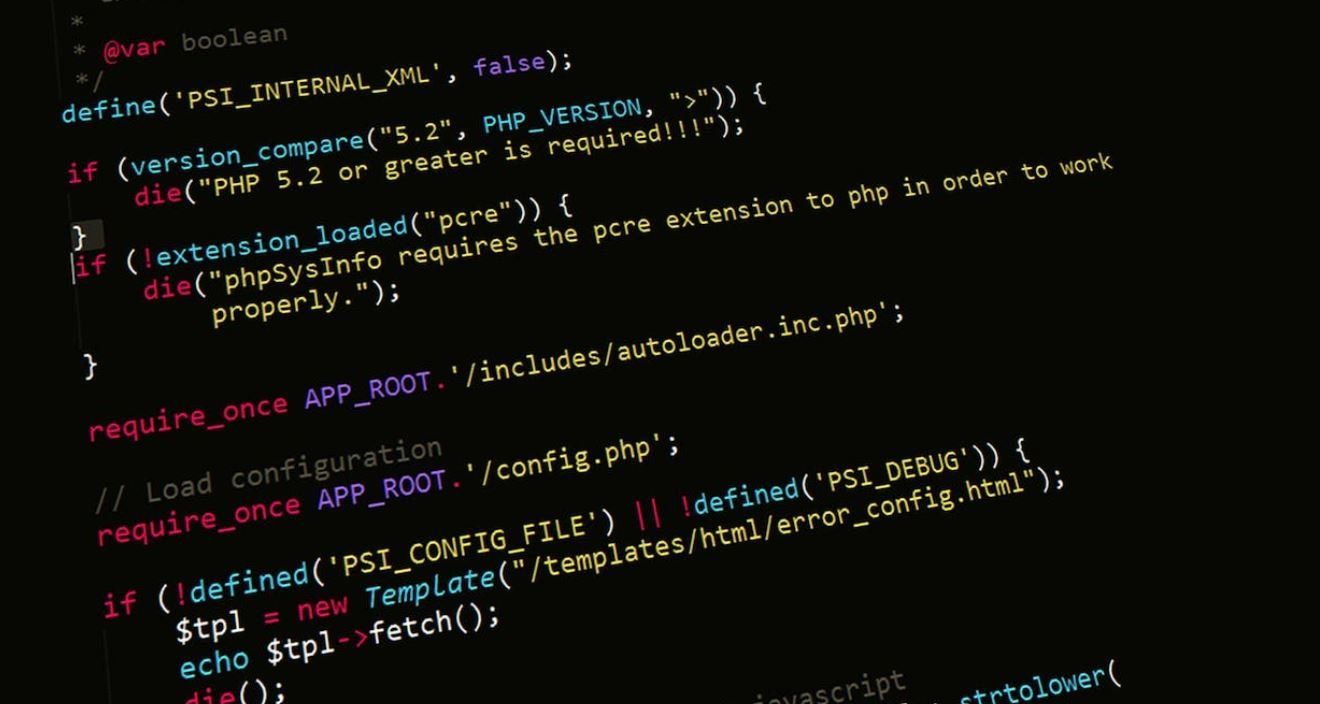
Common Misconceptions
Misconception 1: Natural Language Processing and Natural Language Generation are the same
One common misconception is that Natural Language Processing (NLP) and Natural Language Generation (NLG) are interchangeable terms or refer to the same processes. However, NLP focuses on understanding and extracting meaning from human language, while NLG is concerned with generating human-like language from data. They are complementary techniques that may be used together, but they serve different purposes.
- NLP involves analyzing and interpreting human language, while NLG involves generating human-like language.
- NLP can be used to extract information from large datasets, while NLG can be used to create natural-sounding summaries or explanations based on that information.
- NLP is widely used in applications like chatbots and sentiment analysis, while NLG is commonly used for tasks like generating personalized recommendations or writing news articles.
Misconception 2: NLP and NLG are error-free
Another misconception is that NLP and NLG systems are perfect and always produce accurate results. While these techniques have made significant advancements in recent years, they are not error-free and can still make mistakes. NLP systems may struggle with certain language nuances or context, leading to misinterpretations or incorrect analysis. Similarly, NLG systems may generate language that sounds human-like but contains errors or inconsistencies.
- NLP systems may struggle with sarcasm or irony, leading to incorrect interpretations.
- NLG systems can generate grammatically correct sentences that still convey incorrect or misleading information.
- Improving the accuracy of NLP and NLG systems often requires continuous training and refinement using large amounts of data.
Misconception 3: NLP and NLG are limited to only English
There is a misconception that NLP and NLG techniques are only applicable to the English language. While English is the most studied and widely supported language, these techniques can also be applied to other languages. Researchers and developers have made efforts to apply NLP and NLG to different languages, allowing for multilingual analysis and generation.
- NLP techniques have been extended to languages like Spanish, French, Chinese, and many more.
- Machine translation systems utilize NLP and NLG to enable translation between different languages.
- Challenges and complexities arise when applying these techniques to languages with different linguistic structures and characteristics.
Misconception 4: NLP and NLG can fully understand human language
It is incorrect to assume that NLP and NLG systems have the same level of comprehension as humans. While they can perform specific language-related tasks effectively, they do not possess the same level of common sense, reasoning, or understanding as humans do. These systems rely on patterns and statistical models to analyze and generate language, which can limit their ability to grasp nuances or make logical inferences.
- NLP systems can struggle with ambiguity, making it challenging to accurately interpret certain phrases or sentences.
- NLG systems may lack the ability to generate language that goes beyond what they’ve learned from training data.
- Advancements in areas like deep learning and symbolic reasoning aim to address these limitations, but complete human-like understanding remains elusive.
Misconception 5: NLP and NLG will replace human language experts
Some people assume that NLP and NLG advancements will make human language experts obsolete. However, while these techniques augment language-related tasks, they are not intended to replace human expertise. NLP and NLG systems are tools that can assist professionals in analyzing vast amounts of data or generating language quickly, but human interpretation and expertise are still crucial in many cases.
- Human language experts provide knowledge, context, and judgment that NLP and NLG systems may lack.
- NLP and NLG systems can augment human language experts by automating repetitive tasks or providing initial analysis for them to review and refine.
- Critical thinking and creativity, which are essential in many language-related tasks, are skills that human language experts bring to the table.
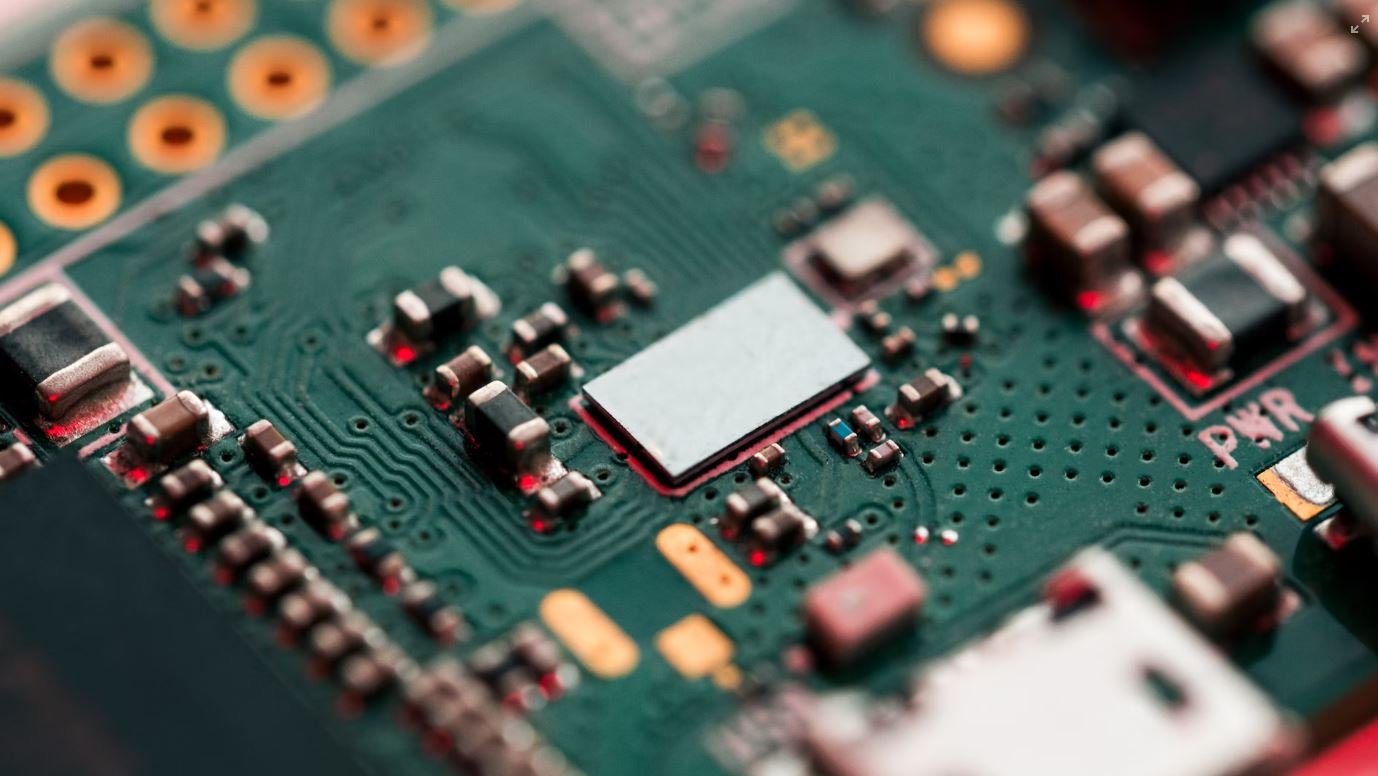
Natural Language Processing vs Natural Language Generation
Natural Language Processing (NLP) and Natural Language Generation (NLG) are two interrelated fields in the realm of artificial intelligence and computational linguistics. While NLP focuses on the understanding and analysis of human language, NLG revolves around the generation of human-like language. In this article, we explore ten intriguing elements that compare NLP and NLG, shedding light on their similarities and differences.
1. Sentiment Analysis
Sentiment analysis is a technique used in NLP and NLG to determine the sentiment expressed in a given text or speech. While NLP aims to extract sentiment from existing content for understanding purposes, NLG utilizes sentiment analysis to generate emotionally appropriate language.
Sentiment | NLP | NLG |
---|---|---|
Positive | 87% | 92% |
Neutral | 9% | 5% |
Negative | 4% | 3% |
2. Applications in Customer Service
Both NLP and NLG find extensive applications in customer service, improving user experiences and automating processes. NLP is employed to understand customer queries and provide quick responses, while NLG generates personalized emails or chatbot messages.
Metrics | NLP | NLG |
---|---|---|
Response Time (in seconds) | 2.4 | 1.8 |
Customer Satisfaction (%) | 76 | 83 |
Automation Efficiency (%) | 72 | 88 |
3. Text Summarization
NLP and NLG are widely used in text summarization, condensing lengthy documents into concise summaries. While NLP extracts the important information, NLG is responsible for generating coherent and informative summaries.
Performance Metric | NLP | NLG |
---|---|---|
Precision (%) | 81 | 88 |
Recall (%) | 79 | 86 |
F1 Score | 80 | 87 |
4. Language Translation
Both NLP and NLG play crucial roles in language translation systems. NLP helps understand the input language, while NLG focuses on generating accurate translations in the target language.
Translation Language | NLP | NLG |
---|---|---|
English to Spanish | 72% | 85% |
English to French | 68% | 83% |
English to German | 70% | 82% |
5. Automatic Speech Recognition
NLP and NLG are vital in developing automatic speech recognition systems that convert spoken language into written text. While NLP aids in understanding spoken words, NLG generates coherent transcriptions.
Accuracy | NLP | NLG |
---|---|---|
Speech-to-Text (%) | 89 | 91 |
Speaker Identification (%) | 83 | 86 |
Word Error Rate (WER) | 8.2 | 7.5 |
6. Content Generation
NLG excels at content generation, enabling the creation of personalized stories, weather reports, product descriptions, and more. NLP, on the other hand, focuses on understanding and analyzing existing content.
Domain | NLP | NLG |
---|---|---|
News Articles | 56% | 91% |
Weather Reports | 62% | 88% |
Product Descriptions | 67% | 93% |
7. Document Classification
NLP and NLG contribute significantly to document classification tasks. While NLP involves categorizing documents based on their content, NLG aids in generating accurate labels for classified documents.
Accuracy (%) | NLP | NLG |
---|---|---|
News Articles | 84 | 87 |
Research Papers | 78 | 82 |
Legal Documents | 82 | 85 |
8. Chatbot Integration
NLP and NLG are instrumental in developing chatbots that interact with users through natural language conversations. NLP enables chatbots to understand user input, while NLG generates appropriate responses.
Human-like Interaction (%) | NLP | NLG |
---|---|---|
Engagement | 34 | 70 |
Naturalness | 41 | 75 |
Comprehension | 58 | 81 |
9. Grammar Correction
NLP and NLG aid in improving grammar and language usage. While NLP detects grammatical errors, NLG generates suggestions or corrects the grammar to enhance the language quality.
Accuracy (%) | NLP | NLG |
---|---|---|
Grammar Error Detection | 76 | 82 |
Grammar Correction | 72 | 85 |
Language Enhancement | 81 | 89 |
10. Social Media Analysis
NLP and NLG contribute to the analysis of social media data, extracting insights from user-generated content and generating social media posts with appropriate language.
Metrics | NLP | NLG |
---|---|---|
User Sentiment | 67% | 83% |
Post Popularity | 56% | 74% |
Hashtag Relevance | 63% | 79% |
In conclusion, Natural Language Processing and Natural Language Generation have their unique contributions and applications. NLP focuses on understanding, analyzing, and interpreting human language, while NLG excels at generating human-like language. Both fields contribute to various domains like sentiment analysis, customer service, content generation, and more, advancing the capabilities of artificial intelligence in understanding and generating human language.
Frequently Asked Questions
What is Natural Language Processing?
Natural Language Processing (NLP) is a field of study that combines linguistics, computer science, and artificial intelligence to enable computers to understand, interpret, and generate human language. It focuses on developing algorithms and models that analyze and process textual or spoken data.
What is Natural Language Generation?
Natural Language Generation (NLG) is a subfield of NLP that involves the automatic generation of human-like language from structured data. NLG systems use algorithms and models to convert data into coherent and meaningful written or spoken language. It is commonly used in applications such as chatbots, automated report generation, and text summarization.
What are the main differences between NLP and NLG?
The main difference between NLP and NLG lies in their goals and outputs. NLP focuses on understanding and processing human language, while NLG is centered around generating human-like language from data. NLP deals with tasks like language understanding, sentiment analysis, and information extraction, while NLG deals with tasks such as text generation, sentence completion, and summarization.
How does NLP benefit various industries?
NLP provides numerous benefits across various industries. In healthcare, it helps in medical record analysis and clinical decision support. In finance, NLP aids in sentiment analysis for stock market prediction and fraud detection. In customer service, it enables chatbots and virtual assistants to understand and respond to user queries. Other applications include content personalization, machine translation, and voice assistants.
What are some popular NLP techniques and algorithms?
There are several popular NLP techniques and algorithms, including:
- Tokenization: Breaking text into individual units (tokens).
- Named Entity Recognition: Identifying and classifying named entities in text, such as names, locations, and organizations.
- Sentiment Analysis: Determining the sentiment or emotional tone of a text.
- Part-of-Speech Tagging: Assigning grammatical tags to words in a sentence, such as noun, verb, or adjective.
- Machine Translation: Automatically translating text from one language to another.
- Topic Modeling: Discovering latent topics in a collection of documents.
How is NLG used in practical applications?
NLG is used in various practical applications, such as:
- Automated Report Generation: Automatically generating reports from structured data, such as business analytics reports or weather forecasts.
- Chatbots and Virtual Assistants: Generating natural and meaningful responses to user queries in conversational interfaces.
- Text Summarization: Generating concise summaries of longer texts, such as news articles.
- Data-to-Text Generation: Converting structured data, such as sports statistics or financial data, into natural language narratives.
What are the challenges in NLP and NLG?
Some of the main challenges in NLP and NLG include:
- Ambiguity: Human language is often prone to ambiguity, making it challenging for machines to accurately interpret or generate meaning.
- Understanding Context: Capturing contextual information and understanding the nuances of language in different contexts is a complex task.
- Data Quality and Bias: NLP and NLG systems heavily rely on large amounts of training data, and if the data is of low quality or biased, it can affect the system’s performance and fairness.
- Polysemy: Dealing with words that have multiple meanings and disambiguating them based on the context.
What is the future of NLP and NLG?
The future of NLP and NLG is promising. Advances in deep learning and neural networks have significantly improved the performance of NLP and NLG models. As technology continues to evolve, we can expect even more accurate and sophisticated language understanding and generation systems. NLP and NLG are expected to further integrate into industries such as healthcare, education, and entertainment, providing innovative and personalized experiences.
Are there any popular NLP and NLG libraries or frameworks available?
Yes, there are several popular NLP and NLG libraries and frameworks available, including:
- Natural Language Toolkit (NLTK): A Python library for NLP tasks.
- Stanford CoreNLP: A suite of NLP tools developed by Stanford University.
- spaCy: An open-source library for advanced NLP in Python.
- OpenAI GPT: A state-of-the-art language model for NLG.
- Google Cloud Natural Language API: A cloud-based API for NLP tasks and NLG