NLP Healthcare
Natural Language Processing (NLP) is revolutionizing healthcare by enabling computers to understand human language, extract meaningful information from text, and derive insights to improve patient care and enhance decision-making. With the exponential growth of electronic health records and medical literature, NLP techniques offer unprecedented opportunities for data analysis, healthcare management, and research advancement.
Key Takeaways:
- NLP improves healthcare by extracting valuable information from text.
- NLP aids in data analysis, healthcare management, and research.
- NLP enhances decision-making and patient care.
The Power of NLP in Healthcare
**Natural Language Processing (NLP)**, a subset of artificial intelligence, uses advanced algorithms to analyze and interpret human language. *NLP tools help healthcare professionals derive insights from unstructured data, such as clinical notes, scientific articles, and social media posts, enabling them to make informed decisions and devise personalized treatment plans for patients.* By processing vast amounts of textual information quickly and accurately, NLP empowers healthcare organizations to discover patterns, identify trends, and uncover hidden knowledge within their data.
NLP techniques can be applied to various healthcare-related tasks, including but not limited to:
- **Clinical Decision Support Systems**: NLP assists in diagnosing diseases, predicting outcomes, and suggesting appropriate treatments based on patient data and medical literature.
- **Electronic Health Record (EHR) Analysis**: NLP simplifies the process of extracting and aggregating patient information from EHRs, enabling efficient analysis and improving patient care.
- **Pharmacovigilance**: NLP helps in monitoring and analyzing adverse drug reactions and identifying potential safety concerns associated with medications.
- **Medical Research**: NLP aids in literature review, information extraction, and knowledge discovery from scientific articles, potentially accelerating research and innovation in healthcare.
NLP Applications in Healthcare
Let’s delve into some specific applications of NLP in healthcare:
Table 1: Applications of NLP in Healthcare
Application | Description |
---|---|
Clinical Documentation Improvement | NLP algorithms analyze clinical notes to identify missing or inaccurate information, improving coding accuracy and reimbursement. |
Sentiment Analysis | NLP techniques analyze patient feedback and social media posts to gauge public opinion, sentiment, and satisfaction with healthcare services. |
Health Information Extraction | NLP tools extract relevant information, such as medications, dosage, and lab results, from textual data to populate EHRs accurately and efficiently. |
In addition to these applications, NLP is also utilized in entity recognition, named entity resolution, question answering, summarization, and translation tasks.
Table 2: Benefits of NLP in Healthcare
Benefit | Description |
---|---|
Improved Efficiency | NLP automates manual tasks, such as data entry and coding, leading to time and cost savings for healthcare providers. |
Enhanced Decision-Making | NLP facilitates evidence-based decision-making by providing clinicians with timely access to relevant information and medical literature. |
Personalized Medicine | NLP helps tailor treatments to individual patients by analyzing their medical history, genetic data, and other relevant factors. |
Future Directions and Challenges
Despite the tremendous potential of NLP in healthcare, several challenges need to be addressed for its wider adoption:
- The need for large and diverse datasets that capture the complexity and variability of healthcare language.
- The protection of patient privacy and ensuring compliance with data protection regulations.
- Addressing bias in NLP models to avoid healthcare disparities.
Challenge | Description |
---|---|
Interoperability | Integrating NLP solutions with existing healthcare systems and ensuring seamless data exchange. |
Ethical Use of Data | Establishing guidelines and frameworks to ensure the ethical use of patient data and protect individual privacy. |
Continual Model Improvement | The constant need for training and refining NLP models to achieve higher accuracy and reliability in healthcare settings. |
In conclusion, NLP has the potential to revolutionize healthcare by unlocking valuable insights from unstructured data and facilitating improved decision-making and patient care. As the technology continues to advance, addressing the challenges related to data, privacy, bias, interoperability, and model improvement will be crucial to fully harness the power of NLP in healthcare.
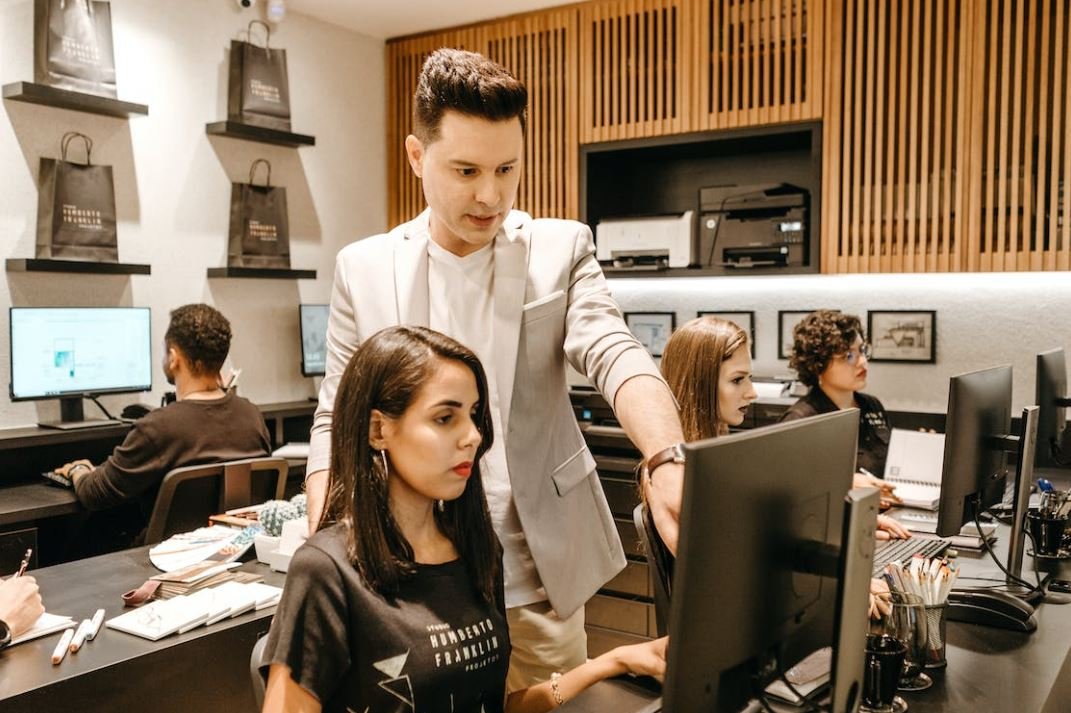
Common Misconceptions
Misconception 1: NLP in Healthcare lacks accuracy
There is a common misconception that NLP (Natural Language Processing) in healthcare lacks accuracy. However, this is not entirely true. While NLP algorithms may not be perfect, significant progress has been made in improving their accuracy over the years.
- NLP algorithms have been extensively trained on large amounts of healthcare data to enhance accuracy.
- The accuracy of NLP models can be further improved through continuous evaluation and refinement.
- NLP healthcare applications are constantly evolving, leading to improved accuracy and precision in extracting valuable insights from medical text.
Misconception 2: NLP in Healthcare is a threat to patient privacy
Another common misconception surrounding NLP in healthcare is that it poses a threat to patient privacy. While it is true that NLP involves analyzing and processing large amounts of textual data, strict privacy measures are in place to protect patient information.
- Data anonymization techniques are employed to remove any personally identifiable information from healthcare text before analysis.
- Healthcare organizations and NLP developers adhere to strict regulatory guidelines, such as HIPAA, to ensure patient privacy and data protection.
- NLP algorithms are designed to focus on the content and patterns within medical text rather than individual patient identities, further safeguarding privacy.
Misconception 3: NLP in Healthcare will replace human medical professionals
Contrary to popular belief, NLP in healthcare is not meant to replace human medical professionals. Instead, it serves as a valuable tool to augment their capabilities and improve overall efficiency and accuracy in medical decision-making.
- NLP in healthcare is designed to assist physicians and researchers in extracting valuable insights from vast amounts of medical text, which would be time-consuming for humans alone.
- Medical professionals remain crucial for interpreting and validating information extracted through NLP techniques in healthcare.
- NLP algorithms can identify patterns and correlations in data, but it is the medical professionals who ultimately make decisions based on these findings.
Misconception 4: NLP focuses solely on clinical documentation
One common misconception is that NLP in healthcare solely focuses on clinical documentation, such as medical reports and records. While clinical documentation is a significant application area, NLP has a much broader scope and potential in healthcare.
- NLP can be used to analyze patient feedback, social media data, and other unstructured healthcare text to gain insights into patient experiences and sentiments.
- It can facilitate the extraction of relevant information from medical literature for evidence-based medicine and research.
- NLP techniques can assist in identifying adverse drug reactions, predicting disease outbreaks, and even improving healthcare delivery through analysis of unstructured data from various sources.
Misconception 5: NLP in Healthcare is only applicable in developed countries
One common misconception is that NLP in healthcare is only applicable in developed countries where electronic health records (EHRs) are prevalent. However, NLP techniques have proven their value in various healthcare settings worldwide, including those with limited resources.
- NLP can assist in processing healthcare text in any language, enabling global application in diverse healthcare settings.
- Even without extensive EHR systems, NLP algorithms can extract insights from alternative data sources, such as medical literature and local health surveys.
- NLP technologies can be adapted to suit the specific needs and resources of different healthcare systems, promoting widespread utilization and benefits.
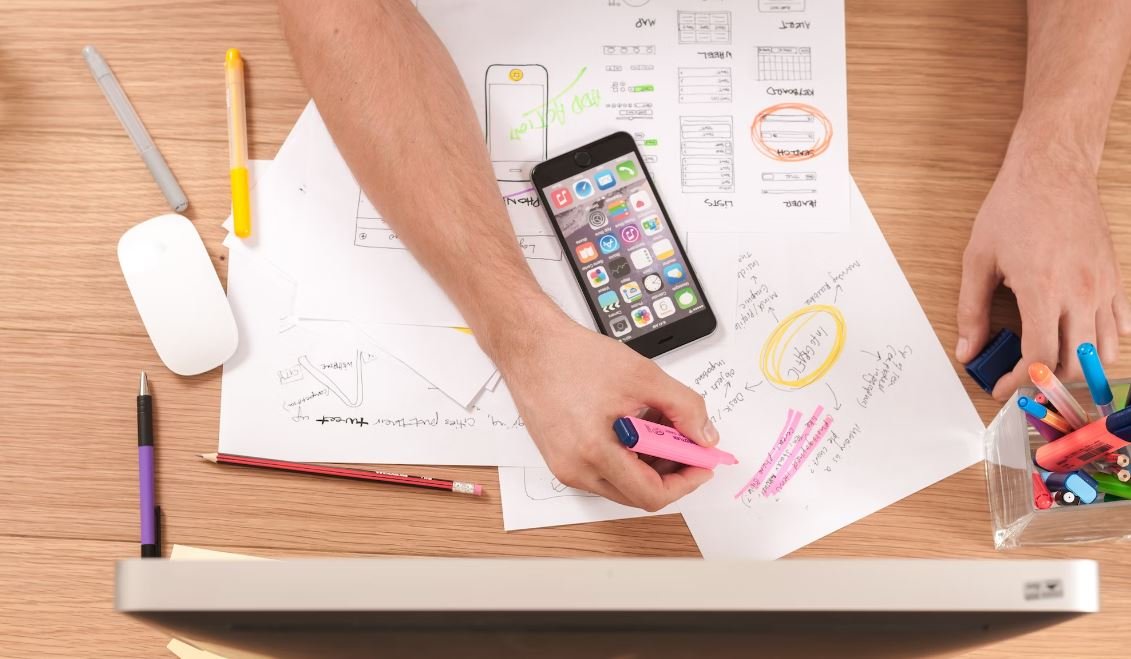
Natural Language Processing in Healthcare: A Game-Changer for Patient Care
In recent years, the application of Natural Language Processing (NLP) in healthcare has revolutionized the way patient data is analyzed and processed. NLP techniques, combined with advanced algorithms, enable healthcare professionals to extract valuable insights from vast amounts of textual data, such as patient records, clinical notes, and research papers. This article highlights ten key areas where NLP is making a significant impact on improving patient outcomes and transforming the healthcare industry as a whole.
1. Early Disease Detection
By leveraging NLP, medical researchers can identify subtle linguistic cues and patterns in patients’ electronic health records (EHR) to aid in early disease detection. For example, analyzing changes in language patterns, tone, and sentiment of individuals with mental health conditions could assist in identifying individuals at risk or in need of immediate intervention.
2. Medical Literature Analysis
NLP techniques facilitate the analysis of a vast amount of medical literature, allowing healthcare professionals and researchers to quickly extract relevant information for clinical decision-making. Through automated literature reviews, NLP helps identify new treatment approaches, drug interactions, or adverse effects, ultimately improving patient care.
3. Patient Sentiment Analysis
NLP enables healthcare providers to gain insights into patient sentiment by analyzing patient feedback, online reviews, and social media posts. This data can help identify areas for improvement in healthcare services, enhance patient satisfaction, and foster patient engagement.
4. Adverse Drug Event Identification
Using NLP algorithms, healthcare systems can identify adverse drug events by analyzing patient records and reports. This helps healthcare professionals promptly recognize potential adverse effects, contributing to improved patient safety and better-informed medication prescribing.
5. Clinical Decision Support Systems
NLP-powered clinical decision support systems assist healthcare professionals in making accurate and evidence-based decisions. By analyzing patient data in real-time, these systems can suggest treatment plans, alert to potential drug interactions, and assist in diagnosis, ultimately enhancing the quality of care delivered to patients.
6. Streamlining Medical Coding
NLP algorithms simplify medical coding by automatically extracting relevant information from clinical documentation. These algorithms identify diagnoses, procedures, and other essential components, reducing the burden on medical coders and enhancing coding accuracy.
7. Predictive Analytics
NLP-based predictive analytics models can forecast patient outcomes, readmissions, and disease progression. By analyzing a patient’s medical history, demographics, and linguistic patterns, healthcare providers can tailor interventions and preventive measures, leading to better patient outcomes.
8. Health Monitoring through Wearables
Wearable devices equipped with NLP capabilities enable real-time monitoring of patients’ vitals, symptoms, and daily activities. By continuously analyzing data captured from wearables, healthcare professionals can gain actionable insights and promptly intervene when critical health indicators are detected.
9. Automated Transcription Services
NLP-driven automated transcription services transcribe patient-doctor conversations and clinical notes accurately. These services significantly reduce administrative burdens, improve documentation speed, and enhance the patient-physician interaction.
10. Personalized Medicine
NLP algorithms contribute to the establishment of personalized medicine by extracting relevant patient data and identifying specific treatment options based on an individual’s characteristics and medical history. This approach ensures targeted therapies, enhances treatment efficacy, and reduces the risk of adverse effects.
Conclusion
The integration of Natural Language Processing (NLP) into healthcare has ushered in a new era where patient data can be efficiently analyzed, leading to improved patient care and outcomes. From early disease detection to personalized medicine, the applications of NLP in healthcare are diverse and far-reaching. By harnessing the power of NLP, healthcare professionals can leverage the vast amount of textual data available to make informed decisions, streamline processes, and ultimately provide better care to patients.
Frequently Asked Questions
What is Natural Language Processing (NLP)?
What is Natural Language Processing (NLP)?
How is NLP used in healthcare?
How is NLP used in healthcare?
What are some applications of NLP in healthcare?
What are some applications of NLP in healthcare?
How does NLP aid in clinical decision support?
How does NLP aid in clinical decision support?
What challenges are associated with NLP in healthcare?
What challenges are associated with NLP in healthcare?
How is NLP used in patient monitoring?
How is NLP used in patient monitoring?
Can NLP be used for detecting medical errors?
Can NLP be used for detecting medical errors?
What are the benefits of using NLP in healthcare?
What are the benefits of using NLP in healthcare?
What is clinical coding and how does NLP assist in it?
What is clinical coding and how does NLP assist in it?
How can sentiment analysis be helpful in healthcare?
How can sentiment analysis be helpful in healthcare?