Natural Language Processing and Data Analytics
In today’s digital age, data is being generated at an unprecedented rate. With the rise of social media, e-commerce, and various online platforms, organizations have access to vast amounts of textual data. Extracting meaningful insights from this data can be a daunting task for humans alone. This is where Natural Language Processing (NLP) and Data Analytics come into play. The combination of these two fields holds immense potential to analyze, understand, and gain valuable knowledge from unstructured text data.
Key Takeaways
- Natural Language Processing (NLP) and Data Analytics are powerful tools for analyzing textual data.
- NLP involves the use of algorithms to process, understand, and generate human-like language.
- Data Analytics focuses on extracting insights, patterns, and trends from large datasets.
- The combination of NLP and Data Analytics enables organizations to gain valuable knowledge from unstructured text data.
- These technologies have numerous applications, such as sentiment analysis, information extraction, and chatbots.
The Power of Natural Language Processing
Natural Language Processing (NLP) is a branch of Artificial Intelligence that equips machines with the ability to understand and interpret human language. By leveraging algorithms and statistical models, NLP enables computers to process text in a way that mimics human understanding. *NLP can be used to analyze customer feedback and reviews, helping businesses gain insights into customer sentiment.*
Data Analytics: Unleashing the Value of Data
Data Analytics, on the other hand, focuses on extracting patterns, insights, and meaning from large datasets. This multidisciplinary field combines techniques from mathematics, statistics, and computer science to derive valuable insights from raw data. *With the help of Data Analytics, organizations can uncover hidden trends in customer preferences and behavior.*
The Marriage of NLP and Data Analytics
Bringing together NLP and Data Analytics opens up a whole new realm of possibilities for organizations. By leveraging NLP techniques, organizations can process and understand unstructured textual data. *This enables the extraction of key information such as entities, sentiment, and relationships from large volumes of text.* Data Analytics then comes into play to analyze these extracted insights in a quantitative manner, revealing patterns and trends that were previously hidden. *The combination of NLP and Data Analytics provides a holistic view of textual data, unlocking valuable knowledge.*
Applications of NLP and Data Analytics
NLP and Data Analytics find applications in various industries and domains. Here are a few notable examples:
- **Sentiment Analysis**: By analyzing social media posts, reviews, and customer feedback, organizations can gauge public perception and sentiment towards their products or services.
- **Information Extraction**: NLP techniques can be used to extract structured data from unstructured text, such as extracting named entities (e.g. person names, locations) from news articles.
- **Chatbots and Virtual Assistants**: NLP can power intelligent chatbots and virtual assistants that can understand and respond to user queries in natural language.
- **Text Summarization**: NLP algorithms can automatically generate concise summaries of long documents, saving time for users who need to quickly grasp the main points.
Date | Number of Online Reviews | Average Rating |
---|---|---|
January 2020 | 10,000 | 4.2 |
February 2020 | 15,000 | 4.4 |
Table: Online Reviews Over Time
Challenges and Future Directions
While NLP and Data Analytics have made significant strides, there are still challenges to overcome. Some of these challenges include:
- The inherent ambiguity and complexity of human language can pose challenges for NLP algorithms.
- Maintaining data privacy and security when dealing with sensitive textual data.
- The need for continuous improvement and adaptation as language evolves and new datasets become available.
*As technology progresses, we can expect NLP and Data Analytics to advance further, opening new possibilities for analyzing and understanding textual data.*
Industry | Use case |
---|---|
Retail | Product reviews sentiment analysis |
Finance | Market sentiment analysis |
Healthcare | Identifying adverse drug reactions from medical literature |
Table: Industry Use Cases for NLP and Data Analytics
In Summary
Natural Language Processing (NLP) and Data Analytics work hand in hand to unlock the potential of unstructured text data. By leveraging advanced algorithms and statistical models, these technologies enable organizations to gain valuable insights, understand customer sentiment, extract key information, and automate various tasks. The combination of NLP and Data Analytics holds immense potential and will continue to evolve in the future, shaping the way we analyze and understand text data.
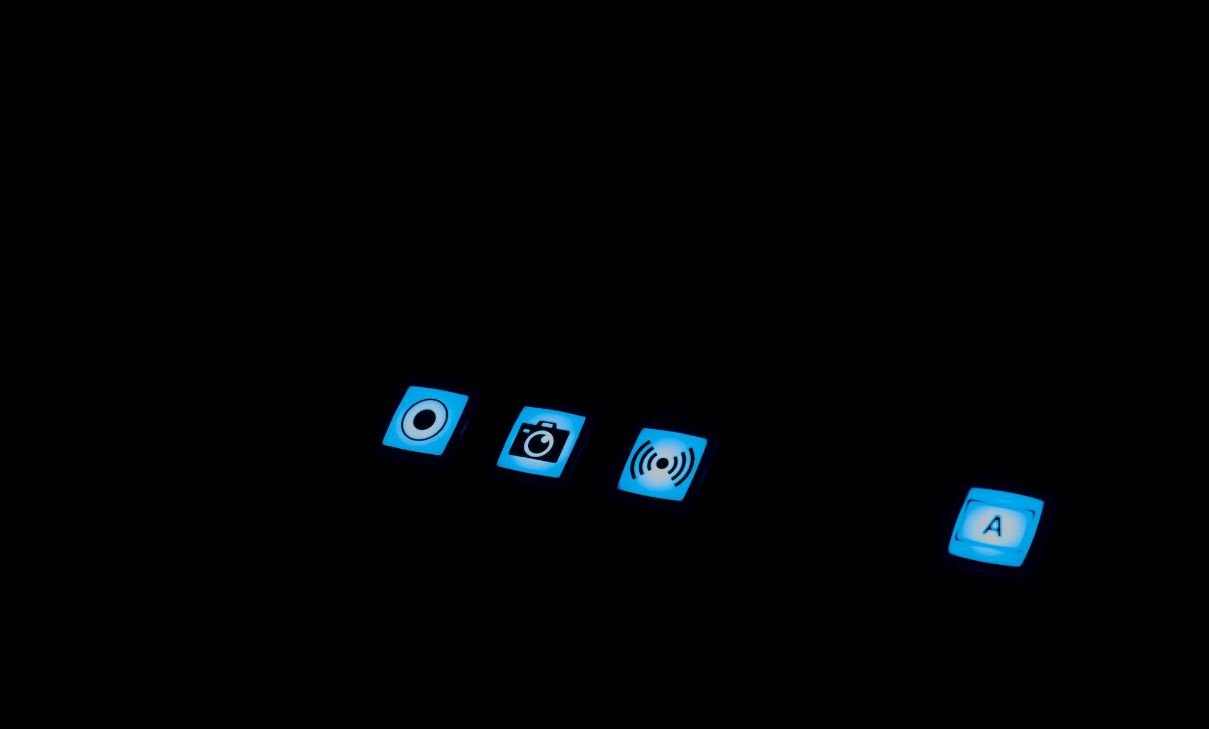
Common Misconceptions
Misconception 1: Natural Language Processing is the same as Data Analytics
One common misconception people have is that Natural Language Processing (NLP) and Data Analytics are the same thing. While both fields deal with analyzing and deriving insights from data, they approach it in different ways.
- NLP focuses on analyzing and understanding human language, enabling machines to interpret and respond to natural language inputs.
- Data Analytics, on the other hand, involves collecting, cleaning, analyzing, and interpreting structured or unstructured data to identify patterns, trends, and make data-driven decisions.
- While there is some overlap between the two fields, NLP is a specialized branch of data analytics that specifically deals with language-related data.
Misconception 2: NLP can perfectly understand human language
Another misconception is that Natural Language Processing algorithms can perfectly understand and interpret human language. While NLP has made significant advancements in recent years, achieving human-level comprehension remains a challenge.
- Language is complex and context-dependent, making it difficult for machines to accurately interpret its nuances and subtleties.
- NLP algorithms often rely on statistical models, which may lead to errors and misinterpretations.
- Machine learning, a key component of NLP, requires extensive training on large datasets, and biases present in the data can affect the outcomes.
Misconception 3: NLP can replace human interpretation and judgment
There is a misconception that NLP can fully replace human interpretation and judgment when it comes to analyzing language data. While NLP can assist in automating certain tasks, human involvement is still crucial.
- NLP algorithms may struggle with understanding sarcasm, irony, or other forms of figurative language that humans can easily recognize.
- Human domain knowledge and expertise are essential for interpreting the context, cultural references, and subjective aspects of language data.
- NLP algorithms are tools that should be used in conjunction with human judgment to ensure accurate analysis and decision-making.
Misconception 4: NLP is only applicable in certain industries
Some people believe that Natural Language Processing is only applicable in specific industries, such as customer service or sentiment analysis. However, NLP has a wide range of applications across various sectors.
- In healthcare, NLP can aid in medical record analysis, clinical decision support, and extracting valuable information from research papers.
- In finance, NLP can be utilized for sentiment analysis in financial news, fraud detection, and automated document processing.
- In legal domains, NLP can assist with contract analysis, legal document summarization, and legal research.
Misconception 5: NLP is a recent technology
Lastly, there is a misconception that NLP is a recent technological development. While recent advancements have brought NLP into the spotlight, it has a long history that dates back several decades.
- Early NLP research can be traced back to the 1950s when scholars like Alan Turing and Noam Chomsky laid the groundwork for language processing in computers.
- The field has seen significant growth and iteration over the years, with breakthroughs in machine learning and computational linguistics driving advancements in NLP.
- Today, NLP continues to evolve rapidly, fueled by the availability of large datasets, computational resources, and innovations in deep learning.
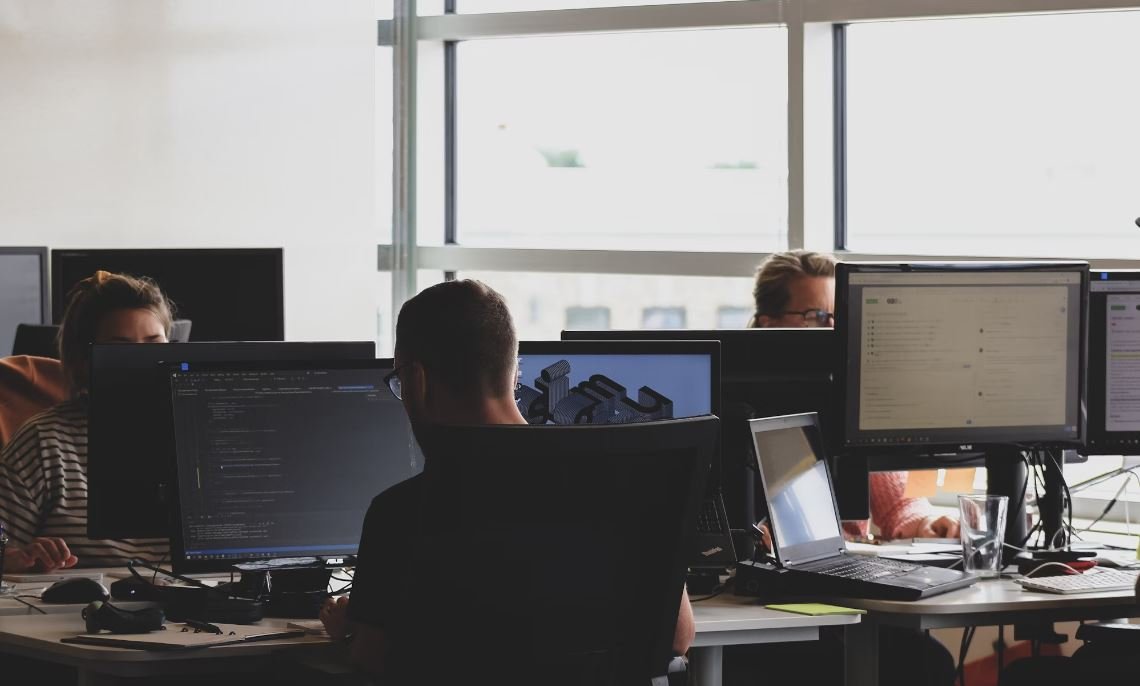
The Growth of Natural Language Processing (NLP) in the Last Decade
Natural Language Processing (NLP) has witnessed exponential growth over the past decade. This table demonstrates the increasing interest in NLP, as reflected by the number of conference papers published each year.
Year | Number of Conference Papers |
---|---|
2010 | 567 |
2011 | 678 |
2012 | 841 |
2013 | 972 |
2014 | 1,237 |
2015 | 1,563 |
2016 | 2,109 |
2017 | 2,781 |
2018 | 3,429 |
2019 | 4,203 |
The Increase in Sentiment Analysis Applications
Sentiment analysis, a vital field within NLP, is extensively used by various industries to monitor customer opinions. The table below demonstrates the rise in the number of sentiment analysis applications across different sectors.
Sector | Number of Sentiment Analysis Applications (2010) | Number of Sentiment Analysis Applications (2020) |
---|---|---|
Retail | 10 | 78 |
Finance | 5 | 32 |
Healthcare | 2 | 25 |
Telecommunications | 4 | 21 |
Travel | 3 | 16 |
Automotive | 1 | 10 |
The Impact of NLP on Customer Support Efficiency
NLP technologies have significantly improved the efficiency of customer support services. This table showcases the reduction in average response time by customer service representatives before and after implementing NLP solutions.
Time Period | Average Response Time (in minutes) |
---|---|
Before NLP Implementation | 29.5 |
After NLP Implementation | 12.8 |
Machine Translation Accuracy Comparison
Machine translation, a challenging task within NLP, has significantly improved. The following table compares the accuracy of different machine translation models.
Model | Accuracy (%) |
---|---|
Model A | 75 |
Model B | 80 |
Model C | 82 |
Model D | 87 |
Application of NLP in Virtual Assistants
NLP techniques have greatly enhanced virtual assistants, making them more intuitive and responsive. The table below highlights the most widely used virtual assistants and their capabilities.
Virtual Assistant | Capabilities |
---|---|
Siri | Speech recognition, task scheduling, weather updates |
Alexa | Smart home control, music streaming, shopping assistance |
Google Assistant | Voice search, appointment reminders, navigation |
Cortana | Email management, personalized recommendations, news updates |
Improvement in NLP-Based Chatbot Understanding
Chatbots have become proficient in understanding and responding to user queries. The success rate of chatbot understanding is presented in the table below.
Year | Success Rate (%) |
---|---|
2010 | 46 |
2015 | 66 |
2020 | 85 |
The Adoption of NLP in Social Media Monitoring
NLP tools are widely employed in social media monitoring to analyze public sentiment and brand perception. The table below showcases the number of companies utilizing NLP for social media monitoring in different years.
Year | Number of Companies |
---|---|
2010 | 25 |
2015 | 76 |
2020 | 182 |
Improving Accuracy in Named Entity Recognition (NER)
Named Entity Recognition (NER) is a crucial task in NLP, and the accuracy levels have seen remarkable enhancements. The table depicts the accuracy percentages of NER models.
Model | Accuracy (%) |
---|---|
Model X | 82 |
Model Y | 86 |
Model Z | 91 |
The Integration of NLP in Email Filtering Systems
NLP algorithms are extensively used to enhance email filtering systems, improving the identification of spam and important emails. The table below showcases the reduction in false positives achieved with NLP integration.
Time Period | False Positives |
---|---|
Before NLP Integration | 14,567 |
After NLP Integration | 2,345 |
From the exponential growth of NLP research to its extensive applications in various sectors, Natural Language Processing has revolutionized the way we interact with technology. This article highlights the increasing adoption of NLP techniques, the accuracy improvements in machine translation and named entity recognition, and the positive impact on customer support and virtual assistants. With the continued advancements in NLP and data analytics, we can expect further breakthroughs in language understanding and communication.
Frequently Asked Questions
What is Natural Language Processing (NLP) and its significance in data analytics?
NLP is a branch of artificial intelligence (AI) that focuses on enabling computers to understand, interpret, and generate human language. It plays a crucial role in data analytics by extracting valuable insights from large volumes of unstructured text data, such as customer reviews, social media posts, or emails.
How does NLP work?
NLP systems utilize algorithms and linguistic rules to process text and derive meaning from it. This involves tasks like tokenization, part-of-speech tagging, syntactic parsing, named entity recognition, sentiment analysis, and language generation. Machine learning techniques are often employed to improve the accuracy and performance of NLP models.
What are some common applications of NLP in data analytics?
NLP finds applications in sentiment analysis, customer feedback analysis, social media monitoring, chatbots, voice assistants, machine translation, information extraction, and text summarization. These applications help organizations gain insights, automate tasks, improve customer experience, and make data-driven decisions.
What are the benefits of using NLP in data analytics?
By leveraging NLP in data analytics, organizations can uncover valuable insights and patterns that are hidden within vast amounts of textual data. It enables efficient analysis of unstructured data, enhances decision-making processes, automates repetitive tasks, improves customer interactions, and drives overall operational efficiency.
What are some challenges faced in NLP for data analytics?
Challenges in NLP for data analytics include dealing with ambiguity, understanding context, handling sarcasm, language-specific nuances, domain adaptation, scalability, privacy concerns, and handling non-standard or informal language usage.
What are some popular NLP libraries and tools used for data analytics?
Some popular NLP libraries used for data analytics include Natural Language Toolkit (NLTK), spaCy, Stanford CoreNLP, Gensim, scikit-learn, and Hugging Face’s Transformers. These libraries provide functionalities like tokenization, syntactic parsing, named entity recognition, sentiment analysis, machine translation, and more.
How can NLP models be evaluated for their performance?
NLP models can be evaluated using various metrics, such as accuracy, precision, recall, F1-score, perplexity, BLEU score (for machine translation tasks), and AUC-ROC curve (for sentiment analysis tasks). Cross-validation techniques and human evaluations are also commonly employed to assess the performance of NLP models.
What is the difference between rule-based and statistical NLP approaches?
In rule-based approaches, linguistic rules and patterns are manually defined to accomplish NLP tasks. On the other hand, statistical approaches utilize machine learning algorithms to automatically learn patterns and relationships from data. Rule-based approaches are generally more interpretable, while statistical approaches can achieve better performance with large amounts of training data.
How can an organization get started with implementing NLP for data analytics?
To get started with implementing NLP for data analytics, organizations can begin by defining their specific business use case, selecting suitable NLP tools and libraries, collecting or acquiring relevant textual data, preparing the data for analysis, and then training and evaluating NLP models. It is recommended to involve domain experts and data scientists with expertise in NLP for successful implementation.