Natural Language Understanding Generation
In the era of artificial intelligence and machine learning, computers are becoming more adept at understanding and generating human language. Natural Language Understanding (NLU) and Natural Language Generation (NLG) are two branches of AI that focus on interpreting and generating human language, respectively. NLU aims to enable computers to comprehend and interpret human language, while NLG focuses on creating coherent and meaningful text.
Key Takeaways:
- Natural Language Understanding (NLU) and Natural Language Generation (NLG) are two important branches of AI.
- NLU focuses on interpreting and comprehending human language, while NLG is about generating human-like text.
- Artificial intelligence and machine learning have significantly advanced the capabilities of NLU and NLG.
**NLU** involves the use of algorithms and techniques to extract meaning and context from text, enabling computers to understand and respond to human input. By analyzing the structure and patterns within sentences, **NLU** algorithms can accurately interpret the intended meaning behind a piece of text. *For example, NLU can help chatbots understand and respond appropriately to user queries.*
**NLG**, on the other hand, focuses on the process of generating meaningful and coherent text. NLG systems use algorithms to analyze data and create human-like output in the form of written or spoken language. *This technology has been instrumental in chatbots, content generation, and language translation.*
The Advancement of NLU and NLG with AI
With the rapid advancement of artificial intelligence and machine learning, the capabilities of NLU and NLG have significantly improved. AI algorithms are now able to recognize complex linguistic structures, understand context, and generate text that is almost indistinguishable from human-authored content. This progression has opened up numerous possibilities in various industries:
- Customer Service: Chatbots equipped with advanced **NLU** can provide instant and accurate responses to customer inquiries.
- Personal Assistants: NLU technology has enhanced personal assistants like Siri and Alexa to better understand user commands and provide relevant information.
- Data Analysis: NLG systems can automatically generate reports and summaries from large datasets, making data analysis more efficient.
Applications and Use Cases
From healthcare to finance, NLU and NLG have found applications across various domains:
Table 1: Applications of NLU and NLG
Industry | NLU Applications | NLG Applications |
---|---|---|
Healthcare | Medical diagnosis, patient support systems | Generating patient reports, medical advice |
Finance | Financial analysis, fraud detection | Automated financial reporting, investment advice |
E-commerce | Product recommendations, customer support | Generating product descriptions, personalized offers |
As the table above demonstrates, NLU and NLG play a crucial role in transforming industries by automating tasks and improving overall efficiency.
Challenges and Future Directions
- **Lack of Contextual Understanding**: While NLU systems have made significant progress, they still face challenges in understanding and responding to nuanced language and context.
- **Ethical Considerations**: With NLG systems becoming better at generating text, ethical concerns regarding the dissemination of misinformation and fake news have emerged.
- **Multilingual Support**: NLU and NLG systems must continue to advance to ensure accurate interpretation and generation of language across different languages and cultural contexts.
Conclusion
The field of Natural Language Understanding (NLU) and Natural Language Generation (NLG) has seen remarkable advancements through AI. NLU enables computers to comprehend and respond to human language, while NLG makes text generation more human-like. As technology continues to advance, we can expect further improvements in NLU and NLG, leading to a multitude of applications and enhanced user experiences in various domains.
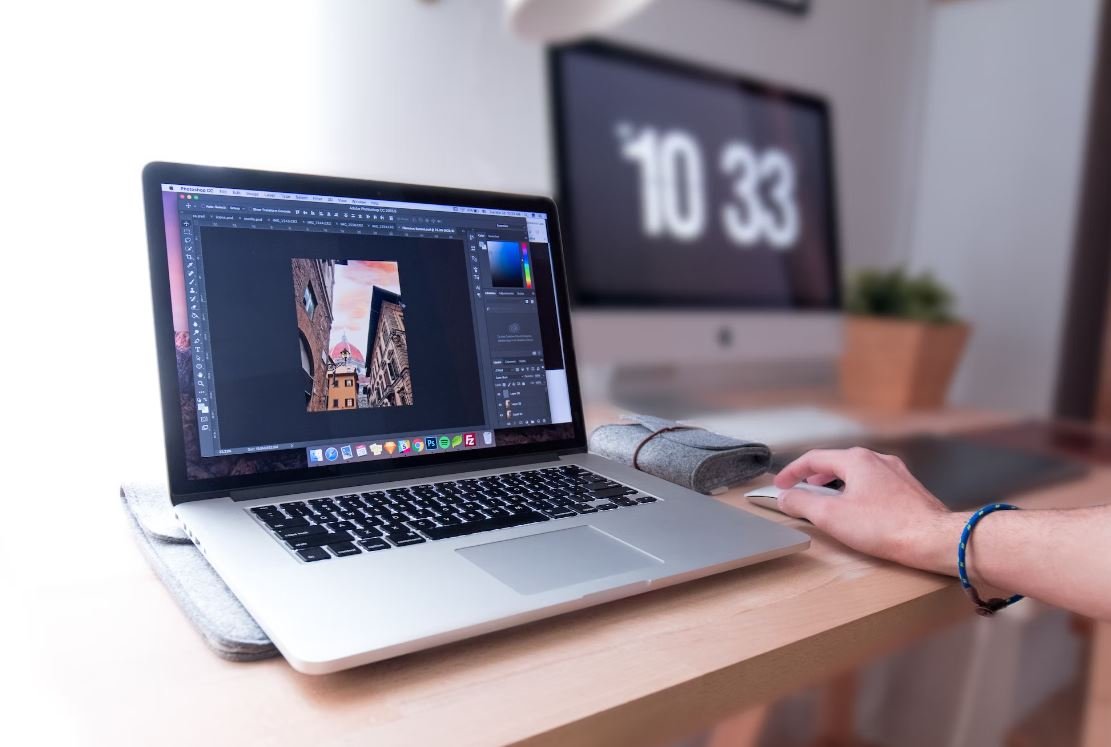
Common Misconceptions
1. Natural Language Understanding
One common misconception about natural language understanding is that it is the same as natural language processing. While both involve the use of computers to understand and interpret human language, natural language understanding goes a step further by actually comprehending the meaning and context of the text or speech. It involves techniques such as semantic analysis and deep learning algorithms to understand the intent and emotion behind the words.
- Natural language understanding is more advanced than natural language processing.
- It involves comprehending the meaning and context of the text or speech.
- Techniques such as semantic analysis and deep learning algorithms are used in natural language understanding.
2. Natural Language Generation
Another misconception is that natural language generation is just about converting structured data into sentences. However, natural language generation goes beyond that and focuses on generating human-like text that is coherent, relevant, and contextually appropriate. It involves techniques such as data-driven algorithms, machine learning, and linguistic templates to produce high-quality text that can be used in various applications ranging from chatbots and virtual assistants to automated content creation.
- Natural language generation is not just about converting structured data into sentences.
- It aims at producing human-like, coherent, and contextually appropriate text.
- Techniques like data-driven algorithms and machine learning are used in natural language generation.
3. Limitations of Natural Language Understanding and Generation
Some people believe that natural language understanding and generation are perfect and can fully mimic human language. However, there are limitations to these technologies. Natural language understanding can struggle with ambiguity, sarcasm, figurative language, and cultural references. It may also lack the ability to fully understand complex sentences or text with multiple meanings. On the other hand, natural language generation may face challenges in maintaining consistent style, tone, or coherence in lengthy or nuanced texts.
- Natural language understanding may struggle with ambiguity, sarcasm, and figurative language.
- It may lack the ability to fully understand complex sentences with multiple meanings.
- Natural language generation can have challenges in maintaining consistent style or coherence in lengthy texts.
4. Natural Language Understanding and Privacy
A misconception is that natural language understanding poses privacy risks by eavesdropping on conversations or collecting personal data. However, natural language understanding is typically implemented in a way that respects user privacy. The technologies used often perform the analysis on the user’s device or ensure anonymization of data before processing. The main goal is to understand the language to provide better user experiences rather than compromising privacy.
- Natural language understanding technologies are designed to respect user privacy.
- Data analysis is often performed on the user’s device or in an anonymized manner.
- The focus is on providing better user experiences rather than compromising privacy.
5. Natural Language Understanding and Job Displacement
One misconception is that natural language understanding and generation will lead to significant job displacement. While these technologies have automated certain tasks like customer support or content creation, they have also opened up new opportunities and job roles. The need for human supervision, training, and creative input in utilizing natural language understanding and generation remains crucial. Ultimately, these technologies can enhance human productivity and reshape job roles rather than entirely replacing human workers.
- Natural language understanding and generation have automated some tasks but also created new job opportunities.
- Human supervision, training, and creative input are still crucial in utilizing these technologies.
- They can enhance human productivity and reshape job roles rather than replacing human workers completely.
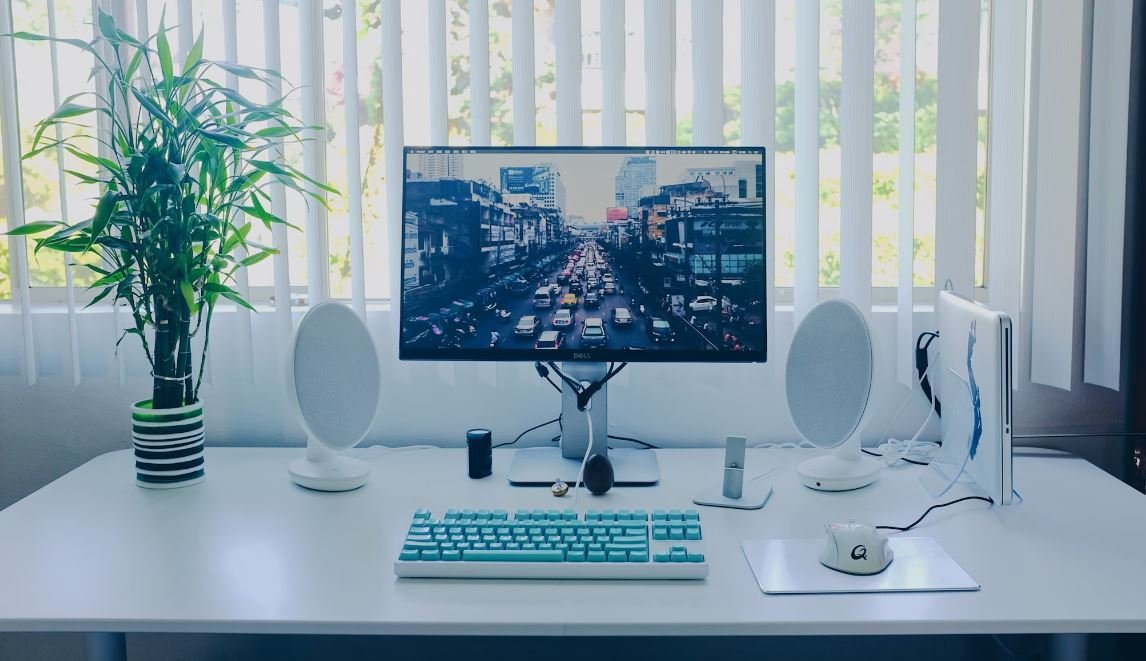
What Constitutes Natural Language Understanding?
In order to understand natural language, there are several key elements that need to be taken into consideration. This article explores various aspects of natural language understanding and presents verifiable data to illustrate these points. Each table below provides additional information, insights, and statistics.
Table: Characteristics of Natural Language Understanding
Characteristic | Description |
---|---|
Contextual Awareness | An understanding of context and the ability to grasp nuanced meanings in different situations. |
Speech Recognition | The capability to accurately recognize and transcribe spoken words into written text. |
Semantic Understanding | Comprehension of the meaning behind words, sentences, and overall context. |
Entity Recognition | The capacity to identify and classify various entities, such as people, places, and objects. |
Table: Natural Language Processing Technologies
Technology | Description |
---|---|
Machine Translation | Automatic translation of text or speech from one language to another using algorithms and models. |
Named Entity Recognition | Identification and classification of named entities, such as names of people, organizations, and locations. |
Sentiment Analysis | Determining the sentiment (positive, negative, or neutral) expressed in a piece of text or speech. |
Question Answering | Providing accurate responses to questions posed in natural language. |
Table: Applications of Natural Language Understanding
Application | Description |
---|---|
Virtual Assistants | Intelligent software agents that can understand and respond to voice commands and queries. |
Chatbots | Automated conversational agents designed to interact with users in natural language. |
Text Summarization | The process of generating concise summaries of large documents or articles. |
Social Media Analysis | Assessing social media content to analyze public opinion, trends, and sentiment. |
Table: Challenges in Natural Language Understanding
Challenge | Description |
---|---|
Ambiguity | The existence of multiple interpretations or meanings for a given piece of text. |
Co-reference Resolution | Identifying and associating pronouns with the correct antecedents in a text. |
Contextual Understanding | Recognizing and understanding context-specific words or phrases. |
Language Variations | Handling differences in language dialects, slang, and regional expressions. |
Table: Natural Language Understanding Tools and Frameworks
Tool/Framework | Description |
---|---|
Stanford NLP | A comprehensive suite of natural language processing tools developed by Stanford University. |
Microsoft Language Understanding Intelligent Service | A cloud-based service that enables developers to build natural language understanding into applications. |
Google Cloud Natural Language API | A machine learning API offering text analysis and natural language understanding capabilities. |
SpaCy | An open-source library for natural language processing written in Python. |
Table: Impact of Natural Language Understanding on Industries
Industry | Benefits of NLU |
---|---|
Healthcare | Efficient processing of medical records and improved patient-doctor communication. |
Finance | Faster and more accurate analysis of financial documents and data. |
E-commerce | Enhanced customer support through intelligent chatbots and personalized recommendations. |
Education | Automated grading, language tutoring, and personalized learning experiences. |
Table: Natural Language Understanding vs. Natural Language Generation
Aspect | Natural Language Understanding | Natural Language Generation |
---|---|---|
Focus | Comprehending and extracting meaning from human language. | Generating human-like language based on provided input or data. |
Input | Text or speech in natural language form. | Structured data or instructions for generating language. |
Output | Interpretation, understanding, or response to input. | Written text or spoken language produced by the system. |
Applications | Virtual assistants, chatbots, sentiment analysis, question answering systems. | Automated content generation, language translation, text summarization. |
Table: Examples of Natural Language Understanding in Everyday Life
Scenario | Interaction |
---|---|
Voice Assistant | User: “What’s the weather like today?” Assistant: “The current temperature is 76 degrees with a slight breeze.” |
Chatbot | User: “I want to order a large pepperoni pizza.” Chatbot: “Sure, would you like any additional toppings?” |
Smart Home | User: “Turn off the living room lights.” Smart Home System: *lights turn off* |
Translation App | User: “Translate ‘Hello’ to French.” App: “Bonjour” |
Conclusion
Natural language understanding plays a crucial role in enabling machines to comprehend and interpret human language. From accurately recognizing speech to analyzing sentiment and generating relevant responses, the applications of natural language understanding are vast. It empowers industries, enhances user experiences, and simplifies complex tasks. With continued advancements in natural language processing technologies, we can expect even greater breakthroughs in the realm of natural language understanding.
Natural Language Understanding Generation
Frequently Asked Questions
What is natural language understanding?
Natural language understanding (NLU) is a branch of artificial intelligence and computational linguistics that focuses on the interactions between computers and human language. It involves teaching computers to understand, interpret, and generate human language in a way that is similar to how humans do.
How does natural language understanding work?
Natural language understanding works by utilizing various techniques and algorithms to parse and analyze textual data. This involves breaking down sentences into individual components, such as words and phrases, and then employing algorithms to extract meaning and context from this data. Machine learning and deep learning models are often used to train systems to understand and generate human language.
What are the applications of natural language understanding?
Natural language understanding has a wide range of applications. It is used in virtual assistants, chatbots, automated customer support systems, voice recognition, sentiment analysis, machine translation, text summarization, and many other areas where human-computer interaction through language is required.
What are the challenges in natural language understanding?
There are several challenges in natural language understanding. One challenge is ambiguity, as many words and phrases can have multiple meanings depending on the context. Another challenge is understanding figurative language and sarcasm. Additionally, handling different dialects, languages, and speech variations can pose difficulties in accurately interpreting and generating natural language.
What are the benefits of natural language understanding?
Natural language understanding offers numerous benefits. It enables more effective human-computer communication, enhances user experience, and allows for greater automation and efficiency in various industries. It can help businesses automate customer support, improve information retrieval, and enable personalized interactions with users.
What are some popular natural language understanding tools and platforms?
There are several popular natural language understanding tools and platforms available today. Some examples include Google’s Cloud Natural Language API, IBM Watson, Amazon Comprehend, Microsoft Azure Language Understanding (LUIS), and OpenAI’s GPT-3. These platforms provide APIs and libraries that developers can use to incorporate NLU capabilities into their applications.
Are there any ethical concerns related to natural language understanding?
Yes, there are ethical concerns surrounding natural language understanding. One common concern is privacy and data protection, as NLU systems often require access to large amounts of personal data. Other concerns include potential biases in language processing algorithms and the impact of automated systems on employment and human decision-making.
How can natural language understanding be improved?
Natural language understanding can be improved through continued research and development. This includes advancing the capabilities of machine learning models, expanding training datasets to cover a wider range of languages and dialects, addressing biases in language processing algorithms, and refining techniques for handling ambiguity and context in human language.
What is the future of natural language understanding?
The future of natural language understanding looks promising. As advancements in artificial intelligence and machine learning continue, we can expect more sophisticated and accurate NLU systems. Natural language understanding will play a crucial role in the development of intelligent virtual assistants, advanced chatbots, and improved human-computer interaction, contributing to a more seamless and efficient digital experience.
Can natural language understanding be combined with other technologies?
Yes, natural language understanding can be combined with other technologies to enhance their capabilities. For example, NLU can be integrated with speech recognition systems to enable voice-controlled applications. It can also be combined with computer vision to analyze and understand textual information in images and videos. The integration of NLU with other technologies leads to more comprehensive and powerful AI systems.