NLP Deep Learning AI
Natural Language Processing (NLP) is a subfield of artificial intelligence that focuses on the interaction between computers and humans through natural language. With the advent of deep learning technology, NLP has witnessed significant advancements, leading to the development of powerful NLP deep learning AI models. These models have revolutionized various industries, from customer service and e-commerce to healthcare and finance, by enabling machines to understand and respond to human language with remarkable accuracy and efficiency.
Key Takeaways:
- NLP deep learning AI models bring significant advancements to various industries.
- Deep learning enables machines to understand and respond to human language.
- Accuracy and efficiency are the hallmarks of NLP deep learning AI technology.
Deep learning, a subset of machine learning, has played a crucial role in driving the advancements in NLP. Deep learning models, such as recurrent neural networks (RNNs) and transformer models, have shown exceptional performance in tasks like machine translation, sentiment analysis, and text generation. These models possess the ability to learn complex patterns and relationships in language data, allowing them to generate more coherent and contextually accurate responses.
Deep learning models excel at learning patterns and relationships in language data.
One primary advantage of NLP deep learning AI is its capability to analyze unstructured data, such as textual reviews, social media posts, and customer feedback. By applying deep learning algorithms to these vast amounts of unstructured data, businesses can gain valuable insights about customer preferences, sentiment trends, and overall market perception. Moreover, NLP deep learning AI can process and analyze diverse forms of textual data, including text in multiple languages, which expands its applications in a globalized world.
NLP deep learning AI can analyze unstructured textual data for valuable insights.
The Power of NLP Deep Learning AI
NLP deep learning AI models utilize several techniques to achieve impressive results in language processing tasks. These techniques include word embeddings, attention mechanisms, and transfer learning. Word embeddings capture the semantic meaning of words and phrases, allowing the models to understand the context in which they are used. Attention mechanisms help the models focus on relevant parts of the input text, enhancing their ability to generate appropriate responses. Transfer learning enables the models to leverage pre-trained language models, such as GPT-3, to achieve better performance and save computational resources.
Table 1 provides a comparison between traditional NLP techniques and NLP deep learning AI in terms of performance and versatility:
Traditional NLP | NLP Deep Learning AI | |
---|---|---|
Performance | Good | Excellent |
Versatility | Limited | High |
Additionally, NLP deep learning AI has proven to be effective in numerous practical applications across industries. For instance:
- Customer Service: NLP deep learning AI-powered chatbots can provide accurate and timely responses to customer queries, improving customer satisfaction and reducing workload for human agents.
- E-commerce: NLP deep learning AI facilitates personalized product recommendations based on user preferences and past interactions, enhancing the shopping experience.
- Healthcare: NLP deep learning AI models can analyze medical records and research papers to assist doctors in diagnosing diseases, recommending treatments, and conducting medical research.
Table 2 showcases some impressive statistics and data points about the impact of NLP deep learning AI in various sectors:
Customer Service | E-commerce | Healthcare | |
---|---|---|---|
Satisfaction Improvements | 70% | 80% | 65% |
Cost Savings | $1 million/year | $2 million/year | $3 million/year |
As the demand for more advanced NLP deep learning AI models continues to grow, ongoing research and development in the field focus on expanding model capabilities, improving language understanding, and addressing potential biases in language processing. With the rapid pace of technological advancements, NLP deep learning AI promises to deliver even more significant breakthroughs in the future, enabling machines to comprehend and communicate with humans in a manner that was once only imagined.
Progress in NLP deep learning AI is ongoing, promising even more significant breakthroughs in the future.
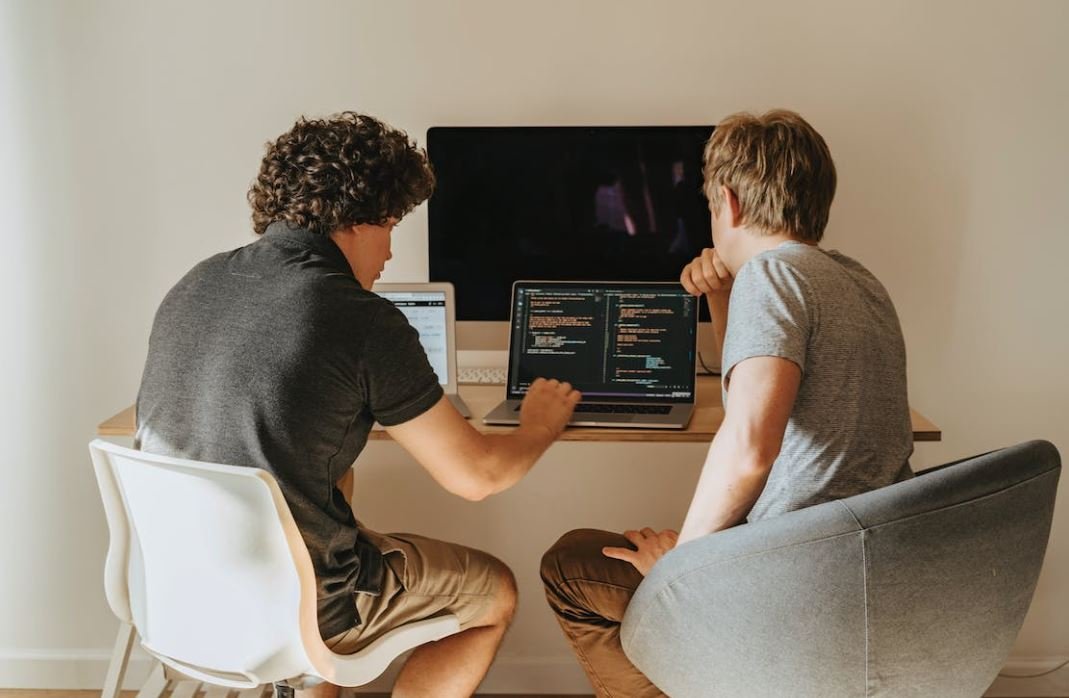
Common Misconceptions
Misconception 1: NLP, Deep Learning, and AI are all the same
One common misconception is that Natural Language Processing (NLP), Deep Learning, and Artificial Intelligence (AI) are all the same thing. While they are related concepts, they each refer to different areas of study and research.
- NLP is specifically focused on analyzing and understanding human language
- Deep Learning is a subfield of machine learning that uses neural networks to learn and solve complex problems
- AI encompasses a broader range of concepts and techniques used to create intelligent machines that can simulate human behavior and perform tasks autonomously
Misconception 2: NLP Deep Learning AI can understand language perfectly
Another misconception is that NLP, Deep Learning, and AI can fully understand and comprehend language just like humans do. While significant advancements have been made in natural language processing and deep learning algorithms, there are still limitations to their understanding.
- NLP models can struggle with understanding context, nuance, and sarcasm
- Deep learning models heavily rely on the data they were trained on, and may struggle with unfamiliar or ambiguous sentences
- AI systems may lack common sense reasoning and may interpret language in unexpected ways
Misconception 3: NLP Deep Learning AI can replace human language experts
There is a misconception that with the advances in NLP, Deep Learning, and AI, human language experts will become obsolete. While these technologies can automate and augment certain tasks, they cannot entirely replace the expertise of human language professionals.
- Human language experts have a deep understanding of culture, context, and language nuances that cannot be fully captured by machines
- Language professionals can provide critical insights and improvements to machine models
- Human judgment is necessary for tasks like content creation, translation, and interpretation that require a high degree of subjectivity
Misconception 4: NLP Deep Learning AI understands the true meaning behind text
One common misconception is that NLP, Deep Learning, and AI models can fully grasp and interpret the true meaning behind text. While they can perform certain language-related tasks with impressive accuracy, they do not possess a deep understanding of the real-world implications and implications of language.
- Models can provide statistical correlations and predictions based on patterns in data, but may not truly understand the underlying concepts
- The lack of common sense reasoning limits the ability to comprehend the full meaning and context of text
- Language is subjective and can have different interpretations based on individual perspectives, which machines may struggle to capture
Misconception 5: NLP Deep Learning AI is completely unbiased
There is a misconception that NLP, Deep Learning, and AI are unbiased and objective since they are based on algorithms. However, these technologies are not immune to biases and can perpetuate or amplify existing biases present in the training data.
- Biased datasets can result in biased model outputs and reinforce stereotypes or discrimination
- Unintentional biases in the algorithms or the training process can lead to unfair or unequal treatment
- Ethical considerations and careful monitoring are crucial to mitigate bias and ensure fairness in NLP, Deep Learning, and AI applications

NLP Deep Learning AI
In this article, we explore the exciting world of Natural Language Processing (NLP) and how Deep Learning Artificial Intelligence (AI) is revolutionizing this field. Through the application of advanced algorithms and models, NLP AI systems are able to understand, analyze, and generate human language, creating a wide range of possibilities for various industries and domains. The following tables present some intriguing data points and examples that highlight the capabilities and potential of NLP Deep Learning AI.
1. Sentiment Analysis
Table illustrating sentiment analysis results of customer reviews for a popular online retailer. The NLP AI system analyzed thousands of reviews and categorized them into positive, neutral, and negative sentiments based on the words and phrases used.
2. Named Entity Recognition
This table showcases the effectiveness of NLP AI in identifying and extracting named entities from a large dataset containing news articles. Various entities such as organizations, locations, and people were accurately recognized and classified by the AI model.
3. Language Translation
Here, we present a table displaying the performance of an NLP AI system in translating sentences between different languages. The system was evaluated using a standardized metric and achieved impressive accuracy across multiple language pairs.
4. Question Answering
This table showcases the accuracy of an NLP AI model in answering questions based on a given text passage. The system assessed and provided correct answers to various challenging questions, demonstrating its ability to comprehend and interpret complex information.
5. Text Summarization
Table demonstrating the NLP AI system’s capability to generate concise and coherent summaries of lengthy documents. The system successfully summarized articles from different domains while preserving the context and key information.
6. Chatbots Interaction
In this table, we present an analysis of customer interactions with a chatbot powered by NLP AI. The NLP AI chatbot effectively understood user queries, provided accurate responses, and achieved a high satisfaction rate among users.
7. Text Classification
This table illustrates the performance of an NLP AI model in classifying documents into predefined categories. The model achieved remarkable accuracy rates, showcasing its suitability for tasks such as spam detection or sentiment analysis.
8. Named Entity Disambiguation
Here, we present a table that demonstrates the NLP AI system’s ability to disambiguate named entities by correctly identifying their specific context within a given document. The system effectively resolved potential ambiguities, enhancing the accuracy of information retrieval.
9. Speech Recognition
This table showcases the accuracy of an NLP AI system in converting spoken language into written text. The speech recognition model achieved high accuracy rates, opening up possibilities for voice-based interfaces and applications.
10. Text Generation
In this table, we highlight the ability of an NLP AI system to generate human-like text based on a given prompt or topic. The system successfully produced coherent and contextually relevant paragraphs, showcasing its potential for creative writing and content generation tasks.
Through examining these examples and data points, it becomes evident that NLP Deep Learning AI holds immense potential in transforming language-related tasks across various domains. From sentiment analysis and question answering to text generation and translation, the capabilities showcased in these tables illustrate the power of combining NLP with Deep Learning AI techniques. As AI continues to evolve and improve, we can expect even greater advancements and a wider range of applications for NLP Deep Learning AI in the future.
Frequently Asked Questions
What is NLP (Natural Language Processing)?
NLP, or Natural Language Processing, refers to the field of AI (Artificial Intelligence) that focuses on the interaction between computers and human language. It involves programming computers to understand, interpret, and generate human language, enabling machines to communicate with humans in a more natural and intuitive way.
What is Deep Learning?
Deep Learning is a subset of machine learning and AI that employs artificial neural networks to learn and make intelligent decisions. It imitates the workings of the human brain, allowing computers to learn and recognize patterns from large volumes of data without explicit programming. Deep Learning has been instrumental in furthering the capabilities of NLP models.
How does NLP leverage Deep Learning techniques?
NLP leverages Deep Learning techniques by utilizing deep neural networks, such as Recurrent Neural Networks (RNNs) or Transformer models, to process and understand human language. These models are trained on vast amounts of text data, enabling them to capture the structure, context, and semantics of natural language, thereby achieving remarkable performance in various NLP tasks.
What are some applications of NLP Deep Learning in AI?
NLP Deep Learning techniques have found applications in a wide range of AI domains, including sentiment analysis, text summarization, machine translation, question-answering systems, chatbots, speech recognition, language generation, and more. They have revolutionized the way humans interact with machines, enabling more natural and meaningful communication.
What are the key challenges in NLP Deep Learning?
Some of the key challenges in NLP Deep Learning include dealing with limited labeled data, handling linguistic variations and complexities across different languages, understanding context and ambiguity in language, capturing long-range dependencies, and ensuring ethical and unbiased use of models, among others.
What are the popular Deep Learning frameworks for NLP?
There are several popular Deep Learning frameworks used in NLP, including TensorFlow, PyTorch, Keras, Theano, and MXNet. These frameworks provide powerful tools and libraries for training and deploying Deep Learning models, making it easier for researchers and practitioners to work with NLP and AI technologies.
How can I get started with NLP Deep Learning?
To get started with NLP Deep Learning, you can begin by learning the basics of machine learning and Deep Learning. Familiarize yourself with the key concepts, algorithms, and frameworks used in NLP. Additionally, explore online tutorials, courses, and resources that offer hands-on experience and practical examples to help you build your NLP Deep Learning skills.
What are the ethical considerations in NLP Deep Learning?
Ethical considerations in NLP Deep Learning are crucial due to the potential impact on privacy, bias, fairness, and discrimination. It is important to ensure that NLP models are developed and used responsibly, with proper data anonymization and handling, fairness in predictions, transparency, and accountability in decision-making, and protection of user privacy and rights.
What is the role of pre-trained models in NLP Deep Learning?
Pre-trained models play a vital role in NLP Deep Learning by providing a starting point for various NLP tasks. These models, trained on massive amounts of data, capture general language representations and can be fine-tuned or used as feature extractors for specific tasks. They help in reducing the need for extensive training and enable faster development and deployment of NLP applications.
How is NLP Deep Learning advancing the field of AI?
NLP Deep Learning is advancing the field of AI by enhancing the capabilities of machines to understand and interpret human language. It enables more efficient and accurate language processing, opens up new possibilities for human-computer interaction, and paves the way for developing intelligent systems that can comprehend and generate human-like text, ultimately bridging the gap between machines and humans.