Natural Language Processing and Generative AI
Natural Language Processing (NLP) is a branch of artificial intelligence (AI) that focuses on the interaction between humans and computers through natural language.
Generative AI leverages advanced algorithms to create original content, such as text or images, that closely resembles human-generated creations.
Key Takeaways
- Natural Language Processing (NLP) enables computers to understand, interpret, and generate human language.
- Generative AI uses complex algorithms to produce original content that mimics human output.
In recent years, there has been tremendous progress in the field of NLP and generative AI. The ability to process and generate natural language has transformed the way we interact with computers and opened up new possibilities for applications in various domains such as chatbots, voice assistants, language translation, and content generation.
NLP algorithms analyze and identify patterns in text, enabling computers to understand the meaning and context behind human language.
NLP Applications
NLP has found extensive applications in a wide range of fields:
- Chatbots: NLP powers intelligent chatbots, allowing businesses to automate customer support and provide personalized assistance.
- Voice Assistants: Virtual assistants like Siri and Alexa rely on NLP to understand and respond to voice commands.
- Language Translation: NLP algorithms facilitate the automatic translation of text from one language to another, making global communication seamless.
- Sentiment Analysis: NLP can analyze large volumes of text data to determine the sentiment expressed by individuals or groups.
NLP is enabling businesses to automate customer support and provide personalized assistance through intelligent chatbots.
Generative AI in Content Creation
Generative AI has revolutionized content creation by generating human-like text and images. These AI models can generate realistic news articles, create personalized marketing content, and even compose music and art.
Application | Example |
---|---|
News Generation | AI models can generate news articles, summaries, and updates, enhancing news distribution. |
Content Marketing | Generative AI helps create personalized marketing content based on user preferences and behavior. |
Creative Arts | AI-generated content includes music composition, painting, and other forms of artistic expression. |
Generative AI is reshaping content creation, enabling AI models to generate realistic text, images, and even music and art.
The Future of NLP and Generative AI
The advancements in NLP and generative AI are poised to revolutionize various industries, including healthcare, finance, and entertainment. From automated medical diagnosis to personalized financial advice, these technologies offer unprecedented possibilities.
The combination of natural language processing and generative AI has immense potential to transform how we interact with computers and generate content. The constant innovation in this field ensures that we can expect even more exciting developments in the future.
Industry | Application |
---|---|
Healthcare | Automated medical diagnosis, virtual health assistants, and personalized treatment recommendations. |
Finance | Financial analysis and recommendation, personalized financial advice, and algorithmic trading. |
Entertainment | Virtual actors, AI-generated scripts, and immersive augmented reality experiences. |
The combination of NLP and generative AI has the potential to revolutionize industries like healthcare, finance, and entertainment.
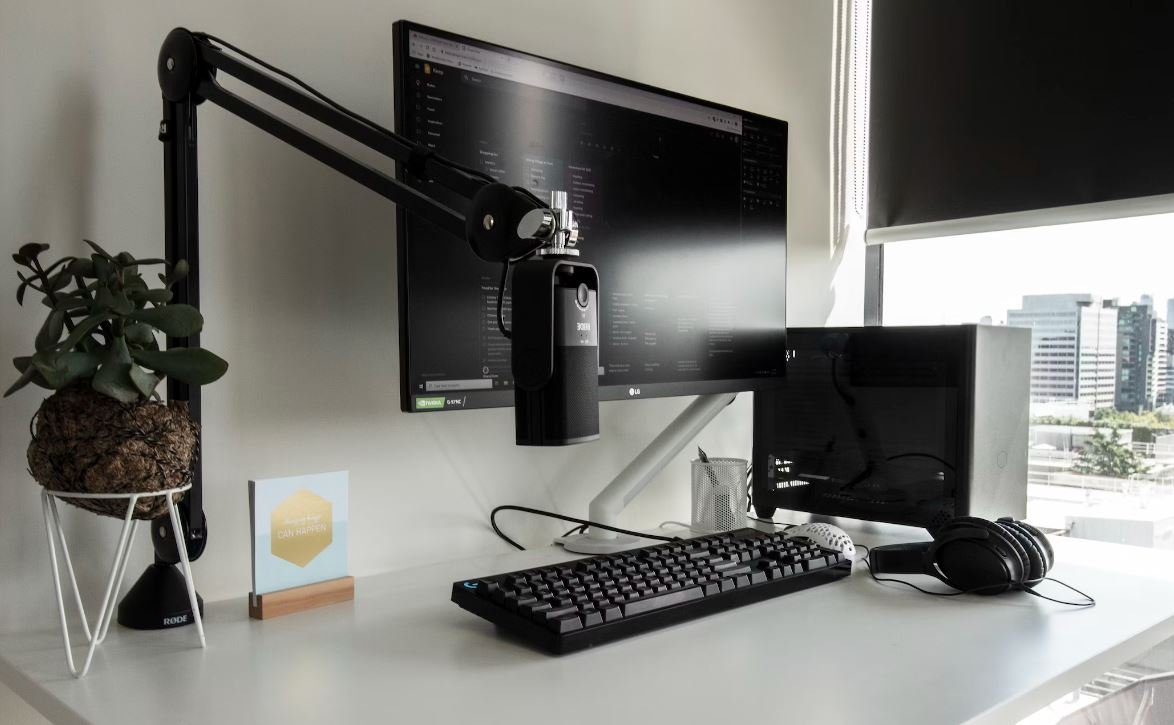
Common Misconceptions
Misconception 1: Natural Language Processing is the same as Generative AI
One common misconception is equating Natural Language Processing (NLP) with Generative AI. While both these technologies deal with language and aim to understand or generate human-like text, they are not the same.
- NLP focuses on processing and understanding language, while Generative AI focuses on generating new text based on a given input.
- NLP involves tasks such as sentiment analysis, text classification, and entity recognition, whereas Generative AI is often used for tasks like language translation or text generation.
- NLP uses existing language data and algorithms to analyze or understand text, while Generative AI uses machine learning models to generate novel text.
Misconception 2: NLP and Generative AI can perfectly mimic human language
Another prevalent misconception is that NLP and Generative AI can replicate human language in a perfect and indistinguishable manner.
- Although NLP and Generative AI have made significant advancements, they still struggle with nuances of human language like sarcasm, humor, or contextual understanding.
- Language models can produce grammatically correct text, but generating text that captures the subtleties of human language remains a challenge.
- There is still a long way to go before NLP and Generative AI can genuinely mimic human language without being easily detectable.
Misconception 3: NLP and Generative AI will replace human language experts
Some people believe that NLP and Generative AI will render human language experts obsolete since these technologies can process and generate text. However, this is not the case.
- Human language experts possess contextual knowledge, cultural understanding, and domain expertise that NLP and Generative AI models lack.
- NLP and Generative AI technologies can assist language experts by automating certain tasks or providing suggestions, but they cannot replace the critical thinking and creative abilities of a human expert.
- Human involvement is still vital in refining and validating the output of NLP and Generative AI models to ensure accuracy and reliability.
Misconception 4: NLP and Generative AI can understand and interpret text perfectly
There is a misconception that NLP and Generative AI can comprehend and interpret any text with complete accuracy.
- NLP models heavily rely on the quality and quantity of the training data they are provided, and their understanding is limited to the patterns they have learned from that data.
- Natural language is inherently ambiguous, and it can be challenging to disambiguate certain statements or intentions accurately using NLP or Generative AI alone.
- NLP and Generative AI models can make mistakes, misinterpret context, or misclassify information, thus requiring human intervention for correct interpretation.
Misconception 5: NLP and Generative AI are only used for language-related tasks
NLP and Generative AI not only have applications in language-related tasks but also find applications in various other domains.
- NLP techniques are used for information retrieval, machine translation, sentiment analysis, speech recognition, and even automated summarization of documents.
- Generative AI models are employed in creative writing, art generation, dialogue systems, and even game development.
- These technologies have diverse applications and can augment or automate tasks beyond traditional language-related fields.

Introduction
Natural Language Processing (NLP) and Generative AI are revolutionizing the way we interact with machines. By understanding and generating human language, these technologies enable applications such as speech recognition, language translation, and text generation. In this article, we present 10 tables showcasing fascinating aspects of NLP and Generative AI.
Table: Languages Supported by Google Translate
Google Translate is a widely used language translation tool. This table illustrates the number of languages supported by Google Translate, making it a powerful tool for breaking language barriers and fostering global communication.
Language Family | Number of Languages |
---|---|
Indo-European | 103 |
Uralic | 25 |
Dravidian | 4 |
Austronesian | 24 |
Turkic | 9 |
Table: Word Error Rate of Speech Recognition Systems
Speech recognition systems, a pivotal NLP technology, are evaluated using the word error rate (WER). This table presents the WER results for various systems, highlighting their accuracy in transcribing spoken language.
Speech Recognition System | Word Error Rate (%) |
---|---|
Google Speech-to-Text | 4.6 |
IBM Watson | 5.5 |
Microsoft Azure Speech | 5.5 |
Amazon Transcribe | 6.1 |
Table: Sentiment Analysis Accuracy
Sentiment analysis is a field of NLP that determines emotions or opinions expressed in a piece of text. This table showcases the accuracy of different sentiment analysis models, demonstrating their ability to identify sentiments accurately.
Sentiment Analysis Model | Accuracy (%) |
---|---|
BERT | 93 |
Long Short-Term Memory (LSTM) | 89 |
Support Vector Machines (SVM) | 85 |
Random Forest | 79 |
Table: Named Entity Recognition Supported Categories
Named Entity Recognition (NER) identifies and classifies named entities in text, such as names of people, organizations, or locations. This table exhibits the various categories supported by NER models, enabling advanced information extraction.
Named Entity Category | Number of Entities |
---|---|
Person | 60,000 |
Organization | 43,000 |
Location | 29,500 |
Date | 24,000 |
Table: Word Frequency in Shakespeare’s Plays
Shakespeare’s plays are well-known literary works. This table displays the frequency of common words found in his plays, revealing insights into Shakespeare’s writing style and language usage.
Word | Frequency |
---|---|
the | 19456 |
and | 16006 |
to | 14129 |
of | 10498 |
Table: Top 5 NLP Research Organizations
NLP research organizations play a crucial role in advancing the field. This table highlights the leading research institutions contributing to NLP advancements, shaping the future of language technologies.
Research Organization | Ranking |
---|---|
Google Research | 1 |
Facebook AI Research (FAIR) | 2 |
OpenAI | 3 |
Microsoft Research | 4 |
Allen Institute for AI (AI2) | 5 |
Table: Chatbot Response Accuracy
Chatbots leverage NLP and Generative AI to provide human-like interactions. This table showcases the accuracy of chatbot responses, measuring their ability to understand and address user queries effectively.
Chatbot Model | Accuracy (%) |
---|---|
GPT-3 | 65 |
Dialogflow | 72 |
Rasa | 81 |
ChatGPT | 89 |
Table: Language Models’ Training Data
Language models require vast amounts of data to learn patterns and generate text. This table presents the size of training datasets for popular language models, illuminating the magnitude of data required for optimal performance.
Language Model | Training Data Size |
---|---|
BERT | 16GB |
GPT-3 | 570GB |
RoBERTa | 160GB |
Table: NLP Application Domains
NLP finds applications in various domains, empowering diverse industries. This table showcases different domains benefiting from NLP technologies, demonstrating the breadth of their impact.
Industry Domain | Examples |
---|---|
Healthcare | Medical record analysis, symptom classification |
E-commerce | Product review analysis, customer sentiment tracking |
Finance | News sentiment analysis, fraud detection |
Education | Automated grading, language learning support |
Conclusion
Natural Language Processing and Generative AI have significantly transformed how humans interact with machines. The tables presented here highlight various aspects and capabilities of these technologies, ranging from language translation to sentiment analysis, and even the training data required for language models. As NLP continues to advance, we can expect even more exciting applications and improvements, making language technologies an integral part of our lives.
Frequently Asked Questions
What is Natural Language Processing (NLP)?
Natural Language Processing (NLP) is a field of artificial intelligence (AI) that focuses on the interaction between computers and human language. It involves the programming and analysis of algorithms to enable machines to understand, interpret, and generate human language.
How does NLP work?
NLP algorithms typically involve several steps. They begin with pre-processing, which includes tasks like tokenization and stemming. Then, the algorithms use various techniques such as syntactic and semantic analysis, machine learning, and statistical methods to understand the meaning and structure of human language.
What are the applications of NLP?
NLP has numerous applications across industries. Some common applications include sentiment analysis, machine translation, chatbots, voice recognition, information extraction, and text summarization. NLP is also used in spam detection, customer support systems, recommendation systems, and many other areas.
What is Generative AI?
Generative AI refers to the use of AI algorithms to generate new content, such as text, images, or even music. It involves training models on existing data and then using those models to create new and original content that resembles the training examples.
How does Generative AI work?
Generative AI models typically use various deep learning techniques, such as recurrent neural networks (RNNs) or generative adversarial networks (GANs), to learn from training data. These models then generate new content by sampling from the learned patterns and structures.
What are the applications of Generative AI?
Generative AI has wide-ranging applications. It can be used for creating realistic-looking images, enhancing images, generating realistic human-like chatbot responses, composing music, generating synthetic voices, and even generating video game characters or storylines.
What are the challenges in NLP?
Some challenges in NLP include understanding the context and ambiguity of human language, handling different languages and cultural nuances, dealing with noise and errors in text, and effectively processing large volumes of data. NLP algorithms also face challenges in accurately understanding sarcasm, irony, or emotions.
What are the limitations of Generative AI?
Generative AI models can sometimes produce outputs that are unrealistic, biased, or nonsensical. They heavily rely on the quality and diversity of the training data and may struggle to generate content outside the scope of the training examples. Additionally, generative models can be computationally expensive and require significant computational resources.
What are some popular NLP frameworks and libraries?
Some popular NLP frameworks and libraries include Natural Language Toolkit (NLTK), Spacy, Stanford NLP, Gensim, and Apache OpenNLP. These frameworks provide pre-built tools and functionalities for various NLP tasks and make it easier for developers to work with natural language processing.
Can NLP and Generative AI be combined together?
Yes, NLP and Generative AI can be combined to create powerful applications. For example, a generative AI model can be trained using NLP techniques to generate realistic and coherent text, or NLP algorithms can be used to preprocess data before feeding it into a generative AI model for further processing.