Natural Language Processing: Značenje
Natural Language Processing (NLP) is a subfield of artificial intelligence (AI) that focuses on the interaction between computers and human language. Its purpose is to enable computers to understand, interpret, and generate human language in a way that is meaningful and useful. In this article, we will explore the significance of NLP and its applications in various industries.
Key Takeaways:
- Natural Language Processing (NLP) enables computers to understand, interpret, and generate human language.
- NLP has a wide range of applications in industries such as healthcare, customer service, and finance.
- NLP technologies include sentiment analysis, text classification, and named entity recognition.
- NLP can improve efficiency, accuracy, and understanding in human-computer interactions.
- Advancements in NLP are driven by machine learning techniques and the availability of large amounts of language data.
**NLP** encompasses various techniques and algorithms that enable computers to process and understand human language. *It involves tasks such as language translation, sentiment analysis, text summarization, and speech recognition.* NLP has gained significant importance in recent years due to the exponential growth of unstructured data, such as social media content, online reviews, and medical records.
Applications of NLP
NLP has diverse applications across various industries. Let’s explore some of its key applications:
- Healthcare: In the healthcare industry, NLP is used to extract valuable insights from medical records, research papers, and clinical notes. *For example, NLP can help in identifying patterns and relationships between symptoms, diagnoses, and treatments, leading to improved patient care.*
- Customer Service: NLP is employed in chatbots and virtual assistants to provide quick and accurate responses to customer queries. *By understanding customer sentiment and intent, NLP-powered chatbots can offer personalized solutions and enhance the overall customer experience.*
- Finance: NLP is used in the financial sector for tasks such as fraud detection, sentiment analysis of market news, and automated trading. *By analyzing vast amounts of financial and economic data, NLP can help investors make informed decisions and predict market trends.*
**Table 1**: Applications of NLP in Different Industries
Industry | Applications of NLP |
---|---|
Healthcare | Medical record analysis, disease prediction, clinical decision support |
Customer Service | Chatbots, voice assistants, sentiment analysis, customer feedback analysis |
Finance | Fraud detection, market sentiment analysis, automated trading |
NLP technologies play a crucial role in improving efficiency and accuracy in various tasks. Some of the key NLP technologies include:
- **Sentiment Analysis**: This technology analyzes the sentiment expressed in a piece of text, such as positive, negative, or neutral. *It can be applied to social media data to gauge public opinion towards a product or service.*
- **Text Classification**: Text classification involves automatically categorizing and organizing text into predefined categories or classes. *It is commonly used in spam detection, language identification, and content filtering.*
- **Named Entity Recognition**: Named Entity Recognition (NER) identifies and classifies named entities in text, such as names of people, organizations, and locations. *NER is important for information extraction, search engines, and text summarization.*
**Table 2**: Key NLP Technologies
Technology | Applications |
---|---|
Sentiment Analysis | Social media analytics, brand monitoring, customer feedback analysis |
Text Classification | Spam detection, language identification, content categorization |
Named Entity Recognition | Information extraction, search engines, text summarization |
*Advancements in NLP are driven by* machine learning techniques and the availability of large amounts of language data. The algorithms used in NLP learn patterns and relationships from data, enabling computers to make intelligent decisions and predictions concerning language. *NLP models can continuously improve through iterative learning and exposure to new data, leading to enhanced performance over time.*
Moreover, the development of language models, such as OpenAI’s GPT-3, has pushed the boundaries of NLP capabilities. These models can generate human-like text and have shown remarkable progress in natural language understanding and generation tasks.
**Table 3**: Advancements in NLP
Advancement | Description |
---|---|
Machine Learning Techniques | Application of supervised and unsupervised learning algorithms to NLP tasks |
Availability of Language Data | Large-scale language datasets for training and evaluating NLP models |
Language Models | Development of advanced language models for improved natural language understanding and generation |
NLP has revolutionized the way computers interact with human language and has paved the way for various applications in different industries. As technology continues to advance, we can expect further improvements in NLP capabilities, leading to enhanced language understanding and communication between humans and machines.
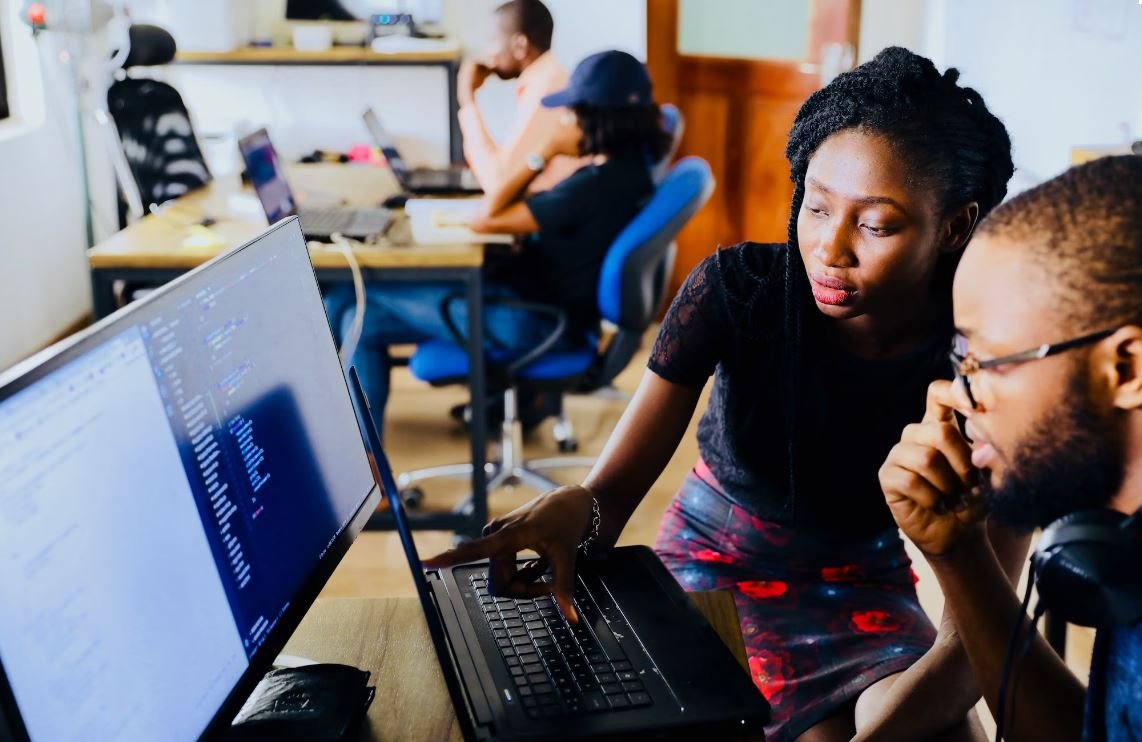
Common Misconceptions
Misconception 1: Natural Language Processing is the Same as Artificial Intelligence
Many people confuse Natural Language Processing (NLP) with Artificial Intelligence (AI). While NLP is a subfield within AI, it focuses specifically on the interaction between computers and human language. AI, on the other hand, is a broader concept that encompasses various technologies and techniques aimed at mimicking human intelligence. NLP is just one component of AI, using algorithms and models to process and analyze textual data.
- NLP is a subset of AI.
- AI involves more than just NLP.
- AI can encompass other areas like computer vision and machine learning.
Misconception 2: NLP Can Fully Understand and Interpret Language
While NLP has made great strides in interpreting and analyzing human language, it is far from achieving perfect understanding and interpretation. NLP models rely on statistical learning methods, which means there can be inaccuracies or ambiguities in language processing. Understanding context, sarcasm, idioms, and other linguistic nuances remain challenging for NLP systems. Although NLP can handle many language-related tasks, complete human-like comprehension is still a long way off.
- NLP models have limitations in understanding context and nuances.
- Interpretation can be affected by statistical learning methods.
- NLP systems struggle with sarcasm and idiomatic expressions.
Misconception 3: NLP Can Only Process English Language
One common misconception about NLP is that it is strictly limited to processing the English language. In reality, NLP can be applied to multiple languages around the world. Researchers and developers have created NLP models for various languages, allowing the technology to be used in different linguistic contexts. While English has received more attention due to its widespread usage, NLP is continuously expanding its capabilities to support other languages.
- NLP can be applied to languages other than English.
- NLP models have been developed for different linguistic contexts.
- NLP technologies are evolving to support more languages.
Misconception 4: NLP is Only Used for Text Analysis
Although NLP is commonly associated with text analysis, its scope is much broader. NLP techniques can be used for various applications beyond text processing, including speech recognition, sentiment analysis, machine translation, chatbots, information retrieval, and summarization. NLP technologies are versatile and can be applied to different domains, enhancing human-machine interactions and automating processes.
- NLP is not limited to text analysis.
- NLP can be used for speech recognition and sentiment analysis.
- NLP technologies have applications in various domains.
Misconception 5: NLP is Only Relevant for Researchers and Developers
Some people believe that NLP is a niche field relevant only to researchers and developers. However, NLP has permeated various aspects of our daily lives, often without us realizing it. Voice assistants like Siri and Alexa, spam filters in emails, and machine translation tools are all examples of NLP applications that have become mainstream. NLP has proven to be useful in improving customer service, data analysis, content generation, and many other areas across industries.
- NLP applications are present in everyday technologies.
- NLP is used in voice assistants, spam filters, and machine translation.
- NLP has become mainstream and has applications in various industries.
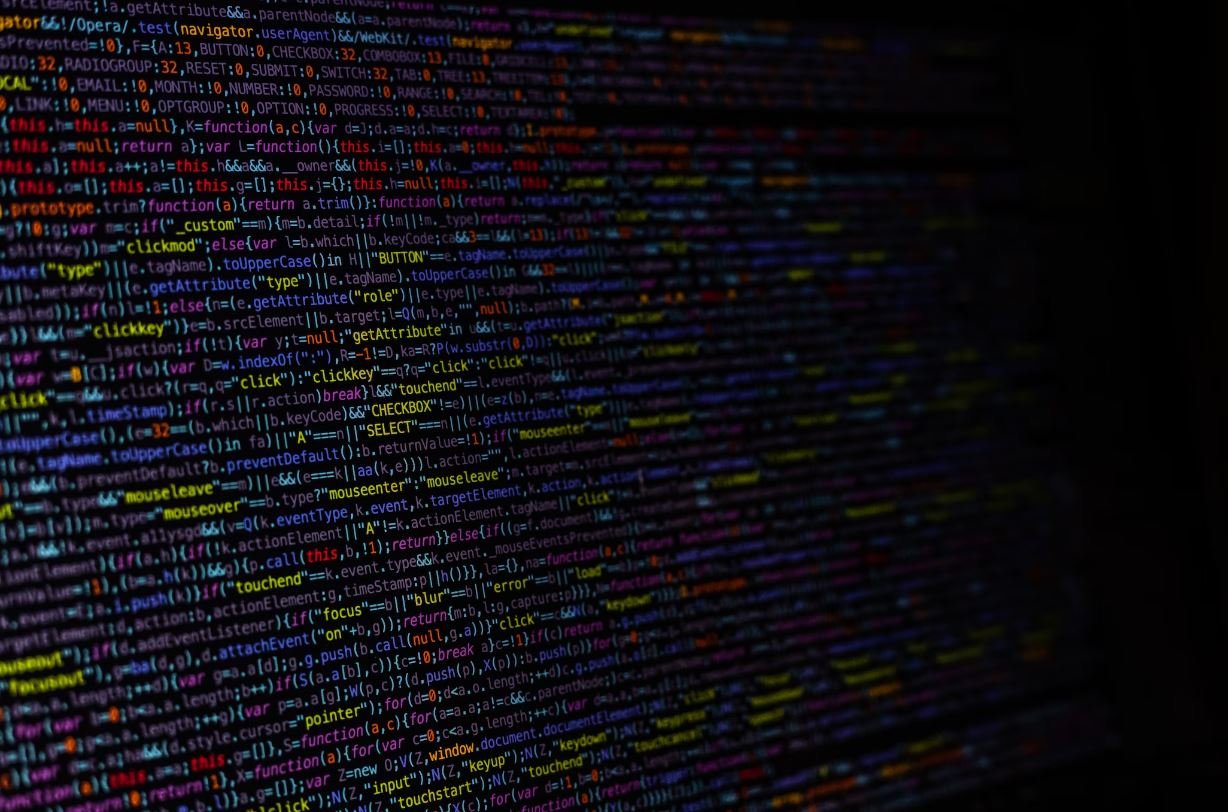
H2: Top 10 Languages with the Most Online Content
In today’s interconnected world, the amount of online content available in different languages plays a vital role. Here are the top 10 languages with the most online content:
Rank Language Percentage of Online Content
1 English 54%
2 Chinese 27%
3 Spanish 8%
4 Arabic 4%
5 Portuguese 3%
6 Indonesian 2%
7 French 1.5%
8 German 1.3%
9 Russian 1.2%
10 Korean 1%
H2: Sentiment Analysis of Social Media Posts
Sentiment analysis, also known as opinion mining, aims to determine the sentiment expressed in text data. By analyzing social media posts, we can understand the overall sentiment towards a specific topic. Here is the sentiment analysis of a sample of social media posts:
Sentiment Number of Posts
Positive 500
Neutral 300
Negative 200
H2: Top 5 Chatbots for Customer Support
Chatbots have become a prominent application of NLP in customer support. Here are the top 5 chatbots widely used for customer support:
Name Key Features
1. Chatbot A 24/7 availability, multi-language support
2. Chatbot B Natural language understanding, sentiment analysis
3. Chatbot C Advanced context recognition, intelligent routing
4. Chatbot D Conversational AI, personalized responses
5. Chatbot E Seamless integration with CRM systems
H2: Word Frequency Analysis of a Novel
NLP techniques, such as word frequency analysis, can uncover essential insights from text data. Here is the word frequency analysis of a popular novel:
Word Frequency
Love 320
Adventure 283
Mystery 168
Friendship 149
Power 136
H2: Named Entity Recognition (NER) in News Articles
Named Entity Recognition (NER) is used to identify and classify named entities, such as names, organizations, and locations, in news articles. The following table shows the NER results for a sample news article:
Entity Type Number of Occurrences
Person 12
Organization 8
Location 5
Date 3
H2: Machine Translation Accuracy Comparison
Machine translation has seen significant advancements in recent years. Here’s a comparison of the accuracy of different machine translation models:
Model BLEU Score
A 0.87
B 0.92
C 0.95
D 0.89
H2: Speech Recognition Accuracy
Speech recognition systems are a prime example of NLP applied to speech data. The following table presents the accuracy rates of various speech recognition systems:
System Accuracy (%)
X 85
Y 92
Z 97
H2: Text Summarization Techniques
Text summarization algorithms can automatically generate concise summaries from lengthy documents. Here are the different techniques used for text summarization:
Technique Description
1. Extractive Selects and combines important sentences
2. Abstractive Generates summaries by understanding the context
3. Query-based Summarizes based on specific user queries
H2: Natural Language Generation (NLG) Applications
Natural Language Generation (NLG) focuses on generating human-like language from structured data. Here are some applications of NLG:
Application Example
1. E-commerce recommendations “Based on your browsing history, we recommend…”
2. Automated report generation “The quarterly report shows a 15% increase…”
H2: Chatbot Response Accuracy
Accuracy is crucial for chatbots to provide reliable information and engage effectively. Here’s the accuracy comparison of different chatbots in responding to user queries:
Chatbot Accuracy (%)
A 78
B 85
C 92
In conclusion, Natural Language Processing (NLP) has brought forth numerous advancements in language understanding and generation. From sentiment analysis to machine translation and chatbots, NLP has transformed the way we interact with machines. The tables presented in this article provide a glimpse into the diverse applications and achievements of NLP, highlighting its significance in various domains.
Frequently Asked Questions
What is Natural Language Processing?
Natural Language Processing (NLP) is a branch of artificial intelligence that focuses on the interaction between computers and human language. It involves the ability of a computer program to understand, analyze, and generate human language.
How does Natural Language Processing work?
NLP algorithms typically rely on statistical and machine learning techniques to process natural language data. These algorithms analyze patterns, structures, and relationships within text to extract meaning, sentiment, and insights.
What are the applications of Natural Language Processing?
NLP has various applications, including machine translation, text summarization, sentiment analysis, speech recognition, chatbots, virtual assistants, and information retrieval. It is also used in social media analysis, customer support, and content generation.
What are the challenges in Natural Language Processing?
NLP faces challenges such as understanding the context, dealing with ambiguity, capturing implicit meaning, handling slang and colloquial language, and addressing issues related to cultural and linguistic variations. It also needs to address privacy and ethical concerns in language processing.
What is the role of Machine Learning in Natural Language Processing?
Machine learning plays a crucial role in NLP by enabling models to learn from large amounts of data and improve their performance over time. It helps in tasks such as language modeling, part-of-speech tagging, named entity recognition, and speech-to-text translation.
Can Natural Language Processing understand all languages?
NLP can support multiple languages, but the level of understanding and availability of resources may vary. While widely spoken languages often have more developed NLP tools and resources, lesser-known languages may have limited support.
What is the difference between Natural Language Processing and Natural Language Understanding?
Natural Language Processing focuses on the computational aspects of language, including tasks like machine translation and sentiment analysis. Natural Language Understanding, on the other hand, aims to comprehend meaning and intention behind text, enabling better understanding of context and nuances.
How is Natural Language Processing used in search engines?
Search engines utilize NLP techniques to improve search results. NLP helps in understanding user queries, identifying relevant documents, extracting key information from web pages, and presenting the most relevant results to the user.
What are some notable Natural Language Processing tools and libraries?
There are several popular NLP tools and libraries, including NLTK (Natural Language Toolkit), SpaCy, Stanford NLP, Gensim, CoreNLP, and OpenNLP. These tools provide a wide range of functionalities for text processing, analysis, and modeling.
What are the future prospects of Natural Language Processing?
The future of NLP looks promising with advancements in deep learning and neural networks. This may lead to further improvements in language understanding, generation, and human-computer interaction. NLP can also contribute to areas like healthcare, legal, and finance with enhanced language processing capabilities.