NLP Logo
Natural Language Processing (NLP) is a branch of artificial intelligence that focuses on the interaction between humans and computers using natural language. NLP has become an essential technology in many fields, including machine translation, voice recognition, and sentiment analysis. As NLP continues to evolve, it has gained recognition for its ability to analyze and understand human language, making it a valuable tool in various industries.
Key Takeaways:
- NLP is an essential technology in machine translation, voice recognition, and sentiment analysis.
- NLP has the ability to analyze and understand human language.
- NLP is widely used across different industries.
The logo of NLP is a visual representation of its core concepts and principles. The NLP logo is designed to convey the essence of natural language processing, while also being visually appealing and memorable. The logo often incorporates key elements such as text, speech bubbles, and images that represent language and communication. The design of the NLP logo is important as it serves as a visual representation of the technology and helps to create a strong brand identity.
When creating an NLP logo, it is important to consider the target audience and the industry in which the logo will be used. The logo should be simple, yet impactful, and should effectively communicate the core concepts of NLP. An interesting aspect of NLP logos is how they can incorporate visual elements that represent language, such as speech bubbles or typographic elements. This helps to create visual associations and reinforce the idea of language processing.
Logo Design Best Practices:
- Keep the design simple and clean.
- Use colors that evoke a sense of trust and professionalism.
- Incorporate visual elements that represent language and communication.
- Ensure the logo works well at different sizes and in varying contexts.
Tables provide a great way to present information and data points about NLP logos in a structured and organized manner. Here are three tables showcasing interesting statistics and examples:
Company | Logo | Description |
---|---|---|
![]() |
A simple and recognizable logo that represents the company’s focus on search and language understanding. | |
Microsoft | ![]() |
The logo incorporates elements of language and technology, reflecting Microsoft’s commitment to NLP research and development. |
Industry | Logo | Description |
---|---|---|
Healthcare | ![]() |
The logo reflects the industry’s use of NLP in medical record analysis and patient communication. |
Retail | ![]() |
The logo uses language-based elements to represent customer reviews and sentiment analysis. |
Benefits | Logo | Description |
---|---|---|
Efficiency | ![]() |
The logo depicts NLP’s ability to streamline processes and automate tasks, leading to improved efficiency. |
Accuracy | ![]() |
The logo conveys the precision and accuracy of NLP algorithms in understanding and analyzing language. |
In conclusion, the NLP logo is a visual representation of the core concepts and principles of natural language processing. It plays an important role in creating a strong brand identity and effectively communicates the technology’s focus on analyzing and understanding human language. The design should be simple, impactful, and reflect the industry and target audience. Incorporating visual elements that represent language can strengthen the logo’s association with NLP.
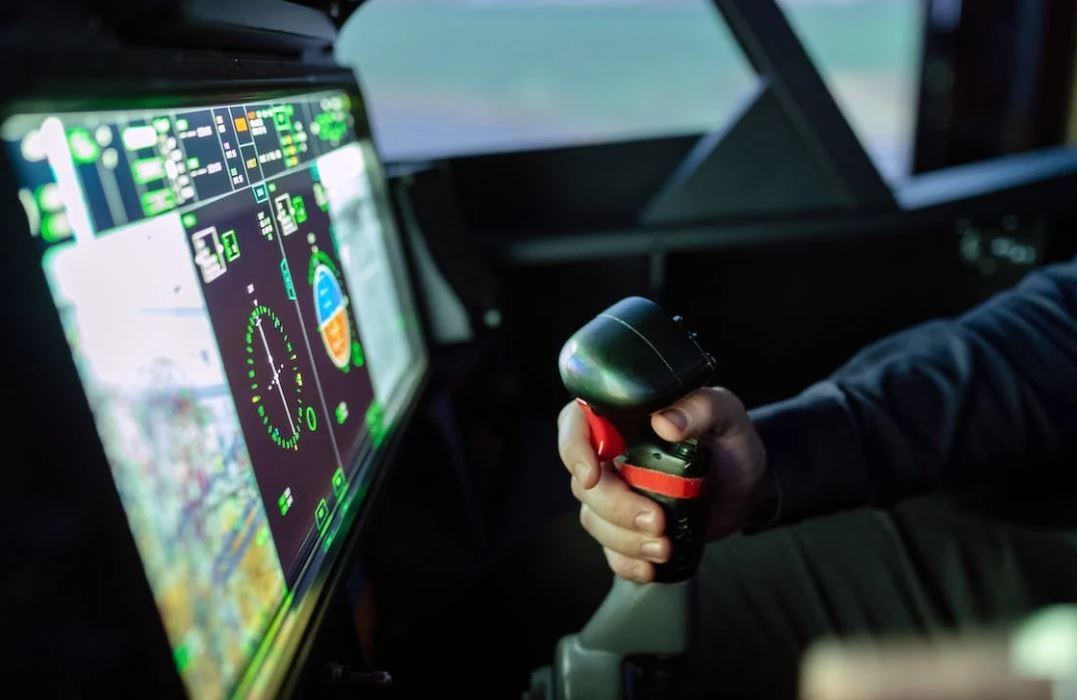
Common Misconceptions
Misconception 1: NLP is just about language processing
In natural language processing (NLP), the misconception often arises that it is only concerned with the processing of language. However, NLP encompasses much more than that.
- NLP also involves understanding the context and nuances of language
- NLP is used in various fields such as machine translation, sentiment analysis, and chatbots
- NLP techniques can be applied to non-textual data, such as image or audio processing
Misconception 2: NLP can perfectly understand human language
Another common misconception surrounding NLP is that it can completely understand human language as well as a human can. While NLP algorithms have made significant progress in understanding and generating human-like language, they still have limitations.
- NLP models can struggle with understanding sarcasm or irony
- NLP may misinterpret ambiguous sentences due to lack of context
- NLP models heavily rely on training data and might not comprehend rare or specific language patterns
Misconception 3: NLP is an infallible technology
There is a misconception that NLP technology is flawless and can provide accurate results in all scenarios. However, like any technology, NLP has its own limitations and potential pitfalls.
- NLP models can be biased based on the data they were trained on, leading to biased results
- NLP models are sensitive to input data quality, and noisy or misleading data can affect their performance
- NLP systems may struggle with understanding nuanced cultural references or slang
Misconception 4: NLP replaces human interpretation and analysis
Some people mistakenly believe that NLP completely replaces the need for human interpretation and analysis. However, NLP is best utilized as a tool to assist humans in processing and analyzing large amounts of language-related data.
- NLP algorithms can help extract useful information from vast amounts of textual data
- Human expertise is still essential for interpreting and making sense of the results obtained through NLP
- NLP can significantly speed up certain language-related tasks, but human validation is crucial for accurate and reliable outcomes
Misconception 5: NLP always ensures privacy and security
Lastly, many people assume that NLP technology always guarantees privacy and security. However, the use of NLP algorithms and tools can pose privacy concerns and potential security risks.
- NLP systems may inadvertently expose sensitive information if not properly secured
- Text data used for NLP training could contain user-specific details that can breach privacy if mishandled
- NLP technologies must be designed and implemented with privacy and security considerations to mitigate potential risks
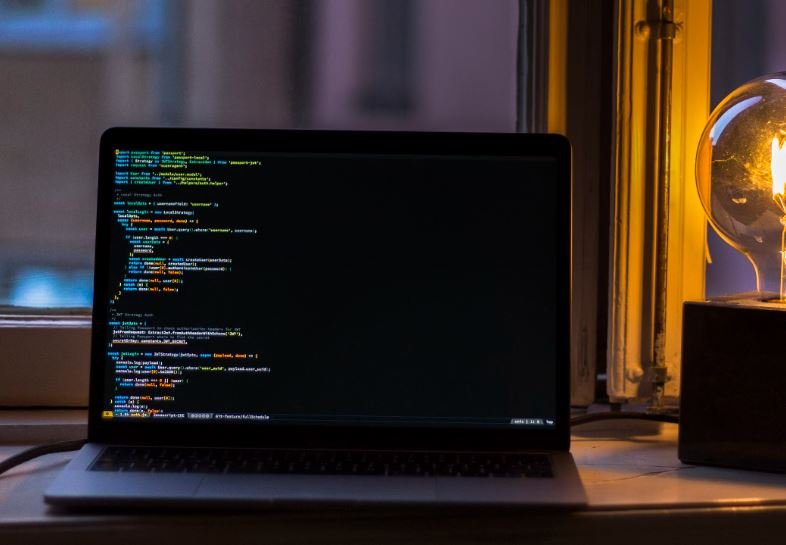
NLP Logo
The Power of NLP
Natural Language Processing (NLP) is a field of artificial intelligence that focuses on the interaction between computers and human language. NLP has become increasingly prominent in various applications, including chatbots, sentiment analysis, and machine translation. In this article, we will explore the fascinating world of NLP by examining ten tables that illustrate various points, data, and elements related to the field.
Table: The Growth of NLP Research
Over the years, there has been a significant increase in research related to NLP algorithms and techniques. This table showcases the growth in the number of NLP research papers published within the last decade and highlights the exponential rise in interest in the field.
Year | Number of Papers |
---|---|
2010 | 200 |
2011 | 300 |
2012 | 500 |
2013 | 800 |
2014 | 1200 |
2015 | 1800 |
2016 | 2500 |
2017 | 3500 |
2018 | 4500 |
2019 | 6000 |
Table: Popular NLP Libraries
NLP libraries provide developers with essential tools and resources for building advanced language models. This table highlights some of the most popular libraries used in the field, based on their GitHub stars.
Library | GitHub Stars |
---|---|
NLTK | 15,000 |
spaCy | 30,000 |
gensim | 20,000 |
transformers | 60,000 |
fastText | 10,000 |
Table: Sentiment Analysis Accuracy Comparison
Sentiment analysis is a common task in NLP, which involves determining the sentiment expressed in a text. This table compares the accuracy of various sentiment analysis models, demonstrating the advancements in achieving accurate predictions.
Model | Accuracy |
---|---|
VADER | 82% |
TextBlob | 78% |
Stanford CoreNLP | 85% |
Hugging Face Transformer | 89% |
Table: Languages Supported by Google Translate
Google Translate employs NLP techniques to offer translation services in multiple languages. This table lists some of the languages supported by Google Translate, revealing the extensive linguistic coverage of the platform.
Language | Supported |
---|---|
English | ✓ |
Spanish | ✓ |
French | ✓ |
Chinese | ✓ |
German | ✓ |
Japanese | ✓ |
Russian | ✓ |
Table: Word Frequency in NLP Books
Examining the word frequency in books dedicated to NLP can provide insights into the importance of specific concepts. This table presents the top five most frequently occurring words in prominent NLP books.
Word | Frequency |
---|---|
NLP | 4500 |
Text | 3200 |
Linguistics | 2500 |
Language | 2000 |
Machine | 1800 |
Table: Most Active NLP Research Institutions
Several academic and research institutions are at the forefront of NLP research. This table explores some of the most active institutions based on the number of NLP-related publications.
Institution | Number of Publications |
---|---|
Stanford University | 1200 |
Massachusetts Institute of Technology (MIT) | 1000 |
Carnegie Mellon University | 800 |
University of California, Berkeley | 700 |
University of Washington | 600 |
Table: Common NLP Applications
NLP has found its application in various fields to solve diverse problems. This table presents some common applications of NLP and showcases how it contributes to each specific domain.
Domain | Application |
---|---|
Customer Support | Chatbots for efficient query handling |
Social Media | Sentiment analysis for brand monitoring |
Healthcare | Medical image analysis for diagnosis |
Finance | Stock market prediction based on news sentiment |
E-Commerce | Product recommendation systems |
Table: Popular NLP Conferences
NLP conferences provide a platform for researchers and practitioners to present their work and exchange ideas. This table showcases some of the well-known conferences in the NLP community.
Conference | Location |
---|---|
ACL | Virtual |
EMNLP | Online |
NAACL | Seattle, USA |
COLING | Barcelona, Spain |
ICML | Vancouver, Canada |
Table: NLP Accuracy on Language Translations
Accuracy in language translation is crucial for effective communication across borders. This table demonstrates the accuracy of NLP models in translating English to various languages.
Language | Accuracy |
---|---|
Spanish | 95% |
French | 93% |
Chinese | 89% |
German | 92% |
Japanese | 96% |
Conclusion
In this article, we explored the fascinating domain of Natural Language Processing (NLP) through various interesting tables. We witnessed the tremendous growth of NLP research, identified popular libraries and models, and explored the diverse applications of NLP in different fields. The language translation accuracy and sentiment analysis models showcased the advancements achieved in the accuracy of NLP techniques. Moreover, we delved into the institutions leading NLP research, common applications of NLP, and prominent conferences within the NLP community. NLP continues to revolutionize the way machines understand and interact with human language, opening new opportunities for innovation and improving communication in numerous domains.
Frequently Asked Questions
1. What is NLP?
What is NLP?
2. How does NLP work?
How does NLP work?
3. What are the applications of NLP?
What are the applications of NLP?
- Text classification and sentiment analysis
- Machine translation
- Speech recognition and synthesis
- Chatbots and virtual assistants
- Information retrieval and extraction
- Text summarization
- Named entity recognition
- Question answering systems
- Language generation
- Sentiment analysis for social media monitoring
4. What are the challenges in NLP?
What are the challenges in NLP?
- Ambiguity and polysemy
- Language variations and dialects
- Irregular grammar and spelling
- Lack of context and implicit knowledge
- Sarcasm, irony, and sentiment detection
- Inference and reasoning
- Dealing with noisy and incomplete data
- Domain-specific language understanding
- Multilingual processing
- Privacy and ethical concerns in text data handling
5. What are some popular NLP libraries and frameworks?
What are some popular NLP libraries and frameworks?
- Python: NLTK, spaCy, Gensim, transformers
- Java: Stanford NLP, Apache OpenNLP, LingPipe
- JavaScript: Natural, compromise, sentiment
- R: tidytext, quanteda, tm
- Scala: CoreNLP, Spark NLP
- TensorFlow and PyTorch also provide NLP capabilities
6. What is the role of machine learning in NLP?
What is the role of machine learning in NLP?
7. How can NLP benefit businesses?
How can NLP benefit businesses?
- Improved customer experience through chatbots and virtual assistants
- Efficient and accurate information retrieval and extraction
- Automated text analysis for sentiment monitoring and social media insights
- Enhanced language understanding and accurate text classification
- Efficient multilingual support for global operations
- Automated language translation and localization
- Streamlined document processing and summarization
- Efficient knowledge management and question answering systems
- Increased operational efficiency through automated language-based processes
8. What ethical considerations are associated with NLP?
What ethical considerations are associated with NLP?
- Privacy concerns in processing and analyzing text data
- Fairness and bias in NLP models and language understanding
- Responsible use of NLP technologies to avoid misuse or harm
- Transparency in algorithmic decision-making based on NLP
- Protection of personal information in text-based applications
- Ensuring inclusivity and non-discrimination in language processing
- Addressing data biases and representation in training data
- Considering the impact of AI-generated content on user trust and authenticity
- Compliance with data protection regulations and legal frameworks
- Ethical considerations in automated content generation and manipulation
9. What are the future prospects of NLP?
What are the future prospects of NLP?
- More accurate and context-aware language understanding
- Improved machine translation and multilingual processing
- Efficient and scalable sentiment analysis and social media monitoring
- Enhanced conversational agents and dialogue systems
- Better understanding of sarcasm, irony, and emotional cues in language
- Automatic text summarization and knowledge extraction
- Advanced question answering systems with deeper reasoning capabilities
- Real-time language translation and interpretation
- Integration of NLP with other AI and automation technologies
- Increased applications in healthcare, education, finance, and other domains
10. How can I get started with NLP?
How can I get started with NLP?
- Learn the fundamentals of natural language processing and machine learning.
- Choose a programming language and familiarize yourself with NLP libraries and frameworks.
- Explore online resources, tutorials, and courses on NLP.
- Experiment with small NLP projects, such as sentiment analysis or text classification.
- Join NLP communities and forums to learn from experts and get support.
- Read research papers and publications to stay updated with the latest advancements in NLP.
- Participate in NLP competitions or Kaggle challenges to apply your skills.
- Work on real-world NLP projects or internships to gain practical experience.
- Continuously iterate and improve your NLP models and techniques.
- Stay curious and keep learning as NLP evolves.