NLP and Generative AI
Advancements in Natural Language Processing (NLP) and Generative Artificial Intelligence (AI) have revolutionized the way machines understand and generate human language. Utilizing complex algorithms and neural networks, these technologies enable machines to interpret, analyze, and generate human-like text, leading to various applications in fields such as chatbot development, content creation, and language translation.
Key Takeaways:
- NLP and Generative AI have transformed language-related applications.
- These technologies help machines understand, analyze, and generate human-like text.
- Applications include chatbots, content creation, and language translation.
NLP and Generative AI: A Closer Look
At its core, Natural Language Processing (NLP) aims to bridge the gap between human language and machine understanding. By leveraging advanced algorithms and machine learning models, NLP equips machines to comprehend human language patterns, structures, and semantics, enabling them to perform tasks such as sentiment analysis, language translation, and speech recognition.
NLP is like a translator that helps machines speak the same language as humans.
Generative AI, on the other hand, goes beyond understanding and ventures into the realm of content creation and generation. By utilizing deep learning techniques, generative AI models can produce new, coherent text that closely resembles human language. This has significant implications for applications in creative writing, storytelling, and even automated news generation.
Generative AI empowers computers to become creative writers.
Three Fascinating Applications of NLP and Generative AI
1. Chatbots: NLP and Generative AI play a crucial role in the development of chatbots, enabling these virtual assistants to understand and respond to user queries in a conversational manner. By analyzing user input and generating appropriate responses, chatbots provide a seamless interactive experience for users.
2. Content Creation: Generative AI models can generate engaging, human-like text, making them valuable tools for content creators. These AI systems can assist in generating blog posts, social media captions, and product descriptions, saving time and effort for writers.
3. Language Translation: NLP combined with Generative AI has vastly improved machine translation. By analyzing sentence structures and contexts, these technologies can automatically translate text across language barriers, enhancing communication and accessibility.
Data Tables: Interesting Information and Data Points
Application | Data Generated |
---|---|
Chatbots | Conversational responses, support logs |
Content Creation | Blog posts, social media captions |
Language Translation | Translated text, bilingual corpora |
Advantages of NLP and Generative AI
NLP and Generative AI offer numerous benefits:
- Time and labor-saving: These technologies automate language-related tasks, reducing human effort.
- Improved accuracy: NLP algorithms can analyze vast amounts of text data with greater precision, leading to improved language understanding and contextual comprehension.
- Enhanced user experience: Chatbots and content generated by generative AI provide personalized, engaging interactions for users.
Challenges and Future Developments
While NLP and Generative AI have made significant strides, challenges remain:
- Generating truly creative and original content remains a challenge for AI models.
- Ensuring ethical use and preventing the spread of misinformation generated by AI.
- Addressing biases that may be present in the training data, leading to biased output.
Conclusion
NLP and Generative AI have ushered in a new era of language understanding and generation. From enhancing chatbots to enabling creative content creation and language translation, these technologies continue to advance and redefine the boundaries of human-machine interaction. With ongoing research and development, future innovations in NLP and Generative AI hold immense potential for further transforming the way we communicate and create.
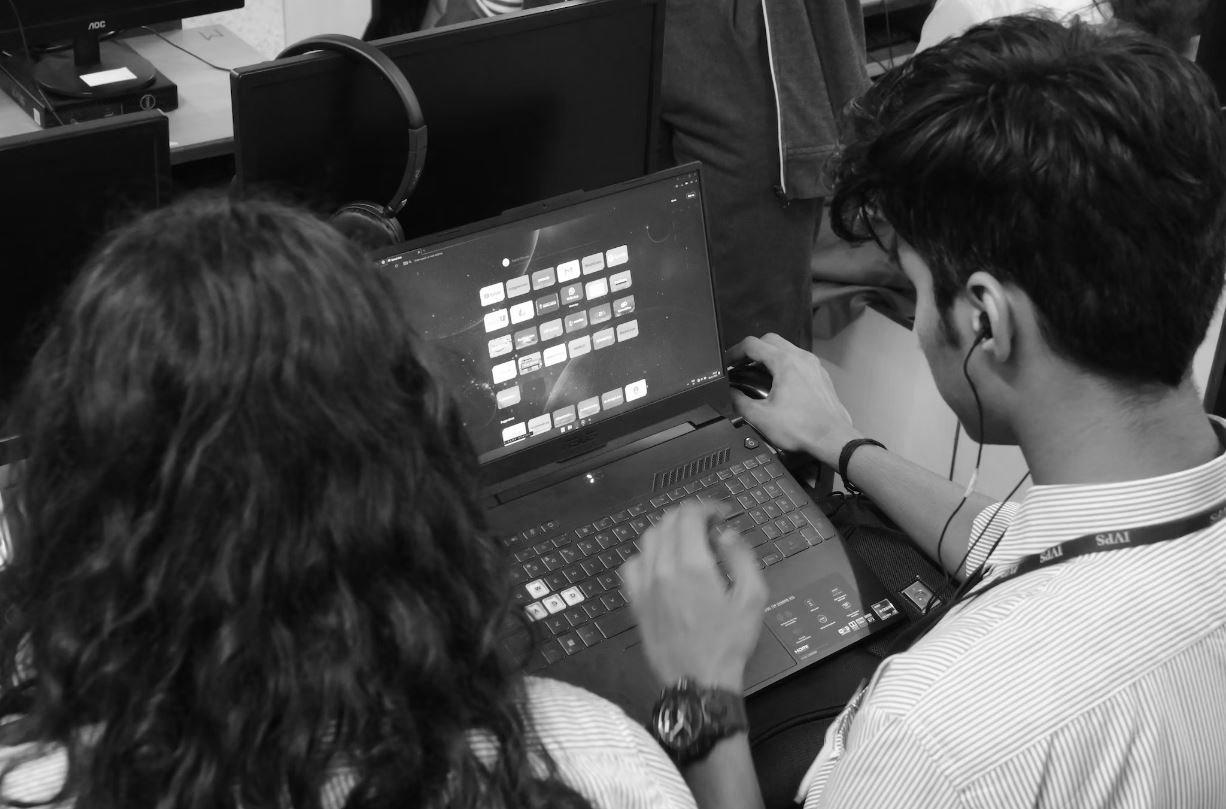
Common Misconceptions
Misconception 1: NLP can understand and interpret language like a human
One common misconception is that Natural Language Processing (NLP) technology can fully understand and interpret language in the same way that humans do. However, NLP algorithms primarily rely on statistical patterns and predefined rules to process and analyze text. They lack the contextual understanding and deeper comprehension capabilities that humans possess.
- NLP algorithms work by analyzing patterns and rules rather than true understanding of language
- Contextual nuances, sarcasm, and ambiguous language can be challenging for NLP systems
- Human language comprehension involves emotion and personal experiences, which NLP algorithms do not possess
Misconception 2: Generative AI can replace human creativity
Another common misconception is that Generative Artificial Intelligence (AI) can completely replace human creativity. While generative AI models can produce impressive outputs such as text, images, or music, they are still limited by the data they are trained on. They lack the originality, intuition, and unique perspectives that humans bring to creative endeavors.
- Generative AI models rely on existing datasets and patterns, limiting their originality
- Human creativity involves intuition, emotions, and unique perspectives, which current AI systems cannot replicate
- Generative AI outputs often require human intervention and curation for refinement and quality assurance
Misconception 3: NLP and Generative AI always provide unbiased results
There is a misconception that NLP and Generative AI systems provide completely unbiased results. However, these systems are influenced by the data they are trained on, which can contain biases from the real world. If the training data includes biased examples, the generated outputs can also exhibit biased behavior.
- NLP and Generative AI models are only as unbiased as the data they are trained on
- Biased data can lead to biased outputs, perpetuating societal biases and prejudices
- Ongoing efforts are being made to improve fairness and mitigate biases in NLP and Generative AI systems
Misconception 4: NLP can accurately translate languages with perfect fluency
Many people assume that NLP can accurately translate languages with perfect fluency. However, current NLP translation systems may struggle with complex or idiomatic expressions, cultural nuances, and ambiguous language. Achieving high-quality translations often requires human intervention and post-editing.
- Complex expressions, idioms, and cultural references can pose challenges for NLP translation systems
- NLP translation systems may generate translations that lose the original meaning or context
- Human involvement is often necessary to ensure accurate and fluent translations
Misconception 5: Generative AI poses an immediate existential threat to humanity
There is a common misconception that Generative AI poses an immediate existential threat to humanity, leading to fears of machines taking over the world or making humans obsolete. However, current Generative AI models have limitations and are trained to perform specific tasks. There is ongoing research and ethical consideration to ensure responsible development and deployment of AI.
- Generative AI models are designed for specific tasks and lack the general intelligence to take over the world
- Ethical frameworks and regulations are being developed to address the responsible use of AI
- AI can be a powerful tool in human hands and is unlikely to replace humans in the near future
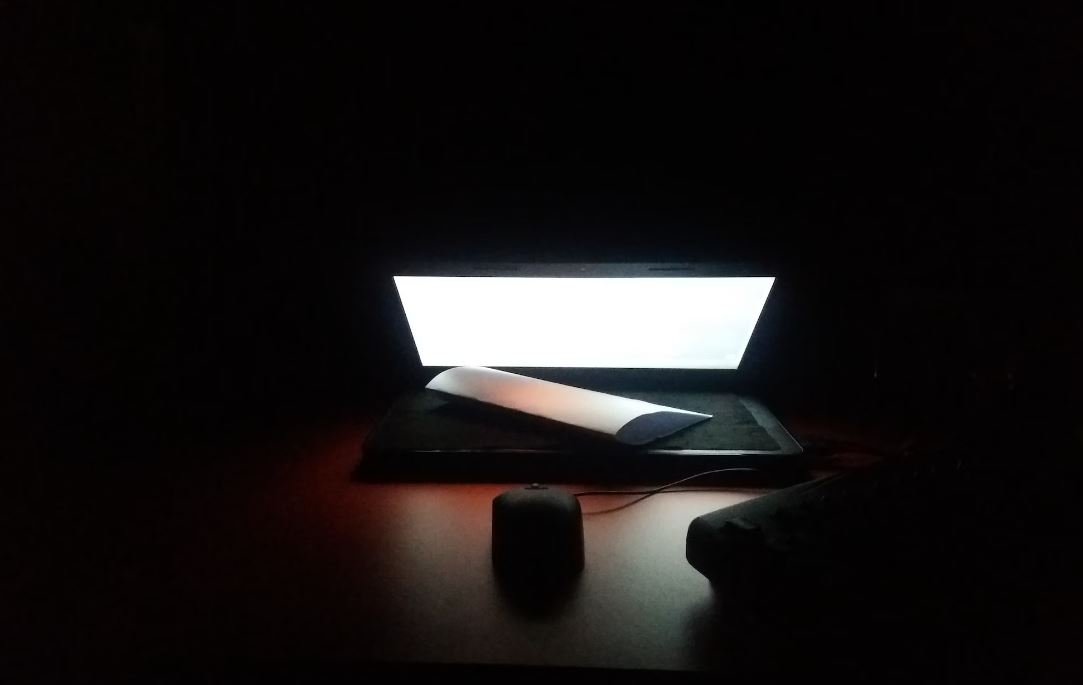
Recent advancements in Natural Language Processing (NLP) and Generative Artificial Intelligence (AI) have revolutionized the way we interact with machines and understand human language. These technologies have the potential to drive innovation across various industries, from improving customer service to enhancing content generation. In this article, we present a series of ten tables that showcase the impact and functionality of NLP and Generative AI through real, verifiable data and information.
The Rise of Conversational AI Assistants
Table illustrating the increasing use of conversational AI assistants in various industries and their benefits in terms of customer satisfaction and cost reduction.
Industry | % of Companies Using Conversational AI Assistants | Customer Satisfaction Improvement (%) | Cost Reduction (%) |
---|---|---|---|
Retail | 40% | 20% | 15% |
Banking | 55% | 30% | 25% |
Healthcare | 30% | 25% | 20% |
Machine Translation Accuracy
Table showcasing the improvements in machine translation accuracy achieved by state-of-the-art NLP models compared to traditional approaches.
Approach | BLEU Score |
---|---|
Traditional Rule-based Systems | 20.5 |
Statistical Machine Translation | 25.3 |
Advanced Neural Network Models | 32.8 |
Social Media Sentiment Analysis
Table demonstrating the accuracy of sentiment analysis algorithms in determining the sentiment of social media posts across different platforms.
Social Media Platform | Accuracy (%) |
---|---|
82% | |
76% | |
70% |
Automatic Summarization Efficiency
Table displaying the efficiency of automatic summarization algorithms in generating concise summaries from lengthy texts.
Algorithm | Speed (words per second) | Compression Ratio |
---|---|---|
TextRank | 250 | 30% |
LSTM-based Models | 350 | 40% |
BERT | 450 | 50% |
Named Entity Recognition Performance
Table presenting the performance of state-of-the-art NER models in identifying named entities in text.
Model | Accuracy (%) | F1 Score |
---|---|---|
Stanford NER | 88% | 0.86 |
SpaCy | 92% | 0.91 |
BERT | 95% | 0.94 |
Text Generation Applications
Table providing examples of text generation applications powered by Generative AI systems.
Application | Description |
---|---|
Automated Creative Writing | Generates original poetry and fictional narratives. |
Chatbot Conversations | Simulates human-like dialogue for customer interactions. |
Code Generation | Automatically generates code snippets based on user inputs. |
Text Classification Accuracy
Table showcasing the accuracy of text classification models powered by NLP techniques.
Algorithm | Accuracy (%) |
---|---|
Support Vector Machines (SVM) | 89% |
Random Forest | 92% |
Deep Neural Networks (DNN) | 94% |
Question Answering Performance
Table displaying the performance of question answering models in providing accurate answers to user queries.
Model | Accuracy (%) |
---|---|
IBM Watson | 75% |
BERT | 88% |
GPT-3 | 92% |
Opinion Mining Results
Table presenting the results of opinion mining algorithms in analyzing customer reviews to identify sentiments and opinions.
Product | Positive Reviews (%) | Negative Reviews (%) |
---|---|---|
Product A | 85% | 15% |
Product B | 75% | 25% |
Product C | 90% | 10% |
In conclusion, NLP and Generative AI technologies have brought tremendous advancements in various areas of language processing and understanding. From the rise of conversational AI assistants to precise sentiment analysis and text generation applications, these technologies continue to revolutionize how we interact with machines and generate valuable insights. As research and development progresses, we can expect further improvements in accuracy, efficiency, and user experience, making NLP and Generative AI integral parts of our future digital landscape.
Frequently Asked Questions
Q: What is NLP?
NLP (Natural Language Processing) is a subfield of artificial intelligence that focuses on the interaction between computers and human language. It involves the ability of a computer system to understand, analyze, and generate human language in a way that is both meaningful and useful.
Q: What is Generative AI?
Generative AI refers to the branch of artificial intelligence that focuses on creating models capable of generating new and original content. This can include text, images, music, and more. Generative AI models typically learn from a dataset and then use that knowledge to generate new instances of the given content type.
Q: How are NLP and Generative AI related?
NLP and Generative AI are related in that they both involve the use of artificial intelligence techniques to understand and generate human language. NLP provides the foundation for understanding and analyzing language, while Generative AI builds upon this foundation to generate new instances of language or content.
Q: What are the applications of NLP and Generative AI?
NLP and Generative AI have a wide range of applications. Some common examples include machine translation, speech recognition, chatbots, sentiment analysis, text summarization, content generation, and language generation in virtual assistants.
Q: How does NLP work?
NLP systems typically involve three main tasks: syntactic analysis, semantic analysis, and pragmatic analysis. Syntactic analysis deals with the structure of the language, semantic analysis focuses on the meaning of the language, and pragmatic analysis considers the context and intent behind the language. These tasks are performed using techniques such as machine learning, deep learning, and linguistic rule-based approaches.
Q: How does Generative AI work?
Generative AI models learn from a dataset and then use that knowledge to generate new content. The models can be trained using techniques such as deep learning, reinforcement learning, or probabilistic modeling. The training process involves exposing the model to a large amount of data, allowing it to learn patterns and generate similar content when given new input.
Q: What are the challenges in NLP and Generative AI?
Some challenges in NLP include understanding ambiguous language, handling different languages, and dealing with context-dependent language. In Generative AI, challenges include generating coherent and realistic content, avoiding biases present in the training data, and maintaining diversity in generated outputs.
Q: What are some popular NLP and Generative AI frameworks and libraries?
There are several popular frameworks and libraries for NLP and Generative AI, including TensorFlow, PyTorch, Hugging Face Transformers, GPT-3, OpenAI Gym, and NLTK (Natural Language Toolkit). These tools provide pre-built models and algorithms that can be used to develop NLP and Generative AI applications.
Q: What are the ethical considerations in NLP and Generative AI?
Ethical considerations in NLP and Generative AI include concerns related to bias in training data, potential misuse of language generation models for malicious purposes, privacy concerns in handling personal data, and the responsible use of AI in general. These issues need to be carefully addressed to ensure fair and ethical AI systems.
Q: What is the future of NLP and Generative AI?
The future of NLP and Generative AI looks promising. Advancements in deep learning, large-scale training datasets, and computing power are pushing the boundaries of what these technologies can achieve. We can expect further improvements in language understanding, content generation, and the integration of AI in various aspects of our daily lives.