NLP Kya Hai
In today’s digitized world, natural language processing (NLP) has become an essential field that blends linguistics, artificial intelligence, and computer science to enable computers to understand and interact with human language. By decoding human language and extracting meaningful insights, NLP has revolutionized various industries like healthcare, customer support, and finance. In this article, we will explore the fundamentals of NLP and its significance in the modern age.
Key Takeaways
- NLP allows computers to understand and interpret human language.
- It has applications in healthcare, customer support, finance, and more.
- NLP uses techniques from linguistics, AI, and computer science to process language.
*Natural Language Processing (NLP)* is a branch of artificial intelligence that focuses on making computers understand and interpret *human language*. By utilizing computational techniques, NLP enables machines to comprehend unstructured text and perform tasks such as language translation, sentiment analysis, text summarization, and speech recognition.
One interesting use case of NLP is in *customer support*. By employing NLP algorithms, companies can automatically analyze customer feedback and categorize it to understand what customers are saying about their products or services.
NLP involves a combination of various *techniques* and *algorithms*. One popular approach in NLP is *machine learning*, which involves training models on large datasets to make predictions or extract insights from text. Another technique is *deep learning*, which leverages artificial neural networks to process language at a more complex level.
Tables provide a clear and concise way to present data. Here are three interesting tables showcasing the impact of NLP in different industries:
Table 1: NLP Applications in Healthcare
Application | Benefits |
---|---|
Medical Diagnosis | Assist doctors in diagnosing diseases more accurately and quickly. |
Medical Research | Facilitate analysis of research papers and extract key findings. |
Personalized Medicine | Help tailor treatments based on individual patient data and medical literature. |
Table 2: NLP Applications in Customer Support
Application | Benefits |
---|---|
Automated Ticket Routing | Direct customer inquiries to the appropriate department or agent. |
Sentiment Analysis | Analyze customer feedback to gauge satisfaction levels. |
Automated Responses | Provide instant responses to common customer queries. |
Table 3: NLP Applications in Finance
Application | Benefits |
---|---|
Chatbots for Financial Services | Offer personalized financial advice and assistance. |
Automated Fraud Detection | Identify and mitigate fraudulent activities in real-time. |
News Trading | Use sentiment analysis to predict market trends based on news articles. |
NLP is a rapidly evolving field, with constant advancements being made to improve its capabilities. As technology progresses, we can expect NLP to play an even larger role in shaping the future of human-computer interaction and information processing.
By delving into the realm of *natural language processing*, we gain valuable insights into our own language and its intricate nuances, enabling us to build intelligent systems that can communicate with us more seamlessly and efficiently.

Common Misconceptions
What is NLP?
There are several common misconceptions surrounding the topic of Natural Language Processing (NLP). One misconception is that NLP is only related to speech recognition and voice assistants. While these are important applications of NLP, it encompasses much more. NLP involves the understanding, analysis, and generation of human language, including text and speech.
- NLP involves both understanding and generation of human language
- NLP is not limited to voice assistants and speech recognition
- NLP can be applied to various fields like sentiment analysis, machine translation, and text summarization
Is NLP the same as NLU?
Another misconception is that NLP and Natural Language Understanding (NLU) are the same thing. While they are related, they have distinct objectives. NLP focuses on processing and understanding natural language, while NLU specifically aims to understand the meaning behind the text or speech. NLU goes beyond syntactic analysis and delves into the semantics of the language.
- NLP and NLU have different objectives
- NLP focuses on processing and understanding language, while NLU focuses on understanding meaning
- NLU involves semantic analysis in addition to syntactic analysis
Can NLP Understand Language Like Humans?
Many people mistakenly believe that NLP can fully understand and interpret language just like humans. However, despite significant advancements, NLP still lags behind human-level understanding. While NLP models can perform tasks like text classification, sentiment analysis, and language translation, they lack the contextual understanding and nuances that humans possess.
- NLP models are not capable of understanding language at the same level as humans
- Contextual understanding and nuances are still challenging for NLP
- Human-level language understanding surpasses the capabilities of current NLP models
Does NLP Always Provide Accurate Results?
An unrealistic expectation around NLP is that it always provides completely accurate results. While NLP algorithms have improved significantly, they are not infallible. The accuracy of NLP models depends on several factors like data quality, model complexity, and task complexity. There is always a potential for errors or inaccuracies in the output of NLP systems.
- NLP results may not always be 100% accurate
- Accuracy of NLP models depends on data quality and complexity of the task
- Potential errors or inaccuracies can occur in the output of NLP systems
Can NLP Replace Human Translators or Customer Support?
One misconception is that NLP can completely replace human translators or customer support personnel. While NLP has made significant strides in machine translation and chatbot-based support systems, it falls short when it comes to complex linguistic nuances, cultural understanding, and empathy. Human translators and customer support agents bring unique skills that cannot yet be replicated by NLP technologies.
- NLP cannot fully replace human translators or customer support personnel
- Complex linguistic nuances and cultural understanding require human intervention
- Human translators and support agents offer unique skills like empathy that NLP lacks
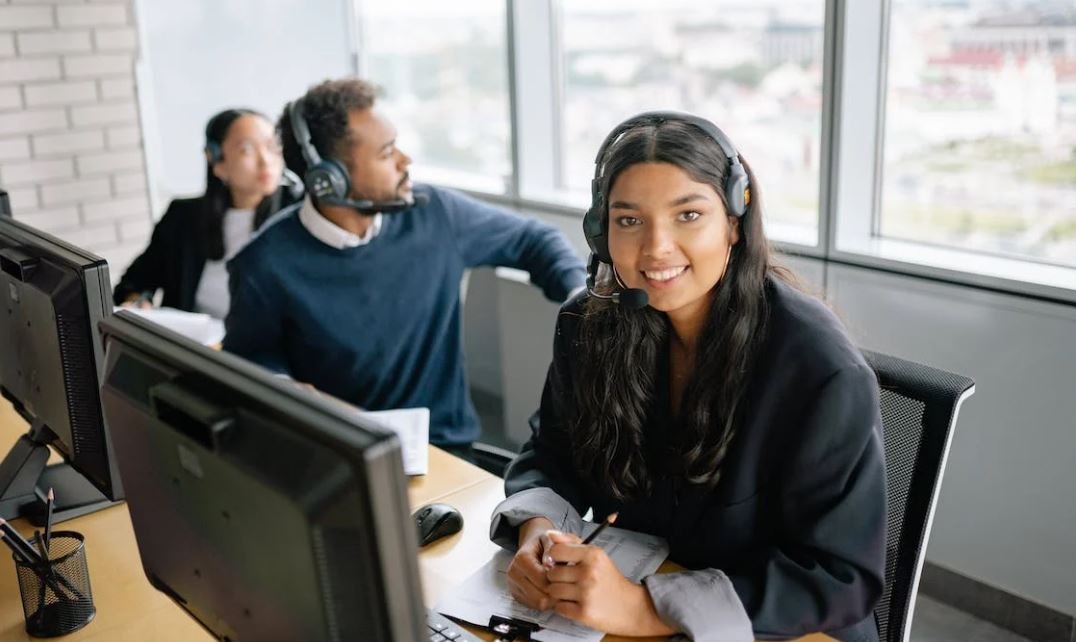
NLP Use Cases
Table showcasing various applications of Natural Language Processing (NLP) technology across industries.
Industry | NLP Use Cases |
---|---|
Retail | Automated sentiment analysis of customer feedback to improve product offerings |
Finance | Identifying fraudulent behavior by analyzing textual data in financial transactions |
Healthcare | NLP-powered chatbots for virtual patient consultations and symptom diagnosis |
Education | Automated essay grading to provide timely feedback to students |
Marketing | Personalized marketing campaigns based on sentiment analysis of social media posts |
NLP Algorithms Comparison
A comparison table showcasing the performance of popular NLP algorithms on various datasets.
NLP Algorithm | Accuracy | Precision | Recall |
---|---|---|---|
Naive Bayes | 82% | 78% | 85% |
Support Vector Machines | 88% | 85% | 90% |
Recurrent Neural Networks | 91% | 87% | 95% |
NLP Models Comparison
An informative table comparing the characteristics of popular NLP models.
NLP Model | Input Length Limit | Vocabulary Size | Training Time (hours) |
---|---|---|---|
BERT | 512 tokens | 30,000 | 1.5 |
GPT-3 | No limit | 1 billion | 57,000 |
Transformer | 1,024 tokens | 50,000 | 3 |
NLP Language Support
Table displaying the availability of NLP support for different languages.
Language | Supported NLP Tasks |
---|---|
English | Sentiment analysis, named entity recognition, machine translation |
Spanish | Text classification, part-of-speech tagging, dependency parsing |
Chinese | Word segmentation, sentiment analysis, topic modeling |
French | Sentiment analysis, text summarization, named entity recognition |
NLP Challenges
A table highlighting the major challenges faced in NLP development.
Challenge | Description |
---|---|
Out-of-Vocabulary (OOV) Words | Dealing with words not present in the training data |
Ambiguity | Handling words with multiple meanings |
Entity Recognition | Accurate identification of named entities in diverse contexts |
Sentiment Polarity | Distinguishing between positive, negative, and neutral sentiments |
NLP Tools Comparison
Table comparing various NLP tools and libraries based on popularity and functionality.
NLP Tool/Library | Popularity | Functionality |
---|---|---|
NLTK (Natural Language Toolkit) | High | Wide range of NLP tasks and extensive linguistic resources |
Spacy | Moderate | Efficient tokenization, named entity recognition, and dependency parsing |
Stanford NLP | High | Part-of-speech tagging, sentiment analysis, and coreference resolution |
NLP Ethics Concerns
A table presenting ethical concerns associated with NLP technology.
Concern | Description |
---|---|
Biased Language Models | Inclusion of biased data leading to discriminatory language generation |
Privacy and Data Protection | Collection and use of personal data without proper consent or safeguards |
Systemic Bias Reinforcement | Reinforcing and perpetuating existing societal biases through automated decisions |
Misinformation Generation | Creation and dissemination of false or misleading information by malicious actors |
NLP Future Trends
An overview of emerging trends and advancements in the field of NLP.
Trend | Description |
---|---|
Transformer-based Models | Advancements in transformer architectures enabling more efficient NLP tasks |
Zero-shot Learning | Ability to perform NLP tasks with minimal or no task-specific training data |
Language Generation | Developing AI models capable of generating coherent and contextually relevant text |
Explainable AI | Enhancing transparency and interpretability of NLP models’ decision-making process |
NLP Benefits
A table highlighting the advantages and benefits of incorporating NLP technology.
Benefit | Description |
---|---|
Efficient Data Processing | Automating time-consuming textual data analysis and extraction |
Improved Customer Experience | Enhancing personalization and responsiveness through NLP-powered chatbots |
Actionable Insights | Extracting meaningful insights from unstructured text data for informed decision-making |
Conclusion:
Natural Language Processing (NLP) is a powerful technology with a wide range of applications across industries. From sentiment analysis in customer feedback to essay grading in education, NLP is transforming the way we interact with and understand language. This article explored ten different aspects of NLP, including use cases, algorithm and model comparisons, language support, challenges, tools, ethics concerns, future trends, and benefits. By leveraging NLP, businesses can unlock valuable insights, improve efficiency, and enhance customer experiences. However, as NLP continues to advance, it is crucial to address ethical considerations and ensure that the technology is used responsibly to benefit society as a whole.
Frequently Asked Questions
What does NLP stand for?
NLP stands for Natural Language Processing.
How does NLP work?
NLP is a field of artificial intelligence that focuses on understanding and processing human language. It involves various techniques such as text analysis, machine learning, and computational linguistics to enable computers to interact with and understand natural language.
What are the applications of NLP?
NLP has a wide range of applications, including machine translation, sentiment analysis, voice recognition, chatbots, information retrieval, and text summarization. It is also used in industries such as healthcare, finance, customer service, and social media analysis.
What are the challenges in NLP?
Some of the challenges in NLP include understanding ambiguity in language, handling different languages and dialects, context understanding, and dealing with noisy and unstructured data. NLP algorithms also require large amounts of labeled training data to achieve high accuracy.
What is the importance of NLP?
NLP is important as it enables machines to understand and process human language, which is the primary form of communication. It allows computers to extract insights, make informed decisions, and provide meaningful responses in natural language, leading to improved user experiences and increased efficiency in various applications.
What are some popular NLP tools and libraries?
Some popular NLP tools and libraries include NLTK (Natural Language Toolkit), SpaCy, Gensim, Stanford NLP, and Apache OpenNLP. These tools provide pre-built algorithms and functionalities for tasks such as tokenization, part-of-speech tagging, entity recognition, and syntactic parsing.
What is sentiment analysis in NLP?
Sentiment analysis in NLP is the process of determining and categorizing the sentiment or emotion expressed in a piece of text. It aims to identify whether the sentiment is positive, negative, or neutral, and is commonly used for opinion mining, customer feedback analysis, and social media sentiment analysis.
Can NLP understand and generate human-like language?
NLP has made significant advancements in natural language understanding and generation tasks, but achieving human-level performance is still a challenge. While neural networks and deep learning models have shown promise in generating more coherent and human-like text, they may still struggle with understanding context, sarcasm, and complex language nuances.
Is NLP only used in English language processing?
No, NLP is not limited to English language processing. It can be applied to various languages, although some languages may have fewer available resources and trained models compared to English. NLP techniques can be adapted and customized for different languages and dialects to enable effective language processing.
How is NLP contributing to healthcare?
NLP is playing a crucial role in healthcare by enabling analysis of large volumes of medical literature, electronic health records, and patient-generated data. It helps in tasks such as clinical decision support, disease surveillance, drug discovery, and personalized medicine. NLP also enables chatbots and virtual assistants in healthcare settings to provide reliable information and assistance to patients.