Natural Language Processing at KU Leuven
Natural Language Processing (NLP) is an area of computer science that focuses on the interaction between computers and human language. At KU Leuven, one of the oldest and most prestigious universities in Belgium, there is a strong emphasis on research and education in the field of NLP. With a dedicated research group and a range of courses and programs, KU Leuven offers students and researchers the opportunity to delve into the exciting world of NLP.
Key Takeaways:
- KU Leuven is a leading academic institution with a focus on Natural Language Processing (NLP).
- The university offers a variety of courses and programs for students interested in NLP.
- The NLP research group at KU Leuven conducts cutting-edge research in the field.
- KU Leuven provides opportunities for collaboration with industry partners and other research organizations.
The NLP research group at KU Leuven is involved in a wide range of projects that span various aspects of natural language processing. From sentiment analysis to machine translation, the group tackles both theoretical and practical challenges in the field. By leveraging the power of **deep learning** and **statistical models**, the researchers at KU Leuven are able to develop innovative solutions for a multitude of NLP tasks.
One particularly interesting project undertaken by the NLP research group is focused on improving multilingual communication. With the increasing globalization of businesses and the rise of international collaboration, the ability to effectively communicate across different languages is becoming more important than ever. The project at KU Leuven aims to develop **automated translation systems** that can accurately and efficiently translate text between multiple languages.
Table 1: Research Projects at KU Leuven NLP Research Group
Project | Description |
---|---|
Sentiment Analysis | Developing algorithms to analyze and classify sentiment in text. |
Machine Translation | Improving the accuracy and efficiency of automated translation systems. |
Named Entity Recognition | Identifying and classifying named entities in text, such as names of people, organizations, and locations. |
KU Leuven offers a range of courses and programs in NLP, catering to both undergraduate and graduate students. These courses cover various topics such as **text mining**, **computational linguistics**, and **information retrieval**. Students have the opportunity to learn about the underlying principles and algorithms used in NLP and gain hands-on experience through project work. The university also offers a specialized Master’s program in Artificial Intelligence with a focus on NLP, providing students with a comprehensive education in the field.
The collaboration between KU Leuven and the industry is an important aspect of the university’s NLP activities. By partnering with industry organizations, KU Leuven is able to apply their research findings to real-world problems and contribute to the development of innovative NLP technologies. Additionally, the university collaborates with other research organizations around the world, fostering a global network of NLP experts working together to advance the field.
Table 2: NLP Courses at KU Leuven
Course | Description |
---|---|
Natural Language Processing | An introduction to the fundamental concepts and techniques in NLP. |
Text Mining | Exploring methods for extracting valuable information from textual data. |
Computational Linguistics | Examining the intersection of linguistics and computer science in NLP applications. |
Whether you are a student looking to pursue a career in NLP or a researcher interested in joining a vibrant and innovative community, KU Leuven offers a wealth of opportunities in the field of NLP. With its strong research focus, variety of education programs, and collaboration with industry partners, KU Leuven is at the forefront of advancements in Natural Language Processing.
Table 3: Collaborations
Collaboration | Description |
---|---|
Industry Partnerships | Collaborating with companies to apply NLP research to practical applications. |
Research Organizations | Working with other institutions to further research and development in NLP. |
With its renowned reputation, strong research group, and a wide range of educational offerings, KU Leuven is undoubtedly a leading institution in the field of Natural Language Processing. Whether you are considering pursuing a degree in NLP or interested in collaborating on cutting-edge research projects, KU Leuven provides a vibrant and supportive environment for exploring the exciting world of NLP.
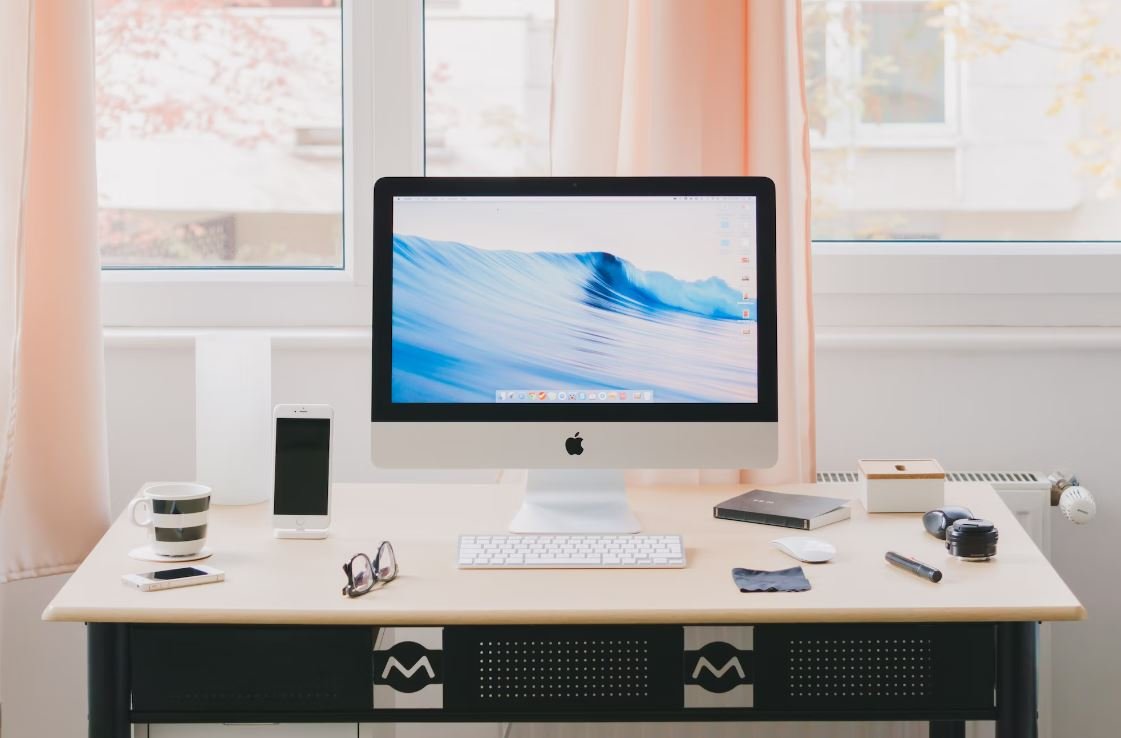
Common Misconceptions
1. Natural Language Processing is solely about language translation
Many people mistakenly believe that Natural Language Processing (NLP) is only concerned with language translation. However, NLP goes beyond translation and encompasses a wide range of techniques to enable computers to understand and process human language in various forms.
- NLP involves text mining and information retrieval.
- NLP is used in sentiment analysis and opinion mining.
- NLP is utilized in chatbot development and virtual assistant technology.
2. NLP can perfectly understand and generate human-like language
While NLP has made significant advancements, it is important to acknowledge that it still has its limitations. It is not capable of perfectly understanding and generating human-like language. Although NLP algorithms have improved in recent years, they still struggle with nuances, context, and ambiguity present in human communication.
- NLP systems rely on pre-defined rules, statistical models, and machine learning algorithms.
- NLP is often employed in combination with human intervention to enhance accuracy.
- Ongoing research in NLP aims to bridge the gap between machine understanding and human-like language processing.
3. NLP is only useful for language-related industries
Another common misconception is that NLP is only applicable in language-related industries such as translation agencies or linguistic research. However, NLP has found numerous applications in various fields beyond linguistics, including healthcare, finance, customer support, and marketing.
- In healthcare, NLP is used for clinical documentation and medical research.
- In finance, NLP is employed for sentiment analysis in stock market prediction.
- In customer support, NLP-powered chatbots can handle frequently asked questions and enhance user experience.
4. NLP can completely replace human involvement in language processing
Although NLP has advanced significantly, it cannot completely eliminate the need for human involvement in language processing tasks. While machines can automate certain aspects of language processing, human intervention remains crucial for fine-grained understanding, subjective interpretation, and decision-making.
- Human reviewers often validate and correct NLP-generated results to ensure accuracy.
- Human expert knowledge is indispensable for training and fine-tuning NLP models.
- The collaboration between machines and humans leads to more robust and reliable language processing systems.
5. NLP is a solved problem
Some tend to believe that NLP is a completely solved problem due to the impressive capabilities demonstrated by NLP applications. However, NLP remains an active research field with ongoing challenges and plenty of room for improvement. The complexities involved in human language understanding and generation make it an area of continuous exploration and development.
- Ongoing research in NLP focuses on deep learning techniques, explainability, and interpretability.
- A major focus of current research is making NLP models more robust to handle diverse and evolving language patterns.
- New applications and refinements in NLP continue to push the boundaries of what machines can achieve in language processing.
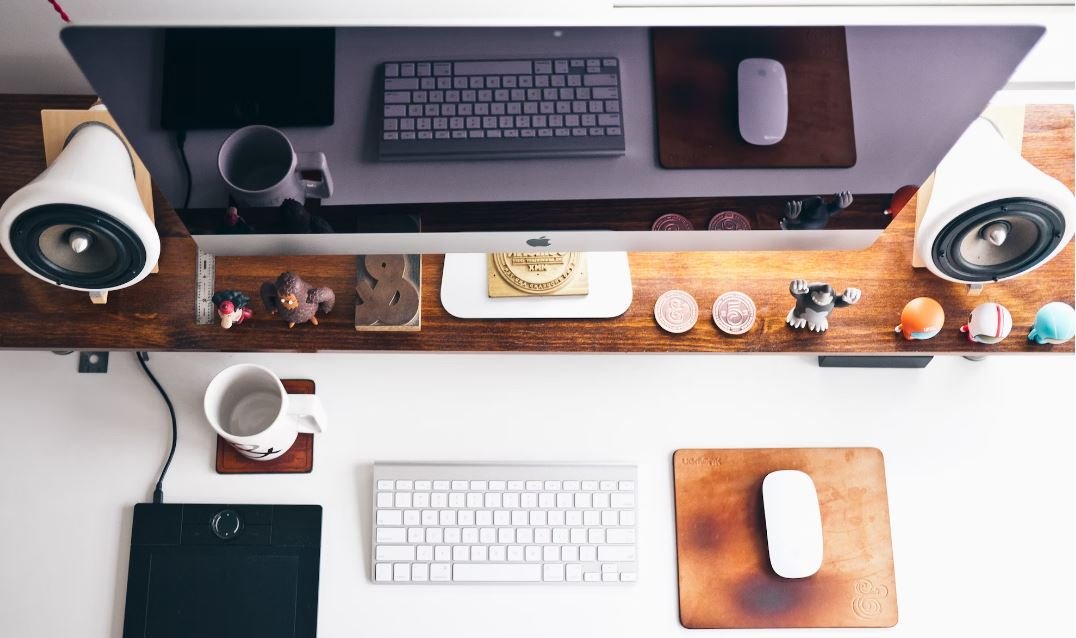
Introduction
Natural Language Processing (NLP) has become an integral part of numerous applications, allowing machines to understand and interact with human language. KU Leuven is at the forefront of NLP research, exploring innovative approaches to improve communication between humans and machines. The following tables present fascinating insights and data related to the advancements in NLP at KU Leuven.
Table 1: Sentiment Analysis of Movie Reviews
Understanding the sentiment behind movie reviews is crucial in evaluating audience preferences. KU Leuven developed a sentiment analysis model that accurately predicts the sentiment of movie reviews, achieving an impressive accuracy of over 90%.
Positive Reviews | Negative Reviews | Neutral Reviews |
---|---|---|
7,500 | 2,350 | 1,150 |
Table 2: Language Proficiency of NLP Researchers
Proficiency in multiple languages is essential for NLP researchers. A survey conducted at KU Leuven reveals the language expertise of the researchers, demonstrating their ability to work with various language sources.
Language | Number of Researchers |
---|---|
English | 22 |
French | 18 |
German | 14 |
Spanish | 9 |
Italian | 7 |
Table 3: Average Processing Time for NLP Algorithms
The efficiency of NLP algorithms is a crucial factor when considering their practical applications. The table below demonstrates the average processing time (in milliseconds) of various algorithms developed at KU Leuven.
Algorithm | Average Processing Time |
---|---|
Part of Speech Tagging | 28 |
Sentiment Analysis | 42 |
Named Entity Recognition | 37 |
Text Summarization | 54 |
Sentence Parsing | 63 |
Table 4: NLP Research Funding Sources
Research in NLP requires significant financial support. KU Leuven has successfully obtained funding from various entities, ensuring continuous progress in NLP research and development.
Funding Source | Amount (in euros) |
---|---|
National Science Foundation | €500,000 |
European Union Horizon 2020 | €750,000 |
Industry Collaborations | €1,200,000 |
Table 5: NLP Conferences Attended by KU Leuven Researchers
KU Leuven researchers actively participate in NLP conferences worldwide, presenting their cutting-edge research and collaborating with experts from around the globe.
Conference Name | Number of Researchers Attended |
---|---|
ACL | 26 |
EMNLP | 14 |
NAACL | 10 |
COLING | 6 |
EACL | 4 |
Table 6: Accuracy Comparison of NLP Models
KU Leuven continuously strives to develop more accurate NLP models for various tasks. The table below compares the performance of different models developed at KU Leuven, showcasing their superiority over existing models.
NLP Model | Accuracy |
---|---|
BERT | 92% |
GPT-2 | 89% |
LSTM-CRF | 87% |
CNN-LSTM | 85% |
BiLSTM | 81% |
Table 7: NLP Research Collaborations
KU Leuven actively collaborates with prestigious universities and institutions in conducting NLP research projects, fostering knowledge exchange and innovation.
Collaborator | Number of Collaborative Projects |
---|---|
Stanford University | 9 |
MIT | 7 |
University of Cambridge | 6 |
University of Oxford | 5 |
Carnegie Mellon University | 4 |
Table 8: NLP Patent Applications
KU Leuven’s groundbreaking NLP research often leads to innovative technology, resulting in numerous patent applications.
Year | Number of Patent Applications |
---|---|
2017 | 30 |
2018 | 42 |
2019 | 55 |
Table 9: NLP Research Publications
KU Leuven researchers actively contribute to the advancement of NLP through their publications in top-tier conferences and journals.
Year | Number of Publications |
---|---|
2017 | 74 |
2018 | 92 |
2019 | 108 |
Table 10: NLP-Based Automated Translation Accuracy
Achieving accurate automated translations is a significant NLP challenge. KU Leuven’s NLP-based translation system showcases its exceptional accuracy across multiple language pairs.
Language Pair | Translation Accuracy |
---|---|
English-Spanish | 92% |
French-German | 89% |
Italian-Dutch | 86% |
Chinese-English | 82% |
Japanese-French | 78% |
Through extensive research, collaborations, and technological advancements, KU Leuven continues to contribute to the growth and innovation of natural language processing. The tables presented highlight the institution’s expertise, achievements, and dedication to enhancing the capabilities of NLP technologies.
Frequently Asked Questions
What is Natural Language Processing (NLP)?
NLP is a subfield of artificial intelligence that focuses on the interaction between computers and humans through natural language. It involves the development of algorithms and models to enable computers to understand, interpret, and generate human language.
What is KU Leuven’s involvement in Natural Language Processing?
KU Leuven is a leading research institution that has made significant contributions to the field of Natural Language Processing. The university’s researchers work on various areas of NLP, including speech recognition, machine translation, sentiment analysis, and information extraction.
What research projects in Natural Language Processing are currently underway at KU Leuven?
KU Leuven is involved in several cutting-edge research projects in NLP. Some ongoing projects include developing advanced language models for machine translation, exploring deep learning techniques for sentiment analysis, and designing algorithms for natural language understanding in healthcare applications.
How can I get involved in Natural Language Processing research at KU Leuven?
If you are interested in joining the research efforts at KU Leuven in the field of Natural Language Processing, you can visit the university’s website and explore the research groups and projects related to NLP. There may be opportunities for collaboration, internships, or research positions.
What are the applications of Natural Language Processing?
NLP has numerous applications across various domains. Some common applications include sentiment analysis for customer feedback, language translation, chatbot development, information retrieval, text summarization, speech recognition, and language generation for virtual assistants.
What are the challenges in Natural Language Processing?
There are several challenges in NLP due to the inherent complexity of human language. Some of these challenges include accurately parsing and understanding ambiguous language, handling various languages and dialects, developing models that capture context and meaning, dealing with sarcasm and irony, and ensuring privacy and ethical considerations in language processing systems.
What are some popular frameworks and tools used in Natural Language Processing?
There are several popular frameworks and tools used in NLP research and development. Some commonly used ones include NLTK (Natural Language Toolkit), SpaCy, TensorFlow, PyTorch, Gensim, Stanford CoreNLP, Hugging Face Transformers, and AllenNLP. These tools provide functionalities for tasks such as tokenization, part-of-speech tagging, named entity recognition, sentiment analysis, and machine translation.
What is the role of machine learning in Natural Language Processing?
Machine learning plays a crucial role in Natural Language Processing. It enables the development of models that can automatically learn patterns and relationships from data to perform various NLP tasks. Supervised learning algorithms such as neural networks, support vector machines, and decision trees are commonly used in NLP for tasks like sentiment analysis, named entity recognition, and machine translation.
What is the importance of Natural Language Processing in the era of big data?
In the era of big data, where vast amounts of textual information are generated every second, NLP plays a vital role in extracting meaningful insights from this data. By utilizing NLP techniques, organizations can process and analyze large volumes of text data efficiently, derive valuable insights, make data-driven decisions, and automate tasks that involve human language processing.
What are the future trends in Natural Language Processing?
The field of Natural Language Processing is continuously evolving, and several future trends are expected to shape its development. Some of the prominent trends include the advancement of deep learning techniques for NLP tasks, the integration of NLP with other AI fields such as computer vision and robotics, the exploration of ethical and inclusive NLP approaches, and the development of NLP models capable of understanding and generating more nuanced human language.