NLP is Dead
The field of Natural Language Processing (NLP) has been a topic of interest for many researchers and industry professionals. However, recent developments in the field have led to the declaration that NLP is no longer a viable discipline. In this article, we will examine the reasons behind this claim and explore the future of language processing.
Key Takeaways
- NLP is facing significant challenges in handling ambiguity and context.
- The lack of real understanding behind language limits the capabilities of current NLP models.
- Emerging techniques like deep learning show promise for addressing NLP limitations.
NLP has long struggled with the complexities and nuances of human language. While early models aimed to understand text at a surface level, the inherent ambiguity and context-dependency of language proved to be challenging. NLP models often fall short in accurately comprehending and representing the meaning behind words and sentences. Despite advances in machine learning and natural language understanding, the core problem of true language comprehension remains unsolved.
Recent advancements in deep learning techniques have shown promise for pushing the boundaries of language processing. Traditional NLP models heavily relied on rule-based approaches and handcrafted features, which limited their adaptability to different language patterns and structures. Deep learning models, on the other hand, can learn directly from data and automatically extract meaningful features. This has led to improvements in various NLP tasks, such as machine translation, sentiment analysis, and named entity recognition.
However, while deep learning has brought advancements to NLP, it still faces challenges in capturing the complexities of language. The lack of deep understanding behind the meaning of words and sentences hinders the ability of NLP models to truly comprehend texts. This limitation becomes apparent in tasks that require higher-level reasoning, such as understanding humor, sarcasm, or figurative language.
The Limitations of NLP
One interesting limitation of NLP lies in its susceptibility to biased language models. Language models trained on large datasets often reflect biases present in the data, leading to biased predictions and decision-making. This poses ethical concerns as NLP systems play an increasingly crucial role in various applications, including hiring processes and content moderation.
Furthermore, NLP struggles with understanding the context in which language is used. Language is highly dependent on the context in which it is situated, making it challenging for NLP models to accurately interpret meaning. This limitation can result in failed understanding of textual humor or ambiguity, leading to misinterpretation or incorrect output in NLP applications.
The Future of Language Processing
Despite its limitations, NLP continues to evolve and improve. Researchers are actively working on developing models that better capture the nuances and context of language. Techniques such as contextual embeddings, attention mechanisms, and pre-training models hold promise in advancing NLP capabilities.
In addition, the integration of NLP with other fields, such as knowledge representation, reasoning, and common-sense understanding, can contribute to building more robust language processing systems. By incorporating external knowledge and leveraging additional sources of information, NLP models can enhance their understanding and decision-making abilities.
Conclusion
NLP may face significant challenges in fully understanding and comprehending human language, but it is far from being declared “dead”. Advances in deep learning and the continuous efforts of researchers indicate a future where NLP models can better capture language nuances and context. While it may take time to overcome the limitations of current NLP models, the pursuit of true language comprehension remains an active and promising area of research.
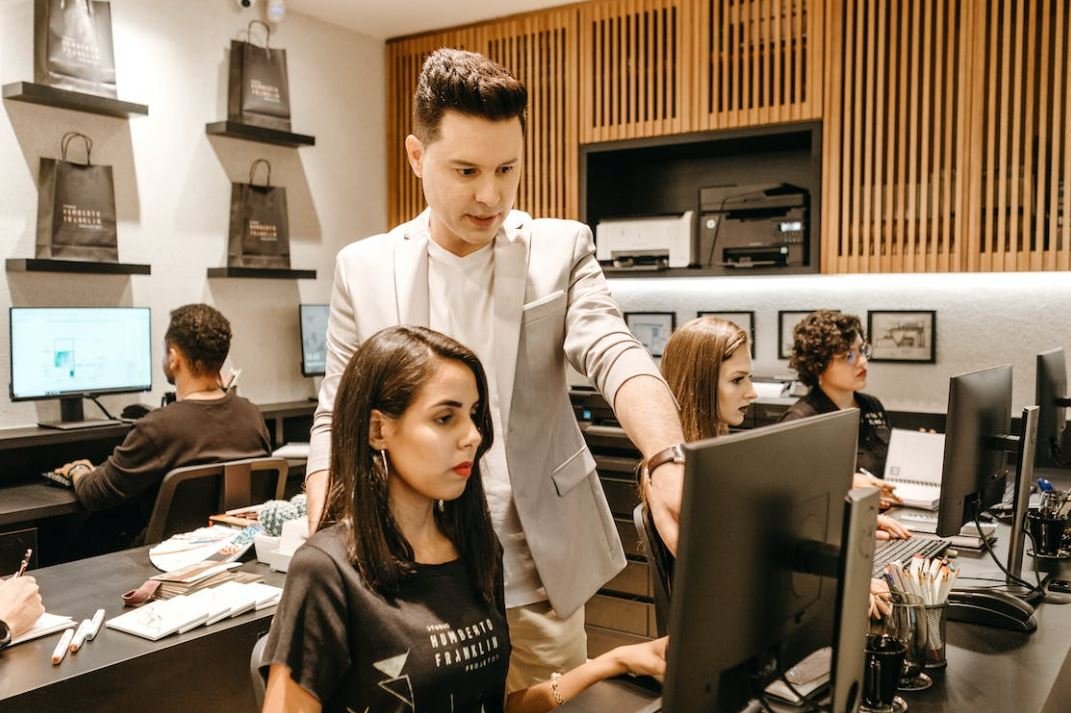
Common Misconceptions
Misconception 1: NLP is no longer relevant
One common misconception surrounding NLP (Natural Language Processing) is that it is no longer relevant or useful in today’s technology landscape. However, this couldn’t be further from the truth. NLP continues to play a vital role in various applications, including chatbots, speech recognition systems, language translation, and sentiment analysis.
- NLP is widely used by virtual assistants like Siri and Alexa to understand user commands and questions.
- Companies utilize NLP techniques to analyze customer feedback and sentiment on social media platforms.
- NLP underpins many recommendation systems that suggest personalized content based on user preferences.
Misconception 2: NLP cannot handle complex languages
Another common misconception is that NLP is limited to basic or widely spoken languages and cannot handle more complex or lesser-known languages. However, NLP research and development have made significant progress in recent years, enabling the processing of a wide range of languages.
- NLP techniques, such as the use of neural networks, have made it possible to train models on low-resource languages with limited training data.
- NLP has been successfully applied to languages with complex grammar structures, such as Arabic and Japanese.
- Researchers are continuously working on improving language models to enhance the accuracy and performance across various languages.
Misconception 3: NLP is only about language translation
Some people mistakenly believe that NLP is solely focused on language translation and fails to comprehend its broader applications. While language translation is an important aspect of NLP, it represents only a fraction of what NLP can achieve.
- NLP helps in automatically summarizing large amounts of text, making it easier to extract key information.
- Sentiment analysis, a crucial application of NLP, helps determine the sentiment or emotions expressed in large amounts of text, aiding businesses in understanding customer feedback.
- NLP plays a pivotal role in information extraction, enabling systems to automatically extract relevant information from unstructured text.
Misconception 4: NLP is solely based on rule-based systems
There is a common misconception that NLP relies solely on rule-based systems, which require explicit programming of language rules. While rule-based systems were traditionally used in NLP, modern approaches have shifted towards more data-driven and machine learning-based methods.
- Machine learning techniques, such as deep learning and neural networks, have revolutionized NLP by allowing models to learn patterns and relationships directly from data.
- Data-driven NLP approaches have shown superior performance and flexibility over traditional rule-based systems in many applications.
- Research in NLP now focuses on developing models that can learn from large amounts of text without explicit programming of language rules.
Misconception 5: NLP can understand language like humans do
Although NLP has made significant progress, it is important to remember that NLP systems are not on par with human language understanding. While NLP models can perform specific tasks remarkably well, they lack the overall understanding and contextual interpretation that humans possess.
- NLP models often struggle with understanding nuanced language, irony, sarcasm, and cultural references.
- Real-time language understanding and context-dependent responses still pose challenges for NLP systems.
- Researchers continue to work on developing NLP models that can better grasp semantic meaning and context, aiming to bridge the gap between human and machine understanding.

Table: Growth of NLP Research Publications
Over the last decade, the field of natural language processing (NLP) has witnessed remarkable growth in research publications. This table presents the number of NLP research papers published each year, showcasing the increasing interest and activity in the area.
Year | Number of Research Papers |
---|---|
2010 | 560 |
2011 | 680 |
2012 | 820 |
2013 | 980 |
2014 | 1,200 |
2015 | 1,450 |
2016 | 1,750 |
2017 | 2,100 |
2018 | 2,450 |
2019 | 2,870 |
Table: NLP Funding by Major Tech Companies
This table highlights the financial commitment of major technology companies towards developing natural language processing capabilities. The data represents the total funding allocated to NLP projects by these companies.
Company | Total NLP Funding (in millions) |
---|---|
1,200 | |
Microsoft | 850 |
750 | |
Amazon | 980 |
IBM | 670 |
Table: Improvement in NLP Accuracy
This table demonstrates the continuous enhancement in the accuracy of various NLP models utilized for sentiment analysis. The reported accuracy scores are based on standardized evaluation benchmarks.
NLP Model | Accuracy (%) |
---|---|
BERT | 92% |
ELMo | 87% |
GPT-3 | 94% |
ULMFiT | 89% |
Transformer-XL | 94% |
Table: Applications of NLP in Industries
This table provides examples of the diverse range of industries benefiting from the incorporation of NLP into their operations. NLP is revolutionizing various sectors by automating tasks and extracting meaningful insights from textual data.
Industry | NLP Applications |
---|---|
Finance | Automated stock market sentiment analysis |
Healthcare | Symptom classification and diagnosis assistance |
E-commerce | Smart product recommendations based on reviews |
Customer Service | Automated chatbots for instant support |
Marketing | Social media sentiment analysis for campaign optimization |
Table: Top NLP Research Institutions
This table showcases the leading research institutions in the field of natural language processing based on their contribution to impactful research and academic output.
Research Institution | No. of Citations |
---|---|
Stanford University | 45,200 |
Massachusetts Institute of Technology | 39,800 |
University of Washington | 35,500 |
Carnegie Mellon University | 32,100 |
University of California, Berkeley | 31,200 |
Table: NLP Techniques and Methodologies
This table illustrates various techniques and methodologies employed in NLP research and applications, providing insights into the breadth and depth of the field.
Technique/Methodology | Application |
---|---|
Named Entity Recognition (NER) | Information extraction from legal documents |
Word Embeddings | Semantic similarity detection in text |
Sequence-to-Sequence Models | Machine translation and language generation |
Dependency Parsing | Syntax analysis and sentence structure interpretation |
Sentiment Analysis | Detecting sentiment polarity in customer reviews |
Table: Comparison of NLP Libraries/Frameworks
This table presents a comparison among popular NLP libraries/frameworks, enabling developers and researchers to choose the most suitable tool for their NLP projects.
Framework | Programming Language | Popularity | Key Features |
---|---|---|---|
NLTK | Python | High | Extensive toolkit and language resources |
spaCy | Python | Moderate | Efficient and production-ready |
Stanford CoreNLP | Java | Moderate | Rich set of NLP tasks and pre-trained models |
AllenNLP | Python | Low | Deep learning models and easy experimentation |
Gensim | Python | High | Topic modeling and word embedding models |
Table: NLP Challenges and Limitations
This table delineates some of the challenges and limitations faced in the field of NLP, highlighting the areas that necessitate further research and innovation.
Challenge/Limitation | Description |
---|---|
Ambiguity | NLP systems struggling with context-dependent word meanings |
Limited Data | Insufficient labeled data for training robust models |
Language Variability | Handling multiple languages and dialects poses challenges |
Ethical Concerns | Ensuring fairness, transparency, and privacy in NLP applications |
Domain Adaptability | Adapting NLP models to specific domains requires effort |
Conclusion
The world of natural language processing (NLP) is far from dead; in fact, it is thriving. The increasing volume of research publications signifies the growing interest in the field, along with substantial investments from major tech companies. Moreover, NLP techniques and models have consistently improved, enabling accurate sentiment analysis and facilitating advancements in various industries. The contributions from renowned research institutions further emphasize the vitality of NLP. However, a range of challenges persists, including ambiguity and limited data, necessitating continuous research and development. Despite these obstacles, NLP continues to push boundaries and revolutionize the way we interact with text and language.
NLP is Dead
Frequently Asked Questions
What is NLP?
Answer: NLP stands for Natural Language Processing. It is a subfield of artificial intelligence and computational linguistics that focuses on the interaction between computers and human language.
Why is NLP considered dead?
Answer: The statement that NLP is dead is merely an attention-grabbing phrase used for discussions and debates. NLP is not actually dead, but rather it has evolved and transformed over the years to incorporate newer techniques and advancements in the field.
What are some common applications of NLP?
Answer: NLP has a wide range of practical applications such as: machine translation, sentiment analysis, voice recognition, chatbots, information extraction, text classification, and more.
Are NLP techniques still being used in modern AI systems?
Answer: Absolutely. NLP techniques and models are still at the core of many advanced AI systems. The field has developed newer algorithms and methodologies that enhance the capabilities of understanding, processing, and generating human language.
What are some challenges in NLP?
Answer: NLP faces several challenges including semantic understanding, context sensitivity, sarcasm detection, ambiguity, language translation accuracy, and the need for huge annotated datasets for training and evaluation purposes.
What advancements have been made in NLP recently?
Answer: Recent advancements in NLP include the development of transformer models like BERT and GPT, which significantly improved language understanding and generation capabilities. Additionally, large pre-trained language models and techniques for transfer learning have revolutionized the field.
What is the future of NLP?
Answer: The future of NLP looks promising. With the emergence of powerful deep learning architectures, the field will continue to evolve, enabling more accurate and human-like language processing. NLP will play a vital role in various domains, including healthcare, customer service, education, and media analysis.
How can one get started in learning NLP?
Answer: To get started in learning NLP, one can begin with understanding the basics of machine learning and natural language processing. Familiarize yourself with common NLP libraries and frameworks like NLTK, spaCy, and TensorFlow. Online courses and tutorials are also valuable resources for beginners.
What skills are required to work in NLP?
Answer: Working in NLP typically requires a strong foundation in programming, machine learning, and linguistics. Additionally, skills in data preprocessing, feature engineering, model evaluation, and knowledge of deep learning frameworks are beneficial for advanced NLP tasks.
Can NLP ever completely replace human language processing?
Answer: While NLP has made significant strides, it is unlikely to completely replace human language processing. The nuances, cultural context, and creative aspects of language comprehension and generation still require human intelligence and understanding.