Is NLP Part of AI?
Natural Language Processing (NLP) and Artificial Intelligence (AI) are two terms commonly heard in tech circles, but what is the relationship between the two? Many people wonder if NLP is a subset of AI or if they are separate entities altogether. In this article, we will explore the connection between NLP and AI and shed light on their roles in the world of technology.
Key Takeaways
- NLP and AI are closely connected technologies.
- NLP focuses on processing and understanding human language.
- AI encompasses a broader spectrum of technologies and applications.
Natural Language Processing (NLP) is a field of study that combines linguistics and computer science to enable computers to understand, interpret, and generate human language. It involves teaching machines to read, listen, and respond to humans in a way that is similar to how people communicate with each other. **NLP** algorithms analyze vast amounts of text data, enabling machines to extract meaning, classify sentiments, and respond intelligently.
*NLP allows machines to comprehend the complexity of human language, including its nuances, idioms, and slang.*
Artificial Intelligence (AI), on the other hand, is an umbrella term that encompasses a wide range of technologies and applications that aim to simulate human intelligence. AI systems exhibit capabilities such as learning, reasoning, problem-solving, and understanding. **AI** enables machines to perform tasks that typically require human intelligence, including visual perception, speech recognition, translation, and decision-making.
*AI drives the development of intelligent systems that provide human-like functionalities.*
NLP forms an integral part of AI, as it enables machines to understand and generate human language, which is essential for effective communication and interaction with users. However, AI extends beyond just language processing and includes other technologies like computer vision, machine learning, robotics, and more. **AI** provides the framework for NLP to function as part of a broader set of intelligent capabilities.
NLP | AI |
---|---|
Focuses on language processing and comprehension. | Encompasses various technologies simulating human intelligence. |
Used for sentiment analysis, language generation, and information retrieval. | Applied in autonomous vehicles, virtual assistants, and cybersecurity. |
*NLP and AI work hand in hand, leveraging each other’s capabilities to create intelligent systems that can understand, process, and respond to human language.*
NLP and AI have revolutionized various industries and transformed the way we interact with technology. They have paved the way for virtual assistants like Siri and Alexa, enhanced online translation services, and enabled sentiment analysis for businesses. These technologies continue to advance at a rapid pace, promising exciting new possibilities in the future.*
Conclusion
In conclusion, NLP is not a separate entity from AI but rather an integral part of it. NLP focuses on language processing and comprehension, while AI encompasses a broader spectrum of technologies related to simulating human intelligence. Together, they empower machines to analyze, understand, and generate human language, enabling more effective communication between humans and technology.
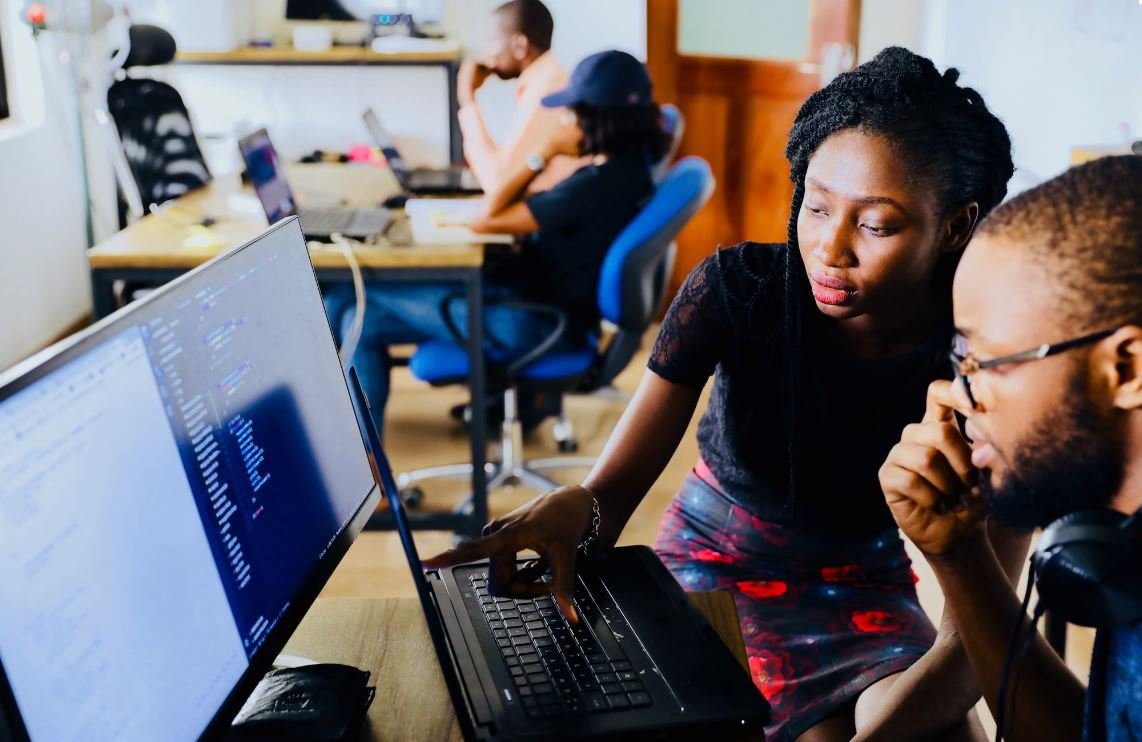
Common Misconceptions
Is NLP Part of AI?
One common misconception is that NLP (Natural Language Processing) is a subfield of AI (Artificial Intelligence). While NLP is closely related to AI, it is not necessarily a part of it. AI encompasses a broad range of technologies and techniques aimed at creating intelligent systems, whereas NLP specifically focuses on the interaction between computers and human language.
- NLP is an essential component of AI systems.
- AI extends beyond language processing to include other cognitive abilities.
- NLP is a specialized field within computer science.
NLP vs AI
Another misconception is that NLP and AI are interchangeable terms. While AI can utilize NLP to enhance its language processing capabilities, it is important to understand that AI encompasses a wide range of subfields and techniques beyond NLP. NLP is just one aspect of AI that deals specifically with language understanding and generation.
- NLP focuses on language processing, whereas AI involves broader applications.
- AI includes other subfields such as machine learning and computer vision.
- NLP techniques can be applied in various AI applications, but they are not the only ones used.
NLP as a Subset of AI
Some people believe that NLP is a subset of AI since it involves teaching computers to understand and process human language. However, this perception fails to acknowledge that AI encompasses numerous other subfields that are not directly related to language processing. NLP may be an important component of AI, but it does not define the entire field.
- NLP is a specialized branch within AI, not its sole component.
- AI includes fields such as robotics, expert systems, and knowledge representation.
- NLP focuses specifically on language-related tasks within the broader scope of AI.
NLP’s Importance in AI
While NLP is not the entirety of AI, it holds significant importance within the field. NLP enables machines to understand, interpret, and generate human language, which is crucial for various AI applications such as virtual assistants, chatbots, and language translation systems. NLP techniques and algorithms play a vital role in improving the language capabilities of AI systems.
- NLP is essential for creating human-like interactions with AI systems.
- NLP enables AI systems to understand context and sentiment in human language.
- NLP advancements have led to significant improvements in AI language processing capabilities.
NLP and AI’s Intersection
Although NLP and AI are distinct fields, they do intersect and complement each other in many ways. NLP plays a crucial role in enhancing AI’s language understanding capabilities, allowing machines to process and generate text or speech. Simultaneously, AI techniques and technologies, such as machine learning and deep learning, have contributed significantly to advancements in NLP, enabling more sophisticated language processing algorithms.
- NLP and AI collaborate to improve language-based AI applications.
- AI technologies enhance the performance and accuracy of NLP algorithms.
- The intersection of NLP and AI leads to advancements in both fields.
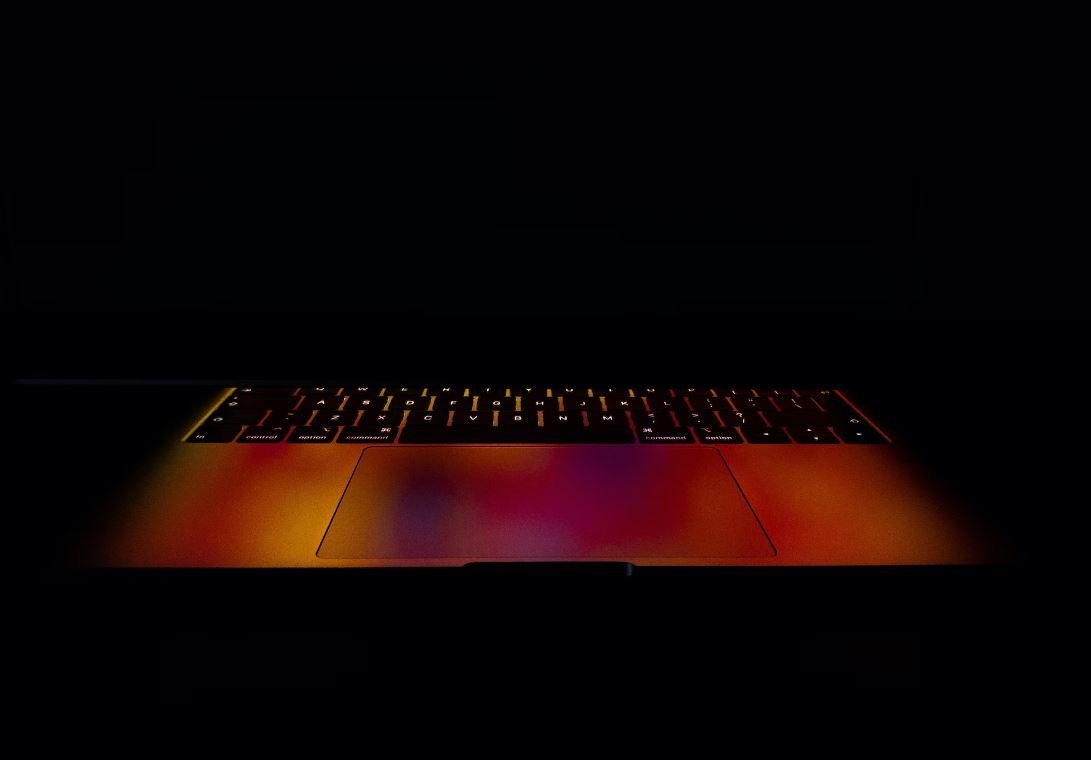
Is NLP Part of AI?
Natural Language Processing (NLP) is a field within Artificial Intelligence (AI) that focuses on the interaction between computers and human languages. It involves analyzing, understanding, and generating human language in a way that machines can comprehend. NLP plays a significant role in various AI applications, including chatbots, voice assistants, and language translation. Let’s explore some fascinating aspects of NLP and its connection to AI through the following tables:
Evolution of NLP
Table illustrating the major milestones in the evolution of Natural Language Processing:
| Year | Milestone |
|——|———————————————————|
| 1950 | Alan Turing proposed the “Turing Test” |
| 1956 | First NLP conference held at Dartmouth College |
| 1966 | ELIZA, the first chatbot developed at MIT |
| 1983 | Domain-specific language parsers introduced |
| 1990 | Statistical methods and machine learning applied to NLP |
| 2003 | Introduction of the widely-used NLTK library |
| 2011 | IBM’s Watson wins against human competitors on Jeopardy! |
| 2018 | Google’s BERT model achieves groundbreaking results |
| 2021 | GPT-3, an advanced language model, is released |
NLP Techniques
Table showcasing various techniques used in NLP:
| Technique | Description |
|——————————|——————————————————————————————————|
| Tokenization | Breaking text into individual units (words, characters, etc.) |
| Part-of-Speech Tagging | Assigning grammatical tags to words (noun, verb, adjective, etc.) |
| Named Entity Recognition | Identifying and classifying named entities (person, organization, date, etc.) |
| Sentiment Analysis | Determining the sentiment or emotion conveyed in a piece of text |
| Word Embeddings | Representing words as dense vectors to capture semantic relationships |
| Machine Translation | Translating text from one language to another |
| Question Answering Systems | Generating answers to questions based on a given context |
| Text Summarization | Generating concise summaries of longer pieces of text |
| Language Generation | Generating human-like language, often used for chatbots or text completion |
| Speech Recognition | Converting spoken language into written text |
NLP Applications
Table highlighting key applications of NLP across different industries:
| Industry | NLP Application |
|—————-|—————————————————————–|
| Healthcare | Electronic health record analysis, clinical decision support |
| Finance | Sentiment analysis in stock market predictions |
| Customer Service | Chatbots for automated customer support |
| E-commerce | Product description generation, sentiment-based recommendations |
| Education | Automated essay grading, intelligent tutoring systems |
| Marketing | Social media sentiment analysis, targeted advertising |
| Legal | Contract analysis, legal document classification |
| News | Automated news summarization, fake news detection |
| Transportation | Voice assistants for navigation and hands-free control |
| Entertainment | Content recommendation, sentiment analysis in movie reviews |
NLP Libraries and Frameworks
Table showcasing popular NLP libraries and frameworks:
| Library/Framework | Description |
|——————|——————————————————————————————————–|
| NLTK | Natural Language Toolkit for research and education purposes |
| SpaCy | Industrial-strength NLP library with an emphasis on efficiency |
| scikit-learn | Machine learning library with NLP capabilities for classification and clustering tasks |
| TensorFlow | Widely-used deep learning framework with NLP functionalities |
| PyTorch | Open-source deep learning framework extensively used for NLP tasks |
| Gensim | Robust library for topic modeling, document similarity, and text processing |
| Stanford NLP | Suite of NLP tools developed by Stanford University, including models for POS tagging and NER |
| Transformers | State-of-the-art library for pre-trained language models, such as BERT and GPT |
| CoreNLP | NLP toolkit offering a wide range of annotators and linguistic analysis capabilities |
| OpenNLP | Apache library for NLP tasks, including tokenization, POS tagging, and named entity recognition |
Common NLP Datasets
Table featuring widely-used datasets for NLP research and benchmarking:
| Dataset | Description |
|—————–|————————————————————————————————–|
| IMDB Reviews | Collection of movie reviews labeled as positive or negative sentiment |
| Reuters | News articles from Reuters labeled into categories like politics, sports, finance, etc. |
| Wikipedia | Multilingual corpus from Wikipedia covering various topics |
| Amazon Reviews | Reviews on different products from Amazon Marketplace |
| Twitter Sentiment | Tweets labeled with sentiment polarity (positive, negative, neutral) |
| SQuAD | Stanford Question Answering Dataset containing human-labeled questions and corresponding answers |
| GloVe | Pre-trained word vectors derived from a large corpus of Wikipedia |
| CoNLL | Shared Task datasets for tasks such as named entity recognition and dependency parsing |
| SNLI | Corpus of sentences labeled for entailment (whether one sentence implies another) |
| Enron Emails | Collection of emails from Enron Corporation (used for email data analysis tasks) |
NLP Performance Metrics
Table presenting commonly used performance metrics for NLP tasks:
| Metric | Description |
|———————-|—————————————————————|
| Accuracy | Proportion of correct predictions over the total predictions |
| Precision | Proportion of true positive predictions over all positive ones |
| Recall | Proportion of true positive predictions over actual positives |
| F1 Score | Harmonic mean of precision and recall |
| BLEU Score | Evaluation metric for machine translation quality |
| ROUGE Score | Evaluation metric for text summarization quality |
| Perplexity | Measure of language model’s predictive power |
| Word Error Rate (WER) | Measure of speech recognition system’s accuracy |
| Mean Average Precision (MAP) | Evaluation metric for information retrieval |
| Cohen’s Kappa | Agreement between multiple annotators on a classification task |
Key NLP Challenges
Table illustrating some challenges faced in NLP:
| Challenge | Description |
|———————————|———————————————————————–|
| Ambiguity | Resolving multiple interpretations or meanings of words or sentences |
| Named Entity Disambiguation | Identifying the correct entity when the same phrase refers to multiple entities |
| Data Sparsity | Inadequate data instances for certain languages or domains |
| Translation Ambiguity | Capturing the nuances and cultural references while translating |
| Sentiment Analysis Subjectivity | Accounting for subjectivity and context in sentiment analysis |
| Out-of-Domain Generalization | Extending NLP models’ performance to unseen domains or data types |
| Noise and Irregularities | Handling spelling errors, typos, abbreviations, slang, etc. |
| Ethics and Bias | Avoiding bias and ensuring ethical implications of NLP technologies |
| Low-Resource Languages | Limited resources and tools available for languages with fewer resources |
| Real-Time Processing | Efficiently processing and responding to human language in real-time |
NLP Research Institutions
Table highlighting renowned institutions involved in NLP research:
| Institution | Country |
|———————|——————|
| Carnegie Mellon | United States |
| Stanford University | United States |
| University of Cambridge | United Kingdom |
| University of Oxford | United Kingdom |
| Massachusetts Institute of Technology (MIT) | United States |
| University of California, Berkeley | United States |
| University of Washington | United States |
| Google Research | Multi-national |
| OpenAI | Multi-national |
| Facebook AI Research | Multi-national |
Current NLP Trends
Table outlining some prevailing trends within the field of Natural Language Processing:
| Trend | Description |
|———————|—————————————————————–|
| Transformer Models | Dominating NLP with their ability to capture long-range dependencies |
| Pre-trained Models | Utilizing large-scale pre-trained models as a starting point for NLP tasks |
| Low-Resource Learning | Improving NLP models’ performance in languages with limited resources |
| Multimodal NLP | Integrating NLP with other modalities like vision and audio |
| Ethical NLP | Addressing biases, fairness, and responsible AI practices in NLP |
| Multilingual NLP | Extending NLP models to support multiple languages |
| Conversational AI | Developing chatbots and voice assistants with enhanced conversational capabilities |
| Explainable NLP | Ensuring transparency and interpretability of NLP models |
| Few-shot Learning | Enabling NLP models to learn effectively with limited training examples |
| Cross-lingual Transfer | Transferring knowledge from one language to another to boost performance |
NLP Challenges and Opportunities
Table outlining the challenges and opportunities within NLP:
| | Challenges | Opportunities |
|———————–|—————————————|————————————————————-|
| Technical | Ambiguity, data sparsity, and noise | Advanced deep learning models and improved data collections |
| Social and Ethical | Bias detection and ethical implications | Responsible AI development and unbiased systems |
| Commercial | High competition and scalability | Exploring new applications and market niches |
| Research and Academia | Limited resources and infrastructure | Collaborative research efforts and industry partnerships |
| User Experience | Effective communication with machines | Enhanced interaction models and natural language interfaces |
In summary, Natural Language Processing (NLP) is an integral part of Artificial Intelligence (AI) and plays a crucial role in bridging the gap between human language and machine understanding. With advancements in language models, increasing availability of datasets, and emerging trends, NLP continues to open up exciting possibilities in various industries and research domains.
Frequently Asked Questions
Is NLP Part of AI?
What is NLP?
NLP stands for Natural Language Processing. It is a field of study within artificial intelligence (AI) that focuses on the interaction between computers and human language.
How does NLP work?
NLP uses algorithms and techniques to analyze, understand, and interpret natural language data. It involves tasks such as text classification, sentiment analysis, named entity recognition, and language translation.
Why is NLP important in AI?
NLP plays a crucial role in AI as it enables machines to understand and interact with human language. It allows AI systems to process and derive meaning from text or speech, enabling tasks like chatbots, voice assistants, language translation, information retrieval, and much more.
What are some applications of NLP?
NLP has various applications such as sentiment analysis, language translation, chatbots, virtual assistants, speech recognition, text summarization, information extraction, and document classification.
Is NLP the same as AI?
No, NLP is a part of AI. While AI is a broad field encompassing various disciplines like machine learning, computer vision, robotics, and more, NLP specifically deals with the processing and understanding of human language using AI techniques.
What are the challenges in NLP?
NLP faces challenges such as language ambiguity, understanding context and sarcasm, working with multiple languages, handling noisy or incomplete data, and ensuring privacy and ethics in language processing.
What are some popular NLP frameworks and tools?
There are popular NLP frameworks and tools available such as NLTK (Natural Language Toolkit), SpaCy, Gensim, Stanford NLP, Hugging Face’s Transformers, and Google’s BERT (Bidirectional Encoder Representations from Transformers).
Can NLP understand all languages?
NLP can work with multiple languages, but the level of understanding varies. Different languages have diverse grammatical structures, idioms, and nuances that require language-specific processing techniques and resources.
Is NLP useful in data analysis?
Absolutely. NLP techniques can be applied to analyze large volumes of unstructured text data, extract valuable insights, perform sentiment analysis, detect patterns, categorize documents, and aid in decision-making processes.
What is the future of NLP?
The future of NLP looks promising. Advancements in deep learning, neural networks, and language models have significantly improved NLP capabilities. It is expected that NLP will continue to evolve, enabling more sophisticated language understanding, generation, and human-like interactions with AI systems.