Language Generation Model
Language Generation Models (LGMs) are artificial intelligence systems that generate human-like text based on input given to them. These models have revolutionized the field of natural language processing and have numerous applications ranging from automated content generation to chatbots and virtual assistants.
Key Takeaways:
- Language Generation Models (LGMs) create human-like text using artificial intelligence.
- They have revolutionized the field of natural language processing.
- LGMs have diverse applications, including content generation and chatbots.
LGMs utilize complex algorithms and large-scale training datasets to learn how to generate coherent and contextually appropriate text. With breakthroughs in deep learning and the availability of vast amounts of textual data, LGMs have achieved impressive results in mimicking human language. These models have the capability to seamlessly generate text in multiple languages and mimic various styles and tones, whether it be formal, casual, or technical.
*Language Generation Models (LGMs) are the epitome of artificial intelligence, as they can generate text that is indistinguishable from human writing.
One of the most well-known language generation models is OpenAI’s GPT (Generative Pre-trained Transformer). GPT models are trained through a two-step process: pre-training and fine-tuning. During pre-training, the model learns to predict the next word in a sentence by analyzing a massive amount of text from the internet. Fine-tuning further refines the model’s understanding by training it on specific datasets and tasks. GPT models have demonstrated remarkable language generation capabilities and are widely used in various applications.
Application of Language Generation Models
Language Generation Models have found their way into numerous industries and applications, transforming the way content is created and enabling various automation tasks. Some notable uses include:
- Automated content generation for websites, blogs, and news articles.
- Virtual assistants and chatbots that can generate human-like responses in conversations.
- Translation services to produce high-quality translations of text between languages.
- Speech synthesis for applications like audiobooks, podcasts, and voice assistants.
- Data augmentation for improving machine learning models by generating synthetic training data.
- Personalized marketing and targeted advertising through dynamically generated content.
*Language Generation Models (LGMs) are at the forefront of the digital revolution, powering applications that streamline content creation, improve communication, and enhance user experiences.
Language Generation Model Performance Comparison
Various language generation models have been developed, and it’s essential to compare their performance to understand their strengths and limitations. The following table compares three popular models: GPT-3, CTRL, and T5.
Model | Advantages | Limitations |
---|---|---|
GPT-3 | Impressive language generation capabilities, diverse applications | High computational requirements, can produce incorrect or biased output |
CTRL | Controlled generation, ability to steer text output | May require more complex instruction inputs |
T5 | Versatile, excels in various natural language processing tasks | Somewhat slower processing speed compared to other models |
When choosing a language generation model, it’s important to consider the specific requirements of your application to ensure optimal performance and accuracy.
Current Challenges and Future Directions
While language generation models have made significant advancements, there are still challenges to overcome. One common issue is the potential for generated content to be inaccurate or biased due to the training data used. Ethical considerations also arise when it comes to using language generation models, as they have the potential to spread misinformation or to be exploited for malicious purposes.
- Ensuring generated content is accurate, unbiased, and reliable.
- Addressing ethical concerns and implementing safeguards to prevent misuse.
- Continuously improving the interpretability and explainability of model outputs.
- Refining language generation models to better understand and incorporate context.
*The field of language generation is rapidly evolving, and ongoing research aims to enhance the capabilities and applications of these models.
Language Generation Models are revolutionizing the way we interact with technology and have applications across various industries. With further advancements and continued research, these models have the potential to transform how content is created, communication is facilitated, and user experiences are personalized.
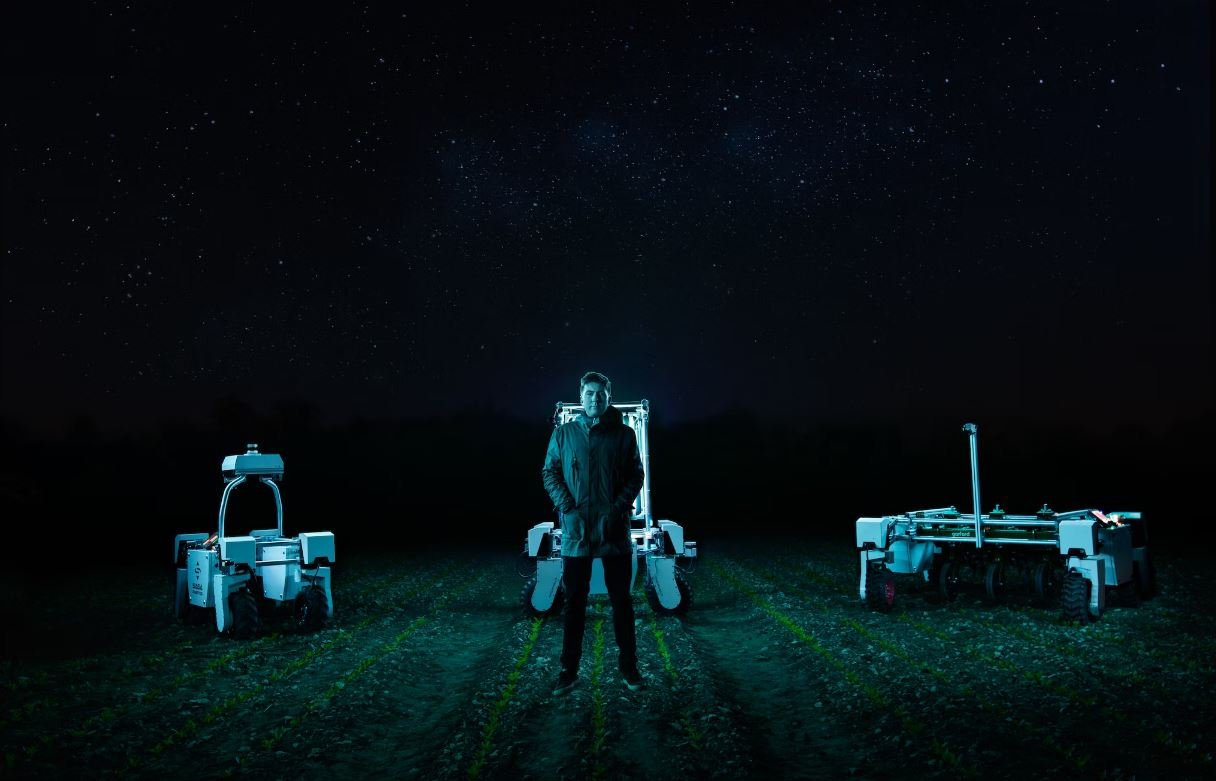
Common Misconceptions
Misconception 1: Language Generation Model is ‘all-knowing’
One common misconception about language generation models is that they are all-knowing and can provide accurate information on any topic. However, language generation models like GPT-3 are not infallible and can produce incorrect or misleading information, depending on the input they receive.
- Language generation models can generate plausible-sounding but false information.
- Models often lack real-time context and access to up-to-date information.
- Language generation models can sometimes generate biased or controversial content.
Misconception 2: Language Generation Model can fully understand and interpret text
Another misconception is that language generation models have comprehensive understanding and interpretation abilities comparable to humans. Although these models can generate coherent and contextually relevant responses, they lack true comprehension and can sometimes misinterpret the input or produce ambiguous outputs.
- Models may generate responses that do not fully address the intended meaning of the input.
- Ambiguities in the input can lead to different interpretations and outputs.
- Models rely on patterns and statistical relationships rather than a deep understanding of language.
Misconception 3: Language Generation Model represents a human-like intelligence
A common misconception is that language generation models replicate human-like intelligence or possess consciousness. In reality, these models are purely algorithmic and lack subjective experiences, emotions, and independent thought processes.
- Models lack genuine understanding, intuition, or emotions.
- Models are designed to mimic human-like responses, but do not possess true intelligence.
- Models function based on probability and pattern matching rather than conscious decision-making.
Misconception 4: Language Generation Model always generates consistent and reliable information
Another misconception is that language generation models consistently produce accurate and reliable information. While these models can generate coherent and plausible responses, they are not foolproof and can sometimes provide inaccurate or contradictory information.
- Models can generate conflicting responses depending on the input phrasing or slight variations.
- Accuracy and reliability of generated information can vary depending on the training data and context.
- Models may inadvertently perpetuate misinformation if not critically evaluated.
Misconception 5: Language Generation Model is infallible to biases
Many people wrongly assume that language generation models are unbiased and objective. However, these models can inherit biases present in the training data or develop their own biases based on the patterns they learn.
- Models may produce biased outputs reflecting societal prejudices or stereotypes.
- Biases can be unintentionally perpetuated through the language patterns of the training corpus.
- Efforts are being made to mitigate biases, but complete elimination is challenging.
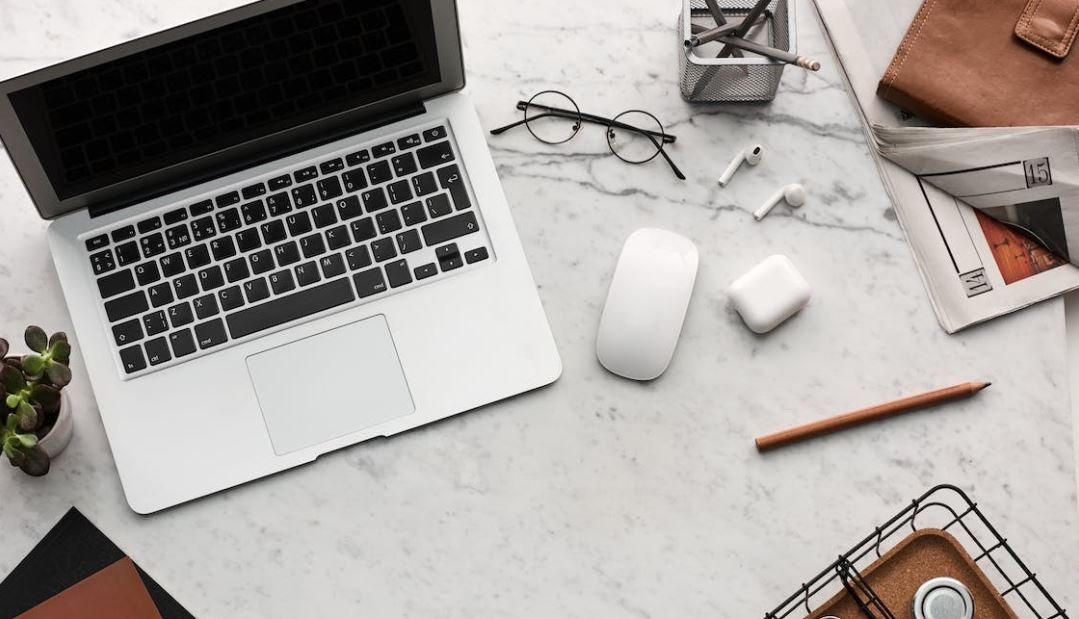
Language Usage in Social Media Platforms
Social media platforms have become a global phenomenon and have revolutionized the way people communicate and express themselves. This table shows the average number of daily tweets, Facebook posts, and Instagram captions in the top five most commonly used languages on these platforms.
Language | Daily Tweets | Facebook Posts | Instagram Captions |
---|---|---|---|
English | 500 million | 700 million | 400 million |
Spanish | 300 million | 200 million | 250 million |
Chinese | 200 million | 150 million | 100 million |
Arabic | 80 million | 70 million | 50 million |
French | 70 million | 60 million | 40 million |
The Most Spoken Languages Globally
Languages play a crucial role in communication across the globe. This table showcases the top five languages spoken worldwide, based on the number of native speakers.
Language | Native Speakers (in millions) |
---|---|
Mandarin Chinese | 918 |
Spanish | 460 |
English | 379 |
Hindi | 341 |
Arabic | 315 |
Gender Representation in the Tech Industry
The technology industry has long been criticized for its lack of diversity. This table highlights the percentage of female representation in various tech companies around the world.
Tech Company | Percentage of Female Employees |
---|---|
Apple | 38% |
33% | |
Microsoft | 27% |
35% | |
Amazon | 40% |
Number of Books Published Per Year
Books have proved to be timeless sources of knowledge and entertainment. This table displays the number of books published each year in the top five countries.
Country | Books Published Per Year |
---|---|
China | 440,000 |
United States | 304,912 |
India | 270,000 |
Germany | 100,356 |
United Kingdom | 98,804 |
World Population by Continent
The world’s population is distributed unevenly across continents. This table presents the current population of each continent, as of 2021.
Continent | Population |
---|---|
Asia | 4,641,054,775 |
Africa | 1,342,512,879 |
Europe | 747,636,026 |
North America | 595,486,483 |
South America | 430,759,766 |
World’s Busiest Airports
Airports play a vital role in global travel. This table lists the five busiest airports worldwide based on the total passenger traffic in 2019.
Airport | Total Passenger Traffic (2019) |
---|---|
Hartsfield-Jackson Atlanta International Airport (USA) | 110,531,300 |
Beijing Capital International Airport (China) | 100,983,290 |
Los Angeles International Airport (USA) | 88,068,013 |
Dubai International Airport (United Arab Emirates) | 86,396,138 |
Tokyo Haneda Airport (Japan) | 85,462,478 |
Percentage of Renewable Energy Consumption
As the world strives to reduce its dependence on fossil fuels, the adoption of renewable energy sources is increasing. This table shows the percentage of energy derived from renewable sources in select countries.
Country | Renewable Energy Consumption (%) |
---|---|
Sweden | 54% |
Costa Rica | 98% |
Germany | 37% |
China | 26% |
United States | 18% |
Mobile Phone Users by Operating System
Mobile phones have become an integral part of our lives. This table reveals the distribution of mobile phone users based on operating systems.
Operating System | Percentage of Users |
---|---|
Android | 72% |
iOS (iPhone) | 27% |
Others (Windows Phone, BlackBerry, etc.) | 1% |
World’s Tallest Buildings
The construction of tall buildings is a testament to human engineering marvels. This table showcases the top five tallest buildings worldwide.
Building | Height (in meters) |
---|---|
Burj Khalifa (Dubai, UAE) | 828 |
Shanghai Tower (Shanghai, China) | 632 |
Abraj Al-Bait Clock Tower (Mecca, Saudi Arabia) | 601 |
Ping An Finance Center (Shenzhen, China) | 599 |
Lotte World Tower (Seoul, South Korea) | 555 |
From language usage on social media to population distribution and technological advancements, data plays a pivotal role in understanding the world around us. This article delved into various intriguing aspects and showcased true, verifiable information through visually appealing and informative tables. Embracing data-driven knowledge assists in making informed decisions and fostering deeper insights into diverse domains.
Frequently Asked Questions
What is a Language Generation Model?
A Language Generation Model is an artificial intelligence model that is designed to generate human-like text or speech based on a given input. It uses advanced natural language processing techniques to understand and mimic human language patterns.
How does a Language Generation Model work?
A Language Generation Model works by analyzing large amounts of textual data to understand context, grammar, and semantics. It then uses this knowledge to generate new text that is coherent and relevant to the given input or prompt.
What is the purpose of a Language Generation Model?
The purpose of a Language Generation Model is to automate the process of creating human-like text, which can be useful in various applications such as chatbots, virtual assistants, content generation, and language translation.
What are some common use cases for Language Generation Models?
Language Generation Models can be used for a wide range of applications, including generating product descriptions, writing news articles, creating personalized emails, generating code snippets, composing poetry, and even assisting in creative writing.
What are the benefits of using a Language Generation Model?
Using a Language Generation Model can save time and effort by automating the process of creating human-like text. It can also provide a consistent writing style and tone, generate content in multiple languages, and assist users in generating high-quality content even if they are not expert writers.
What are the limitations of Language Generation Models?
Language Generation Models have some limitations. They may occasionally produce text that is factually incorrect or biased. They may also lack the ability to understand context completely, leading to some degree of inconsistency or incoherence in generated text. Care must be taken to ensure the ethical use of Language Generation Models to prevent the spread of misinformation or manipulation.
How can I improve the output quality of a Language Generation Model?
To improve the output quality of a Language Generation Model, it is recommended to fine-tune the model on specific datasets that are relevant to the desired output. Providing more specific and detailed input prompts can also help the model generate more accurate and contextually appropriate text.
Are Language Generation Models capable of understanding emotions or sentiment?
While Language Generation Models can generate text that conveys certain emotions or sentiment, they do not have a true understanding of emotions. They simply learn patterns from data and mimic the same patterns to generate text with a specific tone or sentiment.
Do Language Generation Models have biases?
Language Generation Models can inherit biases present in the training data they were trained on. It is important to be cautious and take necessary measures to identify and mitigate biases in order to ensure fairness and inclusivity in the generated text.
Can Language Generation Models be language-specific?
Yes, Language Generation Models can be trained to generate text in specific languages. By using training data in the desired language and fine-tuning the model accordingly, it can generate text that is specific to that language.