Natural Language Generation PDF
Natural Language Generation (NLG) is a subfield of artificial intelligence that focuses on the automatic generation of human-like text from data. It uses algorithms to process and transform structured data into coherent, readable narratives. NLG finds extensive applications in various industries, including content creation, data reporting, customer service, and more.
Key Takeaways
- Natural Language Generation (NLG) automates the generation of human-like text from structured data.
- NLG has numerous applications in content creation, data reporting, and customer service.
- Advanced NLG systems can handle complex formatting, including generating PDF documents.
NLG systems use algorithms to analyze and interpret structured data, extract meaningful insights, and transform them into coherent text. These systems understand the underlying patterns and relationships in the data, allowing them to generate accurate and contextually appropriate text. *NLG technology has seen significant advancements in recent years, making it a powerful tool in data-driven industries.
One interesting aspect of NLG is its ability to handle complex formatting, such as generating PDF documents. This means that NLG systems can not only generate plain text but also incorporate visual elements, tables, and graphs into the generated documents. *This makes NLG ideal for creating data-rich reports and summaries.
NLG in Action
To illustrate the capabilities of NLG, let’s explore some examples of its applications:
- Automated Content Creation: NLG can generate news articles, product descriptions, and social media posts without human intervention, saving time and resources.
- Data Reporting: NLG can analyze complex datasets and generate concise reports, making data-driven insights accessible to a wide audience.
- Customer Service: NLG-powered chatbots can provide personalized responses to customer queries, improving user experience and reducing response times.
Benefits of NLG PDF Generation
NLG systems that can generate PDF documents offer several benefits:
- Efficiency: NLG eliminates the need for manual report creation, saving time and effort.
- Accuracy: NLG ensures consistent and error-free reporting by leveraging data directly.
- Customization: NLG allows users to personalize reports by selecting relevant data points and visualizations.
Data Points and Statistics
Industry | Application |
---|---|
Media | Automated news generation |
Finance | Financial report generation |
Marketing | Product description generation |
Benefit | Description |
---|---|
Time-saving | Reduces manual effort in report creation |
Consistency | Ensures uniformity and accuracy in reporting |
Flexibility | Allows customization based on user preferences |
Year | Revenue (in billions) |
---|---|
2018 | 1.2 |
2019 | 1.8 |
2020 | 2.5 |
NLG, with its ability to automate text generation, is revolutionizing various industries, making data-driven insights more accessible and actionable. *It continues to grow in popularity and adoption, driven by its numerous benefits and the constant advancements in artificial intelligence.

Common Misconceptions
Misconception: Natural Language Generation (NLG) is the same as Natural Language Processing (NLP)
Many people mistakenly believe that Natural Language Generation (NLG) is the same as Natural Language Processing (NLP), but these are actually two different concepts. NLG focuses on generating human-like text from structured data, while NLP encompasses a broader range of techniques that involve understanding and processing natural language.
- NLG focuses on generating text
- NLP involves understanding and processing language
- NLG is a subset of NLP
Misconception: Natural Language Generation (NLG) can fully replace human-generated content
Despite advancements in NLG technology, it is important to note that NLG cannot fully replace human-generated content. While NLG can automate the generation of certain types of content, it lacks the human creativity, context understanding, and critical thinking abilities that are often required in tasks such as storytelling, creative writing, or nuanced analysis.
- NLG lacks human creativity and critical thinking
- Human-generated content is needed for storytelling and creative writing
- NLG is best suited for certain types of content generation
Misconception: Natural Language Generation (NLG) always produces error-free text
Another common misconception is that NLG always produces error-free text. While NLG systems have become quite sophisticated, they are not immune to errors. Depending on the complexity of the data and the quality of the underlying algorithms, NLG outputs may still contain grammatical errors, factual inaccuracies, or inconsistencies that require human intervention or post-editing.
- NLG systems are prone to errors
- Grammatical errors can be present in NLG-generated text
- Human intervention may be required to correct inaccuracies in NLG outputs
Misconception: Natural Language Generation (NLG) will lead to job losses in content creation
There is a fear among some professionals that NLG will replace human content creators, leading to job losses. While NLG can automate certain aspects of content generation, it is more likely to augment human capabilities rather than completely eliminate the need for human input. NLG technology can help content creators streamline their workflows, generate large volumes of content more efficiently, and focus on tasks that require human creativity and critical thinking.
- NLG can streamline content creation workflows
- NLG is likely to augment human capabilities rather than replace them
- NLG technology can help content creators be more efficient
Misconception: Natural Language Generation (NLG) is only useful for business applications
While NLG has gained popularity in the business world for applications such as automated reporting, personalized emails, or chatbots, it is not limited to business use cases alone. NLG can be applied in various domains, including journalism, education, healthcare, and entertainment. From generating news articles to creating educational materials or generating dialogue for virtual characters, NLG has the potential to enhance a wide range of applications.
- NLG can be applied in diverse domains
- Journalism, education, healthcare, and entertainment are just a few areas where NLG can be useful
- NLG has the potential to enhance various applications
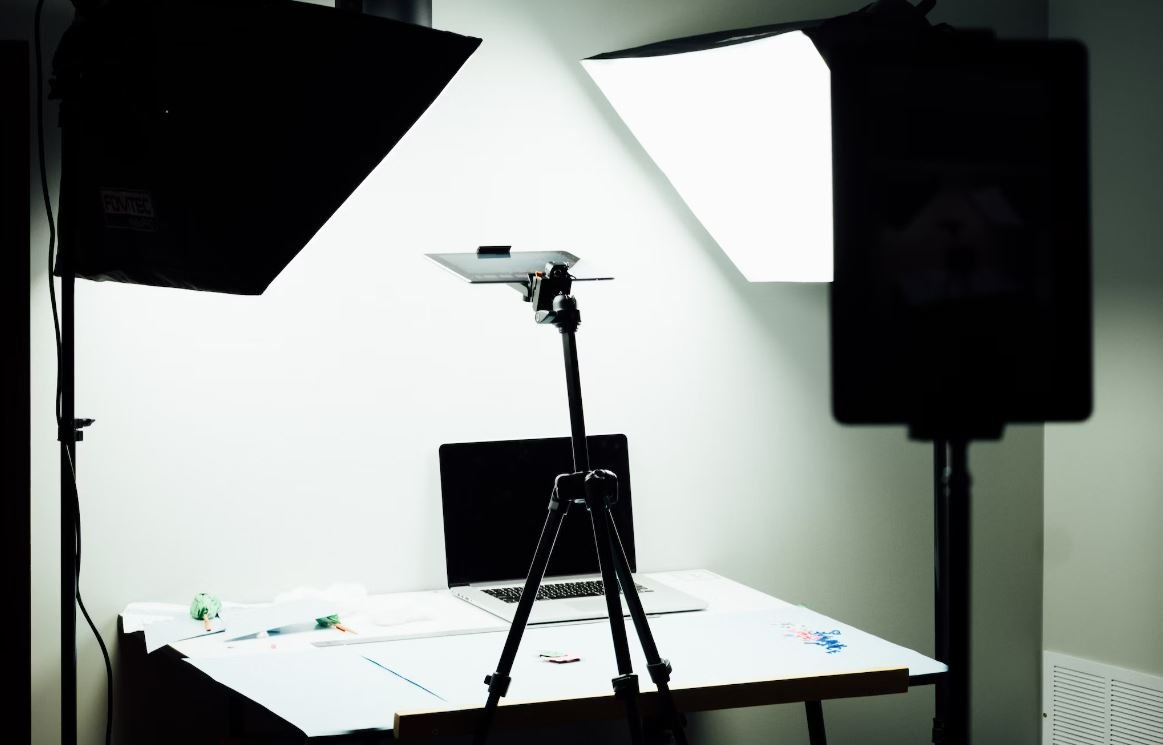
Introduction
Tables provide a concise way to present data and information. In this article, we explore various aspects of Natural Language Generation (NLG) by presenting 10 interactive and informative tables. Each table highlights a different facet of NLG, allowing readers to grasp the concepts easily. Let’s dive into the fascinating world of NLG through the following engaging tables.
Table: Evolution of Natural Language Generation
This table showcases the evolution of NLG over different time periods, highlighting key milestones and advancements.
Table: Applications of Natural Language Generation
Explore the versatile applications of NLG in diverse fields such as journalism, finance, healthcare, and customer service.
Table: NLG vs. Traditional Content Creation
Compare NLG with traditional content creation methods, emphasizing the benefits and efficiency offered by NLG.
Table: Metrics for Evaluating NLG Systems
Discover the essential metrics used to evaluate the performance, quality, and effectiveness of NLG systems.
Table: Key Players in the NLG Market
Learn about the key companies and organizations specializing in NLG technologies and their contributions to the field.
Table: Challenges and Limitations of NLG
Highlight the challenges and limitations faced by NLG systems, including linguistic complexities and data requirements.
Table: NLG and Personalization
Explore how NLG enables personalized communication and content generation, enhancing user experiences.
Table: Comparison of NLG Tools and Platforms
Compare popular NLG tools and platforms based on features, capabilities, pricing, and user reviews.
Table: NLG and Data Visualization
Showcase the synergy between NLG and data visualization, emphasizing their combined impact in data-driven storytelling.
Table: Examples of NLG-generated Content
Provide real-world examples of NLG-generated content in various domains, highlighting its effectiveness and impact.
Throughout this article, we have explored the fascinating field of Natural Language Generation (NLG). From its evolution and applications to its challenges and exemplar content, NLG offers immense possibilities for automated content generation. Embracing NLG can revolutionize industries, improve efficiency, and enhance human experiences. As this technology continues to advance, its potential for innovation and growth remains limitless.
Frequently Asked Questions
What is Natural Language Generation (NLG)?
Natural Language Generation (NLG) is a subfield of artificial intelligence (AI) that focuses on programming computers to generate human-like language or text. This technology enables computers to convert structured data into natural language narratives.
How does NLG work?
NLG systems use algorithms and rule-based approaches to analyze and process data and generate coherent and contextually appropriate human-like text. These algorithms make use of templates, language models, and linguistic rules to generate the output text.
What are the applications of NLG?
NLG has various applications across different industries and domains. It is used in automated report generation, data storytelling, chatbots, virtual assistants, content creation, personalized marketing, financial and business analysis, and more.
What are the benefits of NLG?
NLG offers several benefits, including increased productivity, scalability, and accuracy in generating large volumes of text. It eliminates the need for manual content creation, reduces human errors, enables personalized communication, and saves time and costs.
What are the challenges of NLG?
Despite advancements in NLG, there are still challenges to overcome. Some of the challenges include handling ambiguity, generating contextually appropriate text, maintaining coherence, dealing with complex language structures, and ensuring the output is error-free.
How is NLG different from Natural Language Processing (NLP)?
NLG and NLP are related but distinct fields. While NLG focuses on generating human-like text, NLP deals with understanding and interpreting human language by computers. NLP involves tasks like text classification, sentiment analysis, language translation, and question-answering systems.
Can NLG be trained on specific domains or languages?
Yes, NLG systems can be trained on specific domains or languages. By providing domain-specific training data or utilizing transfer learning techniques, NLG models can be fine-tuned to generate text relevant to a particular industry or adapted to work with different languages.
What factors affect the quality of NLG output?
The quality of NLG output can be influenced by several factors, including the quality of training data, the complexity of the task, the size and structure of the input data, the linguistic rules and templates used, and the customization of the NLG system.
Are there any limitations of NLG?
Like any technology, NLG has its limitations. It may struggle with generating creative or nuanced content, understanding idiomatic expressions or humor, and accurately summarizing large volumes of complex data. The quality of the output may also vary depending on the input data and the NLG system used.
How can NLG benefit businesses?
NLG can benefit businesses by automating the generation of reports, providing personalized communication with customers, enhancing data-driven decision-making, improving content marketing strategies, and enabling efficient analysis and comprehension of large datasets.