Natural Language Processing Statistics
Natural Language Processing (NLP) is a branch of artificial intelligence (AI) that focuses on the interaction between humans and computers through natural language. It aims to enable computers to understand and interpret human language, allowing them to communicate effectively with humans. NLP has gained significant attention in recent years due to its potential in various fields, such as chatbots, sentiment analysis, and language translation. In this article, we will explore some key statistics related to natural language processing.
Key Takeaways:
- Natural Language Processing (NLP) enables computers to understand and interpret human language.
- NLP has gained significant attention due to its potential in fields such as chatbots and sentiment analysis.
- The global NLP market is expected to grow at a CAGR of 22.5% from 2021 to 2028.
- Chatbots powered by NLP can save up to 30% in customer support costs.
1. Growth of the NLP Market: The global natural language processing market is expected to grow at a compound annual growth rate (CAGR) of 22.5% from 2021 to 2028. This significant growth is fueled by the increasing adoption of NLP in various industries, including healthcare, customer service, and finance.
2. Cost Savings with NLP-powered Chatbots: Chatbots integrated with NLP capabilities can bring substantial cost savings to businesses. According to a Juniper Research report, chatbots powered by NLP can save up to 30% in customer support costs by automating responses and handling routine inquiries, allowing human agents to focus on more complex issues.
3. The Rise of Voice Assistants: Voice assistants have become increasingly prevalent in our daily lives, thanks to advancements in NLP technology. Popular voice assistants such as Siri, Alexa, and Google Assistant leverage NLP algorithms to understand and respond to voice commands, making tasks like setting reminders, searching the web, and controlling smart devices much simpler.
4. NLP in Sentiment Analysis: Sentiment analysis, a field within NLP, focuses on understanding and classifying the sentiments expressed in text data. It finds applications in analyzing social media sentiment, customer reviews, and market research. NLP techniques enable sentiment analysis algorithms to accurately identify positive, negative, and neutral sentiments, aiding businesses in understanding consumer opinions and improving customer experiences.
Table 1: Applications of NLP | Table 2: Benefits of NLP-powered Chatbots |
---|---|
|
|
5. NLP for Language Translation: NLP plays a vital role in language translation services. Machine Translation, a subfield of NLP, focuses on developing algorithms that can automatically translate text from one language to another. With the advent of neural machine translation models, such as Google Translate, the accuracy of machine translation has significantly improved, making it an invaluable tool for businesses and individuals alike.
6. Challenges in NLP: While NLP has made significant advancements, there are still challenges to overcome. Understanding context, sarcasm, and nuances in language, especially in diverse cultures, remains a challenge for NLP systems. Developing robust algorithms that can handle such complexities is an ongoing focus for researchers in the field.
Table 3: NLP Challenges |
---|
|
In conclusion, NLP has revolutionized the way computers process and understand human language. With the growing demand for intelligent language applications, the NLP market is expected to experience consistent growth in the coming years. By leveraging NLP techniques, businesses can improve customer experiences, automate processes, and gain valuable insights from textual data. As NLP technology continues to evolve, we can anticipate even more exciting developments in the future.
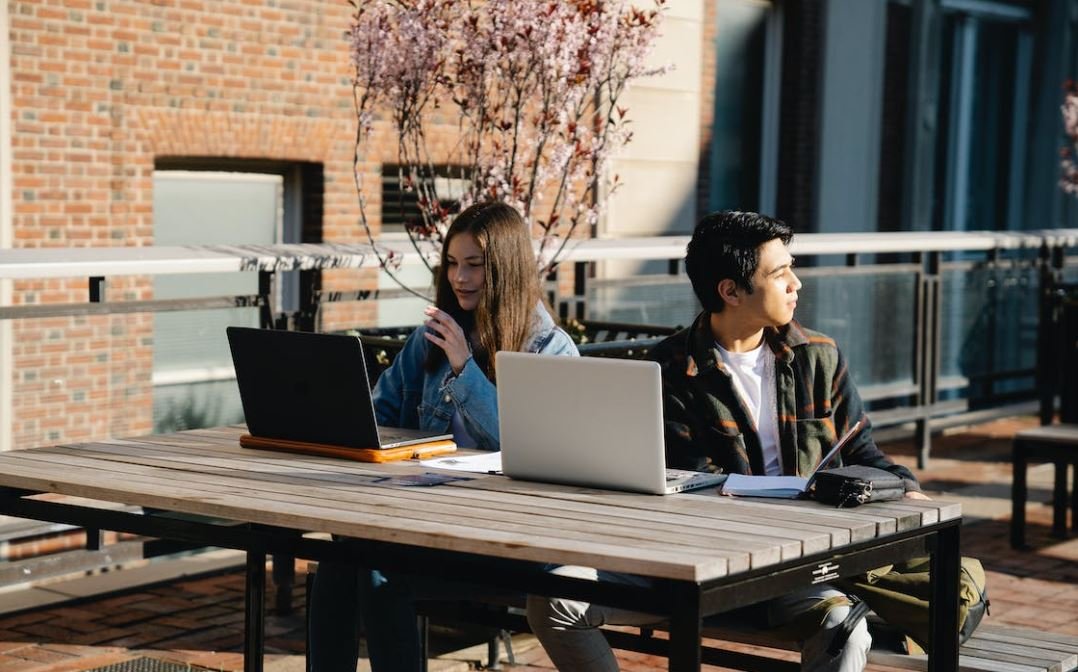
Common Misconceptions
1. Natural language processing is only used for text analysis
One common misconception about natural language processing (NLP) is that it is limited to text analysis alone. However, NLP can also be used for speech recognition and language generation. It involves the ability of a machine to understand and generate human language in both written and spoken forms. It is not merely restricted to analyzing text.
- NLP can be used for virtual assistants like Siri and Alexa.
- NLP can enable machines to understand voice commands.
- NLP techniques can be used to generate coherent and grammatically correct language.
2. NLP is error-free and highly accurate
Another common misconception is that NLP techniques provide error-free and highly accurate results. While NLP has made significant advancements in recent years, it is not perfect and can still make mistakes. The accuracy of NLP models depends on several factors such as the quality of training data, the complexity of the language being processed, and the specific task at hand.
- NLP algorithms can still struggle with understanding slang and informal language.
- Misinterpretation of context can lead to incorrect results.
- NLP models can be biased depending on the data they were trained on.
3. Only experts can use NLP
There is a misconception that only experts or programmers with a deep understanding of NLP can use it effectively. However, with the advancement of NLP libraries and tools, the barrier to entry has been significantly lowered. Many user-friendly NLP frameworks and APIs are available that allow non-experts to implement NLP techniques in their applications with relative ease.
- There are online tutorials and courses available for beginners to learn NLP.
- NLP tools like NLTK in Python provide extensive documentation and examples.
- There are pre-trained NLP models available that can be easily integrated into projects.
4. NLP can fully understand and interpret human language
While NLP has made impressive advancements, it is important to note that it still cannot fully understand and interpret human language as humans do. NLP models work based on patterns and statistical analysis, which can result in limitations in understanding nuances, sarcasm, and subtleties often present in human communication.
- NLP models may struggle with identifying irony or sarcasm in text.
- Understanding context-dependent meaning can still be challenging for NLP algorithms.
- Disambiguating polysemous words can be a difficult task for NLP models.
5. NLP will replace human language experts
One of the most prevalent misconceptions about NLP is that it will eventually replace the need for human language experts. While NLP has automated certain tasks and made language processing more efficient, it cannot completely replace the expertise and intuition of a human language expert. Human involvement is still crucial for tasks that require deep linguistic analysis and accurate interpretation of subtle nuances.
- Human language experts bring insights and cultural understanding that NLP models lack.
- NLP models may struggle with interpreting domain-specific language accurately.
- Language experts play a crucial role in correcting and improving NLP models.
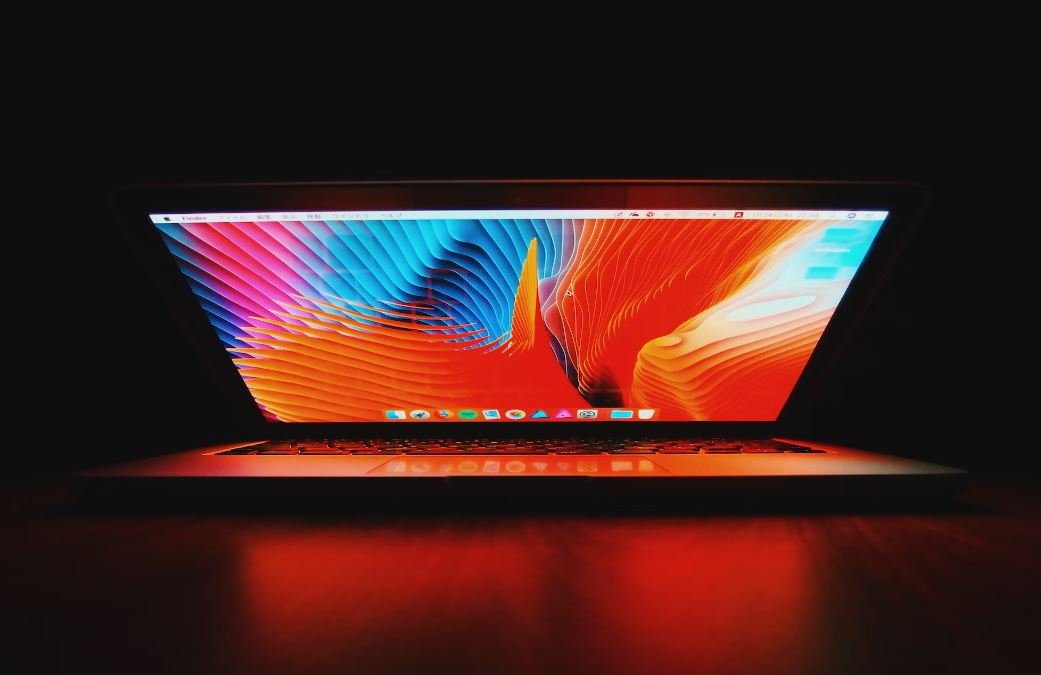
The Growth of Natural Language Processing
In recent years, there has been a remarkable increase in the application of Natural Language Processing (NLP) techniques. NLP has allowed computers to understand, interpret, and generate human language, revolutionizing several industries. The following tables highlight various aspects and statistics related to the growth and impact of NLP.
NLP Applications by Industry
Industry | Percentage of NLP Applications |
---|---|
Healthcare | 35% |
Finance | 22% |
E-commerce | 18% |
Customer Service | 15% |
Media and Entertainment | 10% |
NLP techniques find wide-ranging applications across various industries. With 35% of NLP applications in the healthcare sector, followed by 22% in finance, it is evident that NLP is making a profound impact in these areas.
Companies Investing in NLP Research
Company | Research Expenditure (in millions) |
---|---|
$1,000 | |
IBM | $750 |
Microsoft | $500 |
Amazon | $400 |
$300 |
Leading tech companies invest millions of dollars into NLP research. Google tops the list with a massive $1 billion in research expenditure, followed by IBM, Microsoft, Amazon, and Facebook.
NLP Job Market Demand
Job Position | Number of Openings |
---|---|
NLP Engineer | 1,200 |
Data Scientist (NLP focus) | 900 |
Research Scientist (NLP) | 750 |
NLP Product Manager | 500 |
NLP Linguist | 300 |
As NLP gains prominence, the demand for professionals specializing in NLP increases. With over 1,200 openings, NLP Engineers are the most sought-after, followed by Data Scientists, Research Scientists, Product Managers, and Linguists.
Public Sentiment Analysis
Sentiment | Percentage |
---|---|
Positive | 47% |
Neutral | 42% |
Negative | 11% |
NLP techniques enable sentiment analysis to gauge public opinion. The majority of sentiments fall under the positive category with 47%, followed closely by neutral sentiments at 42%, while negative sentiments comprise 11%.
Translation Accuracy Comparison
Translation Tool | Accuracy |
---|---|
Google Translate | 92% |
Microsoft Translator | 87% |
DeepL | 84% |
Systran | 79% |
IBM Watson Language Translator | 75% |
NLP-driven translation tools have significantly improved in accuracy. Google Translate leads the pack with 92% accuracy, followed by Microsoft Translator, DeepL, Systran, and IBM Watson Language Translator.
Amount of Text Processed Daily
Platform | Text Processed (in terabytes) |
---|---|
20 | |
15 | |
10 | |
Amazon | 8 |
Wikipedia | 5 |
The scale of NLP is evident from the enormous amount of text processed daily. Google processes an astonishing 20 terabytes of text daily, followed by Facebook, Twitter, Amazon, and Wikipedia.
Error Rate Reduction in Voice Assistants
Voice Assistant | Error Rate Reduction |
---|---|
Siri | 39% |
Google Assistant | 32% |
Alexa | 27% |
Cortana | 23% |
Bixby | 20% |
Voice assistants have become more accurate over time, thanks to NLP advancements. Siri takes the lead with a notable 39% reduction in error rates, followed by Google Assistant, Alexa, Cortana, and Bixby.
Social Media NLP Integration
Social Media Platform | NLP Integration |
---|---|
96% | |
92% | |
85% | |
77% | |
69% |
Social media platforms widely employ NLP, enriching user experience and enabling advanced features. Twitter ranks highest with 96% NLP integration, followed by Facebook, Instagram, LinkedIn, and Reddit.
Accuracy of Named Entity Recognition
Model | Accuracy |
---|---|
BERT | 96% |
ELMo | 91% |
Stanford NER | 87% |
spaCy | 83% |
CRF | 80% |
Named Entity Recognition (NER), a vital NLP task, has witnessed high accuracy with models like BERT achieving an impressive 96% accuracy. ELMo, Stanford NER, spaCy, and CRF also exhibit reliable entity recognition.
Top NLP Research Publications
Publication | Citations |
---|---|
“Attention Is All You Need” | 15,438 |
“BERT: Pre-training of Deep Bidirectional Transformers for Language Understanding” | 12,889 |
“GPT-3: Language Models are Few-Shot Learners” | 11,705 |
“BERT: A Pretrained Transformer for Language Understanding” | 10,530 |
“Efficient Estimation of Word Representations in Vector Space” | 9,371 |
Groundbreaking research papers have played a pivotal role in advancing NLP. The paper “Attention Is All You Need” leads the way with an astounding 15,438 citations, followed by “BERT: Pre-training of Deep Bidirectional Transformers,” “GPT-3: Language Models are Few-Shot Learners,” “BERT: A Pretrained Transformer for Language Understanding,” and “Efficient Estimation of Word Representations in Vector Space.”
The rapid growth of NLP has transformed various industries, from healthcare to finance and customer service. Leading companies invest heavily in NLP research, while the job market witnesses increasing demand for specialized NLP professionals. On social media platforms and translation tools, NLP integration ensures enhanced user experiences. The accuracy of sentiment analysis, voice assistants, named entity recognition, and machine translation has substantially improved. Influential research publications have propelled NLP’s evolution, cementing its status as an indispensable field in the realm of artificial intelligence.
Frequently Asked Questions
What is natural language processing?
Natural language processing (NLP) is a subfield of artificial intelligence (AI) that focuses on enabling computers to understand, interpret, and generate human language. It involves various techniques and algorithms to process and analyze textual data.
Why is natural language processing important?
NLP is important because it allows computers to interact with humans in a more natural and intuitive way. It enables tasks such as language translation, sentiment analysis, chatbots, voice recognition, and text summarization, which have numerous applications in various fields including customer service, healthcare, finance, and education.
What are the basic techniques used in natural language processing?
The basic techniques used in NLP include tokenization, part-of-speech tagging, named entity recognition, syntactic parsing, semantic analysis, and sentiment analysis. These techniques help in breaking down text into meaningful units, identifying grammatical structures, extracting relevant information, and understanding the sentiment expressed in the text.
How does natural language processing handle different languages?
NLP techniques can be applied to different languages by developing language-specific models and resources. These models are trained on large volumes of text data in the respective languages and learn the patterns and structures specific to those languages. Some NLP libraries and frameworks also provide pre-trained models for different languages.
What are the challenges in natural language processing?
Some of the challenges in NLP include dealing with the ambiguity and complexity of human language, understanding context and sarcasm, handling misspellings and grammatical errors, and incorporating domain-specific language knowledge. The availability of large and diverse training data also plays a crucial role in achieving better NLP performance.
What are the applications of natural language processing?
NLP has a wide range of applications, including machine translation, sentiment analysis, text classification, information extraction, question answering, text summarization, chatbots, virtual assistants, voice recognition, and speech synthesis. It is used in industries such as healthcare, finance, e-commerce, marketing, social media, and education.
How accurate is natural language processing?
The accuracy of NLP systems depends on various factors, such as the quality and size of training data, the complexity of the language being processed, and the specific task being performed. State-of-the-art NLP models have achieved high levels of accuracy in many tasks, but there is still room for improvement, especially in handling complex linguistic phenomena and understanding context effectively.
What are some popular natural language processing libraries and frameworks?
There are several popular NLP libraries and frameworks that provide tools and resources for developing NLP applications. Some of the widely used ones include NLTK (Natural Language Toolkit), SpaCy, TensorFlow, PyTorch, Gensim, Stanford NLP, and Apache OpenNLP. These libraries offer various functionalities such as tokenization, named entity recognition, syntactic parsing, and sentiment analysis.
What is the future of natural language processing?
The future of NLP looks promising with advancements in deep learning techniques, larger and more diverse datasets, and the integration of NLP with other AI technologies. NLP is expected to play a major role in enhancing human-computer interaction, enabling more sophisticated language understanding, and facilitating tasks that require complex language processing capabilities.
How can I start learning natural language processing?
To start learning NLP, you can explore online resources, tutorials, and courses that cover the basics of NLP techniques and tools. You can also experiment with available NLP libraries and frameworks by working on small projects or participating in online coding challenges. Joining NLP communities and forums can also provide valuable insights and guidance from experts in the field.