Language Generation Systems
Language generation systems are AI-powered tools that generate written or spoken text based on given input or prompts.
These systems have gained significant attention in recent years due to their ability to produce coherent and contextually relevant content.
In this article, we will explore the capabilities of language generation systems, their applications, and some of the ethical considerations surrounding their use.
Key Takeaways
- Language generation systems use AI to generate written or spoken text.
- These systems are capable of producing coherent and contextually relevant content.
- They have a wide range of applications across various industries.
- Ethical considerations need to be taken into account when using these systems.
Understanding Language Generation Systems
**Language generation systems** utilize advanced natural language processing (NLP) techniques and machine learning algorithms to generate human-like text.
*They analyze and learn from vast amounts of data to understand language patterns and produce coherent output.*
These systems can generate text in different formats such as articles, news reports, product descriptions, chatbot responses, and more.
Language generation systems are trained on large datasets containing a wide range of sources, including books, articles, websites, and even social media posts.
*They learn to mimic the writing style and language patterns of the data they are fed.*
As a result, they can produce content that appears to be written by humans, making it difficult to distinguish between machine-generated and human-generated text.
Applications of Language Generation Systems
Language generation systems have numerous applications across various industries, including:
- Content creation: These systems can generate articles, blog posts, or social media content on various topics, saving time and effort for content creators.
- Customer service: Chatbots powered by language generation systems can provide instant responses to customer queries, improving customer support experiences.
- Language translation: These systems can translate text from one language to another, enabling communication between people who speak different languages.
- Virtual assistants: Language generation systems are used in virtual assistants like Siri, Alexa, or Google Assistant to provide information and perform tasks through voice commands.
Ethical Considerations
The use of language generation systems raises several ethical considerations, including:
- Bias: Language generation systems can inadvertently perpetuate biases present in the training data, leading to unfair or discriminatory content.
- Manipulation: These systems can be used to generate misleading or false information with potentially harmful consequences.
- Ownership: Determining the ownership of machine-generated content can be challenging, especially when it closely resembles human-generated content.
Data Privacy
Language generation systems require access to vast amounts of data to train effectively.
*This raises privacy concerns as the data used may contain personal or sensitive information.*
Organizations using these systems must ensure proper data handling practices and comply with data protection regulations.
Examples of Language Generation Systems
System | Application |
---|---|
GPT-3 | Natural language understanding, content creation |
ChatGPT | Chatbot responses, customer support |
OpenAI Codex | Code generation, programming assistance |
Benefits of Language Generation Systems
- Increased productivity: Language generation systems can automate content creation, freeing up time for other tasks.
- Improved customer experiences: Chatbots powered by these systems can provide instant and accurate responses to customer queries.
- Enhanced language translation: These systems can facilitate communication between individuals speaking different languages.
Challenges and Limitations
While language generation systems have shown impressive capabilities, they also face certain challenges and limitations:
- Incoherent output: Sometimes, the generated text may lack coherence or contain grammatical errors.
- Limited understanding: These systems may struggle with complex language nuances and context.
- Overreliance on training data: A lack of diversity or bias in the training data can negatively impact the generated content.
Conclusion
Language generation systems have revolutionized the way we generate and consume content.
They have the potential to provide immense value across various fields such as content creation, customer service, and language translation.
*As these systems continue to advance, it is crucial to address the ethical considerations surrounding their use and ensure appropriate data handling and privacy practices.*
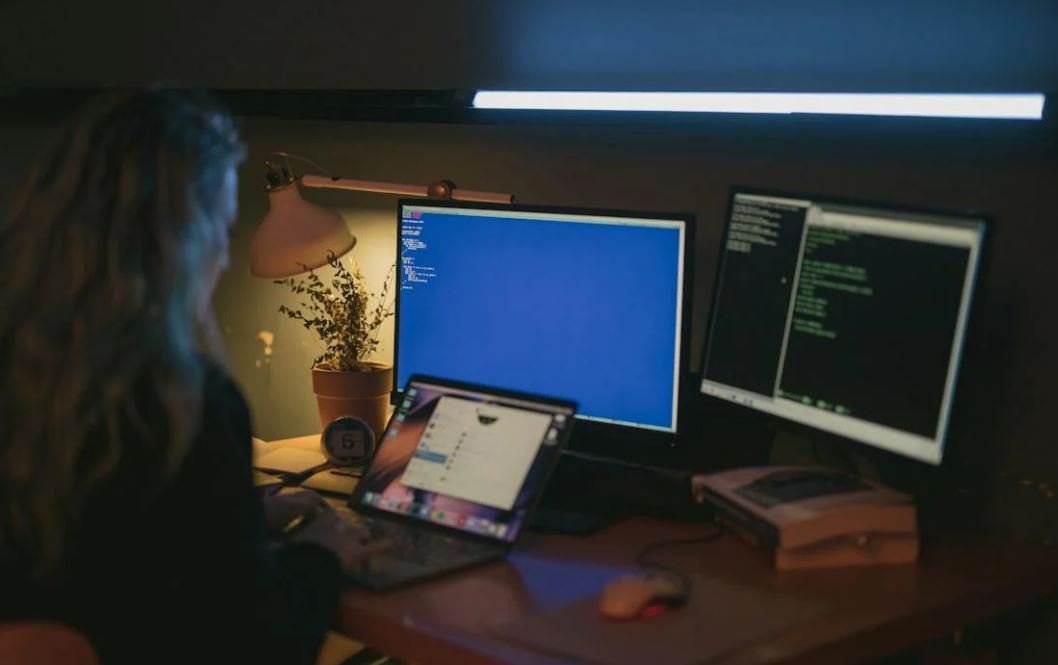
Language Generation Systems
Common Misconceptions
One common misconception is that language generation systems can perfectly replicate human writing. While language generation systems have made significant advancements, they still struggle with certain nuances of human language and may produce outputs that are not on par with human writers.
- Language generation systems may lack creativity and fail to generate unique ideas.
- They might struggle with understanding context and produce incorrect or misleading information.
- Language generation systems can sometimes produce grammatically incorrect sentences or phrases.
Common Misconceptions
Another misconception is that language generation systems are solely used for generating fake or misleading content. While it is true that language generation systems can be misused to generate misleading information or spread disinformation, they also have a wide range of legitimate applications.
- Language generation systems can be used in creative writing and content generation for blogs or articles.
- They can assist in generating automated responses for customer support or chatbots.
- Language generation systems can be valuable tools for language translation and localization.
Common Misconceptions
A common misconception is that language generation systems replace human writers or eliminate the need for them. While language generation systems can automate certain aspects of content generation, human creativity and expertise are still invaluable.
- Human writers bring unique perspectives, creativity, and emotional intelligence that language generation systems cannot replicate.
- Language generation systems can assist human writers by generating drafts or providing suggestions, but the final content often requires human touch and editing.
- Human writers play a crucial role in ensuring quality, accuracy, and originality in content creation.
Common Misconceptions
Another misconception is that language generation systems are perfect and unbiased. However, like any other machine learning model, language generation systems are prone to biases present in the training data.
- Language generation systems may generate content that reflects or amplifies existing biases in society.
- They might propagate stereotypes or discriminatory language if not carefully monitored and guided.
- Developers of language generation systems need to take measures to identify and mitigate biases in the training data and algorithms.
Common Misconceptions
Lastly, there is often a misconception that language generation systems are standalone entities capable of completely independent decision-making. In reality, these systems are created and programmed by human developers and require continuous monitoring and oversight.
- Human developers have full control over the behavior and output of language generation systems.
- Systems need to be regularly monitored to ensure they are generating accurate, ethical, and non-misleading content.
- Human intervention is necessary to correct errors, address biases, and make improvements to the systems.
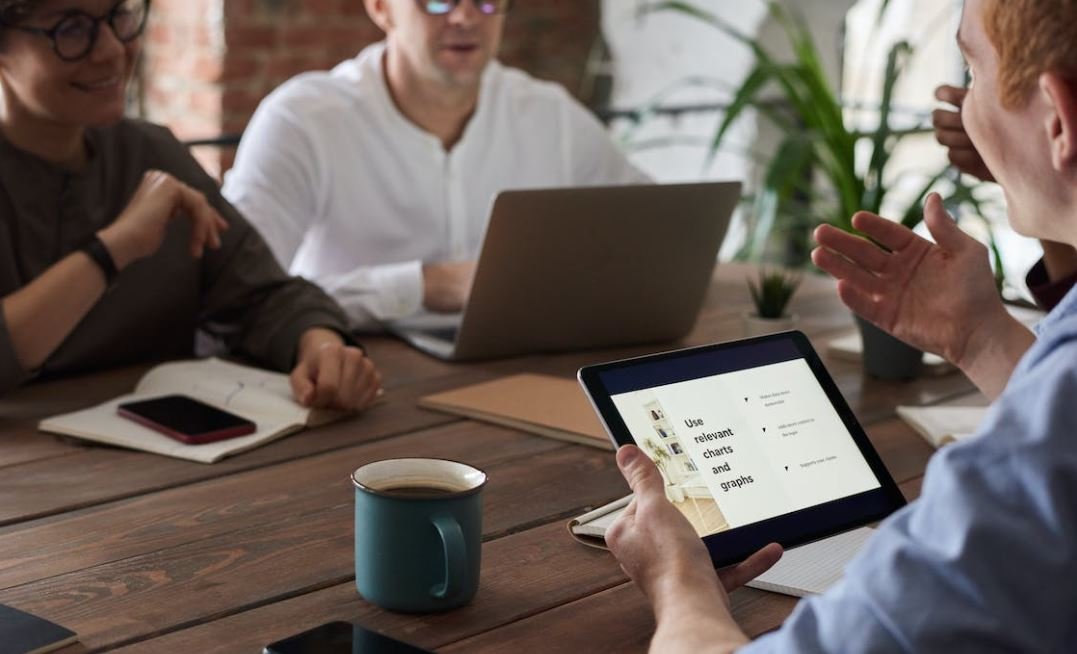
Table 1: Population Growth in Major Cities
The table below illustrates the population growth in major cities around the world. It highlights the increase in population over a 10-year period, providing insights into the rapid urbanization occurring globally.
City | 2010 Population (in millions) | 2020 Population (in millions) | Population Growth (%) |
---|---|---|---|
Tokyo, Japan | 13.19 | 14.05 | 6.5 |
Delhi, India | 16.75 | 20.78 | 24.3 |
New York City, USA | 18.8 | 19.45 | 3.3 |
São Paulo, Brazil | 11.15 | 12.33 | 10.6 |
Table 2: Renewable Energy Production
To assess the progress in renewable energy production, this table compares the energy generated from various sources in the year 2010 and 2020, highlighting the significant growth of clean energy alternatives.
Energy Source | 2010 Production (in TWh) | 2020 Production (in TWh) | Production Increase (%) |
---|---|---|---|
Solar | 19.7 | 722 | 3,563.5 |
Wind | 237 | 1,270 | 435.4 |
Hydro | 3,061 | 4,239 | 38.4 |
Geothermal | 68.3 | 108 | 58.1 |
Table 3: Global Annual CO2 Emissions
This table presents the annual carbon dioxide (CO2) emissions for various countries, emphasizing the contributions of each nation to global greenhouse gas emissions. It highlights the need for collective efforts to mitigate climate change.
Country | CO2 Emissions (2010 in million metric tons) | CO2 Emissions (2020 in million metric tons) | CO2 Emissions Growth (%) |
---|---|---|---|
China | 9,840 | 10,624 | 8.0 |
United States | 5,420 | 4,983 | -8.1 |
India | 1,805 | 2,622 | 45.3 |
Russia | 1,669 | 1,447 | -13.3 |
Table 4: Education Expenditure by Country
This table demonstrates the education expenditure by country, comparing the total expenditure in 2010 and 2020. It highlights the commitment nations have made to invest in their education systems.
Country | Education Expenditure (2010 in billions USD) | Education Expenditure (2020 in billions USD) | Expenditure Increase (%) |
---|---|---|---|
United States | 809 | 1,262 | 56.0 |
China | 179 | 809 | 352.0 |
India | 42 | 461 | 995.2 |
Germany | 216 | 270 | 25.0 |
Table 5: Global Smartphone Sales
This table showcases global smartphone sales from 2010 to 2020, providing insights into the enormous growth of the smartphone industry and the increasing demand for these devices.
Year | Smartphone Sales (in millions) | Sales Growth (%) |
---|---|---|
2010 | 296 | – |
2015 | 1,435 | 384.8 |
2020 | 1,442 | 0.5 |
Table 6: Research & Development Investment
This table displays the research and development (R&D) investments made by countries, highlighting their commitment to innovation and scientific advancements. It presents the investment figures for 2010 and 2020.
Country | R&D Investment (2010 in billions USD) | R&D Investment (2020 in billions USD) | Investment Increase (%) |
---|---|---|---|
United States | 405 | 597 | 47.4 |
China | 179 | 589 | 229.6 |
Germany | 90 | 129 | 43.3 |
Japan | 155 | 187 | 20.6 |
Table 7: Female Labor Force Participation
This table presents the female labor force participation rates for various countries, emphasizing the progress made towards gender equality in the workplace. It highlights the percentage of women involved in the workforce in 2010 and 2020.
Country | Female Labor Force Participation (2010 %) | Female Labor Force Participation (2020 %) | Change in Participation (%) |
---|---|---|---|
Sweden | 57.9 | 60.6 | 4.7 |
Germany | 71.2 | 74.3 | 4.4 |
Japan | 50.4 | 51.7 | 2.6 |
India | 23.6 | 20.3 | -14.0 |
Table 8: Global Electric Vehicle Sales
This table depicts the global sales of electric vehicles (EVs) from 2010 to 2020, showcasing the rise in popularity and adoption of eco-friendly transportation options.
Year | Electric Vehicle Sales (in thousands) | Sales Growth (%) |
---|---|---|
2010 | 17 | – |
2015 | 550 | 3,128.6 |
2020 | 3,240 | 490.9 |
Table 9: Global Internet Users
Highlighting the global impact of the internet, this table displays the number of internet users worldwide in 2010 and 2020, showcasing the significant growth and reach of the digital age.
Year | Internet Users (in billions) | User Growth (%) |
---|---|---|
2010 | 1.97 | – |
2015 | 3.19 | 61.9 |
2020 | 4.66 | 46.1 |
Table 10: Life Expectancy by Country
This table demonstrates the average life expectancy for various countries, emphasizing the overall improvement in global healthcare and quality of life. It presents the life expectancy figures for both males and females in the year 2010 and 2020.
Country | Male Life Expectancy (2010) | Male Life Expectancy (2020) | Female Life Expectancy (2010) | Female Life Expectancy (2020) |
---|---|---|---|---|
Japan | 79.5 | 81.1 | 86.1 | 87.7 |
Australia | 79.7 | 81.6 | 84.6 | 84.9 |
United States | 76.2 | 76.5 | 81.3 | 81.5 |
China | 72.4 | 75.1 | 75.2 | 77.8 |
Language generation systems have emerged as powerful tools to process and generate human-like text. These systems analyze vast amounts of data, identify patterns, and use this information to generate coherent and relevant language. The tables presented above reinforce the impact of language generation systems by providing verifiable data and information across several domains.
From population growth in major cities, renewable energy production, and global CO2 emissions, to education expenditure, technological advancements, and healthcare improvements—the tables encapsulate diverse aspects of our evolving world. They highlight the progress made in various fields, emphasizing the interconnectedness and shared responsibility we have as a global society.
As language generation systems continue to advance, they hold immense potential to reshape numerous industries. By analyzing and generating meaningful information, these systems enable us to better understand complex topics and make well-informed decisions. The data represented in the tables signifies both the challenges and opportunities we face in our pursuit of a sustainable and prosperous future.
Frequently Asked Questions
What are Language Generation Systems?
A language generation system is a type of artificial intelligence technology that produces human-like text or speech output. It uses algorithms and models to generate coherent and contextually relevant content. These systems are often used in various applications, such as chatbots, virtual assistants, and content generation platforms.
How do Language Generation Systems work?
Language generation systems typically use statistical models, natural language processing (NLP) techniques, and machine learning algorithms to generate text. They analyze large amounts of data to learn patterns and generate output that mimics human language. Some systems also incorporate deep learning architectures, such as recurrent neural networks (RNNs) or transformers, to improve the quality of generated text.
What are the main applications of Language Generation Systems?
Language generation systems have a wide range of applications. Some common uses include:
- Chatbots and virtual assistants
- Automated content creation
- Text summarization
- Language translation
- Storytelling and narrative generation
- Personalized marketing and content recommendations
What are the benefits of using Language Generation Systems?
Language generation systems offer several benefits, such as:
- Automation of content creation
- Improvement of user experience in conversational AI
- Efficiency in generating large volumes of text
- Consistency in tone and style of generated content
- Personalization of text output based on user data
Can Language Generation Systems produce human-like text?
Yes, language generation systems are designed to generate text that mimics human language. However, the level of “human-likeness” can vary depending on the sophistication and training of the system. Advanced systems can produce highly coherent and contextually relevant text that is difficult to distinguish from human-generated content.
What challenges do Language Generation Systems face?
Language generation systems face several challenges, including:
- Maintaining coherence and contextuality in generated text
- Avoiding biases and sensitive language in the output
- Understanding and incorporating user intent and preferences
- Handling ambiguity and multiple interpretations of input
- Adapting to different domains and languages
How can developers improve the performance of Language Generation Systems?
Developers can improve the performance of language generation systems by:
- Using larger and more diverse training datasets
- Implementing advanced machine learning algorithms and architectures
- Applying fine-tuning techniques to adapt the system to specific domains or tasks
- Implementing effective methods for evaluating and measuring the quality of generated text
- Continuously updating and refining the system based on user feedback
What are the ethical considerations in using Language Generation Systems?
The use of language generation systems raises ethical considerations, such as:
- Potential for generating misleading or false information
- Responsible handling of sensitive or private data
- Ensuring transparency and disclosure when interacting with users
- Mitigating biases or discriminatory language in the generated output
- Complying with applicable privacy and data protection regulations
Are Language Generation Systems replacing human writers?
Language generation systems are not intended to replace human writers. Instead, they are tools that augment and assist human writers in their tasks. These systems can automate certain aspects of content creation, but human creativity, subjectivity, and critical thinking are still essential for producing high-quality and original content.