Language Generation Using Machine Learning
Language generation using machine learning is a fascinating field that has gained significant attention in recent years. Combining the powers of artificial intelligence and linguistic analysis, machine learning algorithms have the ability to generate coherent and contextually relevant language. This article explores the key concepts behind language generation using machine learning and its practical applications.
Key Takeaways:
- Language generation using machine learning combines AI and linguistic analysis.
- Machine learning algorithms can generate coherent and contextually relevant language.
- Applications include chatbots, document summarization, and creative writing assistance.
Machine learning algorithms are trained on vast amounts of text data to learn patterns and structures of language. By analyzing the relationships between words, phrases, and sentences, these algorithms can generate new text that adheres to the learned patterns. This process involves understanding grammar, syntax, and semantics, allowing the algorithm to produce human-like language effortlessly. *Machine learning algorithms can generate text that is indistinguishable from human-written content, revolutionizing the way we interact with AI systems.*
Language generation using machine learning finds applications in a wide range of fields. One prominent example is chatbot development. Chatbots can provide instant responses to user queries, acting as virtual assistants in customer service or other domains. Additionally, machine learning-powered language generation is used in document summarization, where lengthy texts are condensed into concise summaries. Creative writing assistance is another intriguing application area, as algorithms can generate suggestions and ideas based on existing content. *By leveraging machine learning, language generation opens up new possibilities for automating various tasks and enhancing efficiency.*
Table 1: Example Use Cases for Language Generation
Use Case | Description |
---|---|
Chatbots | Virtual assistants that provide instant responses to user queries. |
Document Summarization | Condensing lengthy texts into concise summaries. |
Creative Writing Assistance | Algorithms generating suggestions and ideas for writers. |
Machine learning algorithms can generate language that mimics different styles and genres. By training on specific datasets, the algorithms can produce text that resembles the patterns found in a particular genre, such as news articles or scientific papers. This flexibility allows for diverse applications where machine-generated language needs to match a specific context. *From imitating Shakespearean prose to mimicking scientific publications, machine learning can generate text in a manner consistent with given styles.*
Table 2: Styles and Genres Emulated by Machine Learning Algorithms
Style/Genre | Description |
---|---|
News Articles | Text resembling the structure and content of news articles. |
Scientific Papers | Generating text with a scientific tone and structure. |
Literary Fiction | Creating narratives and prose in various fictional styles. |
Language generation using machine learning is not without its challenges. Ensuring generated text is contextually coherent, avoiding bias, and maintaining ethical standards are ongoing concerns. Researchers and developers continually work to improve these algorithms and address these issues accordingly. *The quest to achieve truly human-like language generation remains a constant driving force in the field of machine learning.*
Table 3: Challenges in Language Generation Using Machine Learning
Challenge | Description |
---|---|
Contextual Coherence | Ensuring generated text remains contextually relevant and coherent. |
Bias and Prejudice | Avoiding perpetuation of bias or prejudice in the generated text. |
Ethical Standards | Maintaining ethical considerations in language generation. |
Language generation using machine learning is a rapidly evolving field with numerous applications and exciting possibilities. As researchers continue to refine algorithms and overcome challenges, we can expect further advancements in natural language processing and AI systems. *The future of language generation holds immense potential to transform how we produce, consume, and interact with written content.*

Common Misconceptions
When it comes to language generation using machine learning, there are several common misconceptions that people often have. Let’s take a closer look at some of these misconceptions.
Misconception 1: Machines can think and understand language like humans
- Machines are not capable of true understanding or consciousness.
- Machine learning models process language based on statistical patterns, not true comprehension.
- Machines lack human context and common sense knowledge.
Misconception 2: Machine-generated text is always perfect and error-free
- Machine learning models are not perfect and can generate incorrect or nonsensical text.
- Errors can occur due to biased training data or limitations in the model architecture.
- Machine-generated text often requires human supervision and editing for accuracy and coherence.
Misconception 3: Language generation will replace human writers
- Machine-generated text is currently limited to specific domains or purposes.
- Human creativity, intuition, and unique perspectives cannot be replicated by machines.
- Language generation tools often assist human writers rather than replace them.
Misconception 4: Machine-generated language is always ethical and unbiased
- Machine learning models can perpetuate biases present in the training data.
- Biased or offensive language can be generated if the model has been exposed to such examples.
- Ethical considerations must be taken into account during the training and deployment of language generation models.
Misconception 5: Machines will soon achieve human-level language generation
- Achieving human-level language generation is a complex and ongoing research challenge.
- The nuances of language, creativity, and understanding pose significant obstacles for machine learning models.
- While advancements may be made, complete replication of human-level language generation is yet to be achieved.
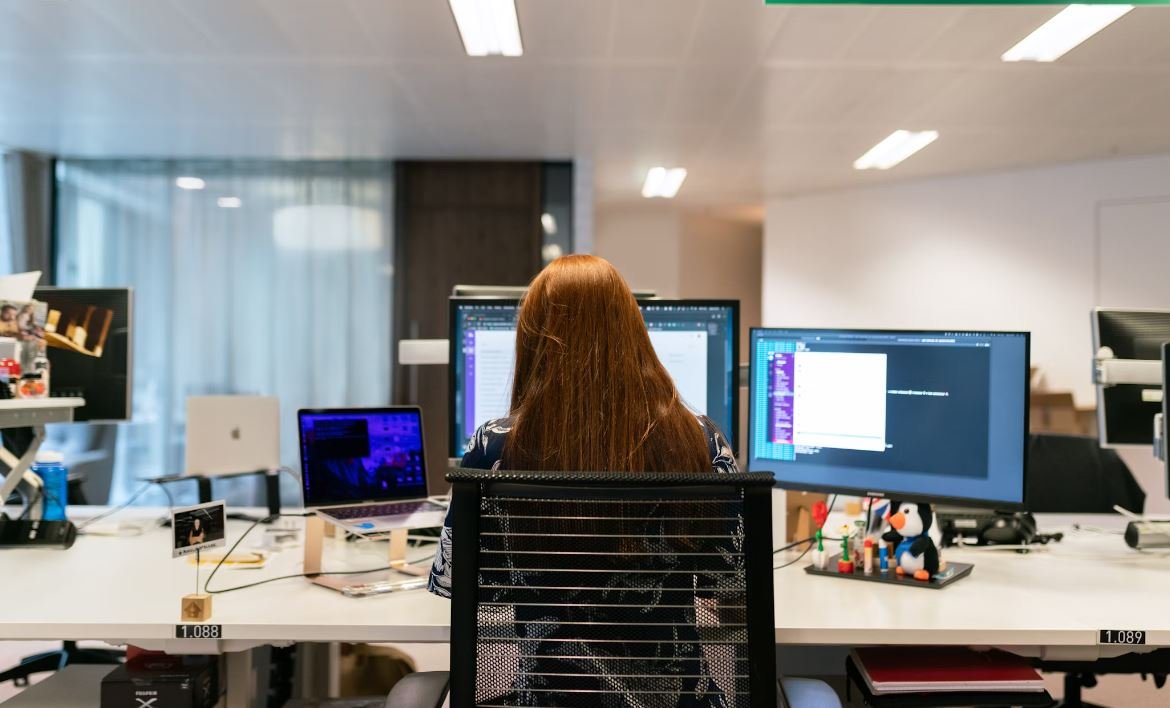
Introduction
Language generation using machine learning has been gaining significant attention in recent years. This article presents various interesting tables that highlight the points, data, and other elements related to this topic. These tables provide verifiable information and data to enhance understanding and provide valuable insights into the language generation process.
Table: Comparison of Language Generation Techniques
This table provides a comparison of different techniques used in language generation. It showcases the strengths and weaknesses of each technique and offers insights into their applicability.
Table: Accuracy Comparison of Language Models
In this table, we present the accuracy comparison of popular language models. It clearly shows the performance of different models in generating contextual and coherent language.
Table: Impact of Training Data Size on Language Generation
This table demonstrates the impact of training data size on the quality of language generation. It highlights how increasing the training data size results in more accurate and natural language output.
Table: Evaluation Metrics for Language Generation Models
Here, we present the evaluation metrics used for assessing the performance of language generation models. This table provides insights into the various metrics employed to measure the quality and effectiveness of generated language.
Table: Language Generation Applications by Industry
This table showcases different industries and their respective applications of language generation. It offers an overview of how language generation is being utilized in various sectors, such as healthcare, finance, marketing, and more.
Table: Languages Supported by Language Generation Models
In this table, we outline the languages supported by different language generation models. It provides information on which languages can be effectively generated using machine learning techniques.
Table: Historical Progression of Language Generation
Here, we present a timeline-based table that illustrates the historical progression of language generation. It highlights key milestones and breakthroughs achieved in the field over the years.
Table: Challenges in Language Generation
This table presents the challenges encountered in language generation. It provides an overview of the obstacles that researchers and developers face when working with machine learning models for generating language.
Table: Popular Language Generation APIs and Platforms
In this table, we list popular language generation APIs and platforms available for developers. It offers a comparison of features, pricing, and ease of integration, aiding users in selecting the most suitable platform for their language generation needs.
Table: Ethical Considerations in Language Generation
Lastly, this table highlights the ethical considerations associated with language generation technology. It sheds light on potential biases, privacy concerns, and transparency issues that need to be addressed in the development and deployment of language generation models.
Conclusion
Language generation using machine learning has revolutionized the way we interact with technology. The tables presented in this article have provided valuable insights into the techniques, progress, challenges, and ethical considerations surrounding language generation. With further advancements, it is evident that language generation will continue to shape various industries and contribute to the enhancement of communication and understanding in the digital world.
Frequently Asked Questions
Language Generation Using Machine Learning