NLP Vision Meaning
Natural Language Processing (NLP) is a subfield of artificial intelligence (AI) focused on the interactions between computers and humans through natural language. With the rapid advancements in NLP, one particular area of interest is NLP Vision, which aims to enable computers to understand and interpret visual information, transforming the way we interact with technology.
Key Takeaways:
- NLP Vision encompasses the integration of natural language processing and computer vision technologies.
- It enables computers to process and extract meaningful information from images and videos.
- NLP Vision has applications in various industries, including healthcare, retail, and surveillance.
- Advancements in deep learning algorithms have significantly improved the accuracy and performance of NLP Vision models.
- NLP Vision has the potential to revolutionize areas such as image recognition, object detection, and visual understanding.
Understanding NLP Vision
NLP Vision combines natural language processing techniques with computer vision to understand and interpret visual information. This interdisciplinary field focuses on extracting meaning from images and videos using advanced machine learning algorithms and deep neural networks. By teaching machines to recognize objects, understand scenes, and interpret emotions conveyed by facial expressions, NLP Vision enables computers to process visual data and interact with the world in a more human-like manner.
One interesting aspect of NLP Vision is its ability to caption images. By generating descriptive captions for images, computers gain a deeper understanding of the visual content. This capability opens up numerous possibilities in areas such as autonomous vehicles, content moderation, and image indexing, where computers can automatically analyze and interpret vast amounts of visual data.
The Applications of NLP Vision
NLP Vision technology has wide-ranging applications across various industries:
- Healthcare: NLP Vision can assist in medical imaging analysis, helping doctors in diagnosing and treating diseases by quickly analyzing X-rays, CT scans, and MRI images.
- Retail: Visual search and recommendation systems powered by NLP Vision can enhance the online shopping experience, allowing users to find and purchase products based on visual similarity or specific attributes.
Industry | Application |
---|---|
Healthcare | Medical imaging analysis |
Retail | Visual search and recommendation systems |
3. Surveillance: NLP Vision can improve security systems by identifying suspicious activities, recognizing faces, and providing real-time alerts based on visual data.
Industry | Application |
---|---|
Surveillance | Enhanced security systems |
Advancements in NLP Vision
Recent advancements in deep learning algorithms and the availability of large datasets have propelled the progress of NLP Vision. These advancements have led to breakthroughs in areas such as:
- Image recognition: NLP Vision models can accurately identify objects, scenes, and even fine-grained details within images.
- Object detection: NLP Vision algorithms can detect and locate objects within images or videos, enabling applications like autonomous driving and surveillance.
- Visual understanding: NLP Vision techniques allow machines to comprehend and interpret the content of images, making them capable of answering questions about visual data.
The Future of NLP Vision
With ongoing advancements, the potential of NLP Vision is immense. By unlocking the ability to process and understand visual data, computers can assist in various tasks, revolutionizing industries and improving our daily lives. As research continues and technology progresses, NLP Vision will continue to shape the future of human-computer interaction and pave the way for new applications we cannot yet imagine.
Remember, the possibilities with NLP Vision are boundless.
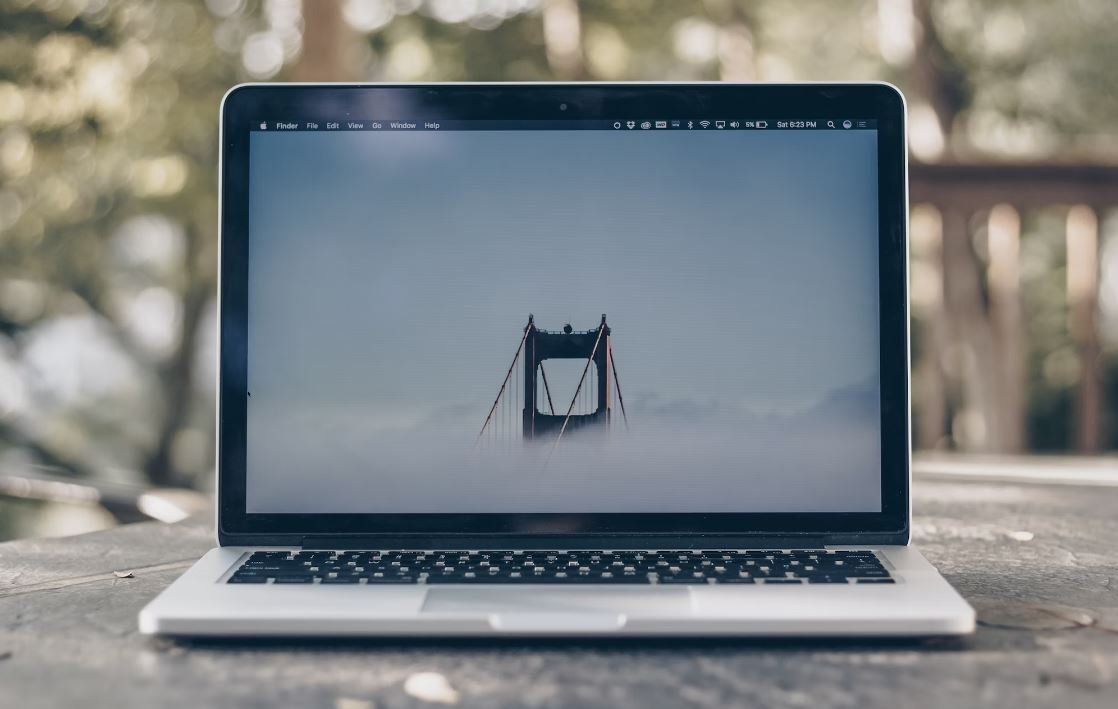
Common Misconceptions
Misconception 1: NLP is the same as natural language understanding
One common misconception about NLP is that it is the same as natural language understanding (NLU). While NLU is a subfield of NLP, NLP encompasses a broader range of techniques and applications. NLP focuses on the interaction between computers and natural languages, encompassing tasks such as language generation, sentiment analysis, and machine translation.
- NLP is not limited to understanding and processing text
- NLP includes tasks beyond language understanding, such as language generation
- NLU is a specific subset of NLP
Misconception 2: NLP can perfectly understand human language
Another common misconception is that NLP can perfectly understand and interpret human language. While NLP has made significant advancements in understanding and processing language, it still faces challenges in handling nuances, context, sarcasm, and ambiguity. NLP models are trained on large datasets and can learn patterns, but they are not infallible in comprehending the complexities of human language.
- NLP struggles with understanding contextual cues
- Handling ambiguous language is a challenge for NLP
- NLP models may not be able to interpret sarcasm effectively
Misconception 3: NLP can replace human translators
Many people believe that NLP technology, such as machine translation, can fully replace human translators. While NLP has significantly improved machine translation capabilities, it still falls short when compared to human translation. Human translators have cultural and contextual knowledge that allows them to produce more accurate and nuanced translations, especially for complex texts.
- Human translators possess cultural and contextual knowledge
- NLP machine translation may produce inaccurate or unnatural translations
- Complex texts may require human translation for accuracy
Misconception 4: NLP can read and understand text like humans do
There is a misconception that NLP can read and understand text like humans do. While NLP models can process and analyze large amounts of text, their understanding is based on statistical patterns rather than true comprehension. NLP models lack common sense reasoning, background knowledge, and the ability to infer information that is not explicitly stated in the text.
- NLP relies on statistical patterns rather than true comprehension
- NLP models lack common sense reasoning
- NLP cannot infer information not explicitly stated in the text
Misconception 5: NLP can replace human interaction and customer support
Some people believe that NLP can fully replace human interaction and customer support services. While NLP-based chatbots and virtual assistants have become popular, they still have limitations in understanding complex queries, handling emotions, and providing empathetic responses. Human interaction and customer support are essential for resolving complex issues and providing personalized experiences.
- NLP-based chatbots may struggle to understand complex queries
- NLP lacks the ability to provide empathetic human responses
- Human interaction is often needed for resolving complex issues
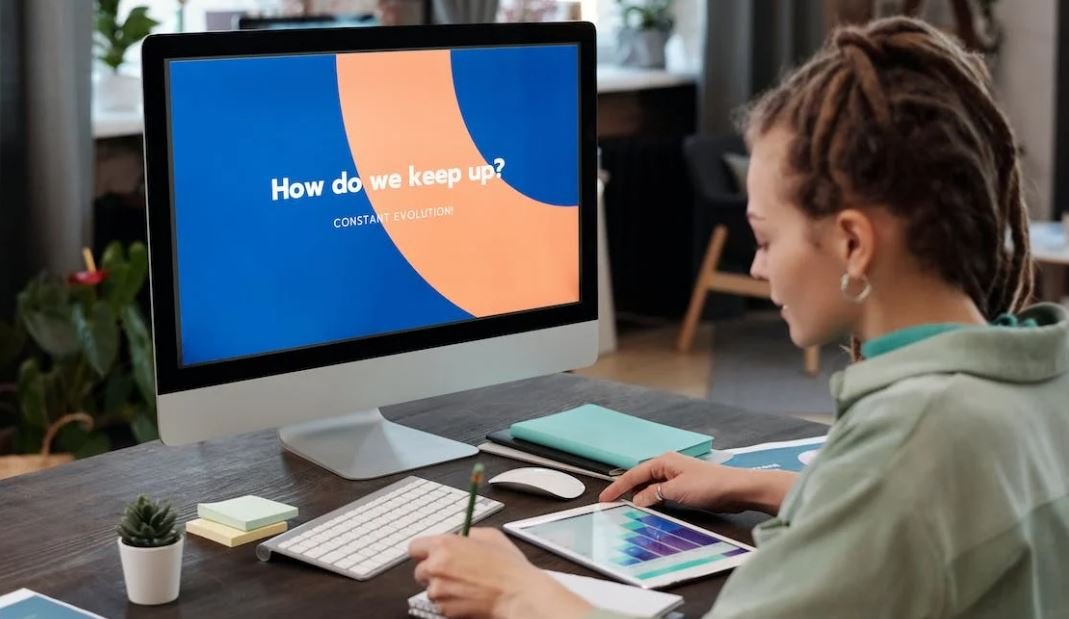
Introduction
In this article, we will explore various aspects of NLP (Natural Language Processing) and its significance in understanding the meaning behind textual data. Through the following tables, we will delve into different dimensions of NLP, including language processing techniques, applications, and the impact of NLP on various industries.
Table: Most Common NLP Techniques
Table displaying the most commonly used NLP techniques utilized in language processing tasks.
Technique | Description |
---|---|
Tokenization | Splits text into individual words, phrases, or symbols. |
Part-of-Speech Tagging | Labels each word with a grammatical tag. |
Sentiment Analysis | Determines the emotional tone of a text. |
Named Entity Recognition | Identifies named entities such as names, locations, etc. |
Table: Applications of NLP
Table highlighting the diverse range of applications that benefit from NLP techniques.
Industry | Application |
---|---|
Healthcare | Automated diagnosis and medical record analysis. |
E-commerce | Product recommendation systems based on customer reviews. |
Finance | Sentiment analysis for stock market prediction. |
Social Media | Automated content moderation and sentiment monitoring. |
Table: Impact of NLP in Various Industries
Table showcasing the transformative impact of NLP techniques in different industries.
Industry | Benefit |
---|---|
Customer Service | Improved chatbot interactions and automated responses. |
Education | Enhanced language learning tools and personalized feedback. |
Legal | Efficient document review for legal cases. |
Media | Automated summarization and content extraction. |
Table: Popular NLP Libraries and Frameworks
An informative table presenting widely used NLP libraries and frameworks with their respective descriptions.
Library/Framework | Description |
---|---|
NLTK | A Python library for NLP tasks, providing extensive tools and resources. |
spaCy | An efficient and user-friendly NLP library for various language processing tasks. |
TensorFlow | A popular deep learning framework for NLP applications. |
PyTorch | A flexible deep learning framework widely used in NLP research. |
Table: Common Challenges in NLP
A table outlining the key challenges faced in NLP tasks and language processing.
Challenge | Description |
---|---|
Ambiguity | The multiple interpretations of words or sentences. |
Out-of-vocabulary Words | Words or phrases not present in the language model’s vocabulary. |
Context Understanding | The ability to comprehend the contextual meaning of text. |
Domain Adaptation | Transferring linguistic knowledge across different domains. |
Table: NLP Performance Evaluation Metrics
A comprehensive table presenting the evaluation metrics used to measure the performance of NLP models.
Metric | Description |
---|---|
Accuracy | The ratio of correctly predicted outcomes to the total number of predictions. |
Precision | The proportion of true positives among the predicted positives. |
Recall | The proportion of true positives among the actual positives. |
F1-Score | The harmonic mean of precision and recall. |
Table: Ethical Considerations in NLP
A table highlighting ethical considerations surrounding NLP and language processing techniques.
Consideration | Description |
---|---|
Privacy | Respecting confidentiality and securing user data. |
Bias | Addressing potential biases in the language models and datasets used. |
Disinformation | Combatting the spread of fake news and disinformation. |
Transparency | Ensuring interpretability and transparency of NLP models. |
Table: Future Trends in NLP
A captivating table highlighting potential future trends and advancements in the field of NLP.
Trend | Description |
---|---|
Zero-shot Learning | Training models to generalize to unseen tasks or domains. |
Multilingual NLP | Developing models capable of understanding and translating multiple languages. |
Explainable AI | Achieving better interpretability and explainability of NLP models. |
Lifelong Learning | Enabling models to continually learn and adapt over time. |
Conclusion
NLP plays a pivotal role in unlocking the meaning within textual data, revolutionizing various industries and domains. Through this exploration of NLP techniques, applications, challenges, and ethical considerations, it is evident that NLP has immense potential to transform the way we interact with language. As advancements continue and new trends emerge, NLP will drive innovations and further bridge the gap between human language and machine understanding, empowering a wide range of practical applications.
Frequently Asked Questions
What is NLP and how does it relate to vision?
Natural Language Processing (NLP) is a field of study that focuses on the interaction between computers and human language. It involves programming computers to process and analyze large amounts of textual data. Vision, on the other hand, deals with image processing and computer vision tasks. NLP and vision can be combined to create systems that understand and interpret both text and images.
How does NLP help in understanding the meaning of visual content?
NLP techniques can be used to analyze textual context associated with visual content. By processing text descriptions, captions, or surrounding text, NLP can provide insights into the meaning and intent of visual content. By combining NLP with computer vision algorithms, we can build systems that have a better understanding of visual content and its semantic context.
What are some real-world applications of NLP and vision?
NLP and vision can be applied in various domains. Some examples include:
- Automated image captioning
- Visual question answering
- Image sentiment analysis
- Brand monitoring through social media image analysis
- Automatic video summarization
- Visual search
What are the challenges in NLP and vision integration?
Integrating NLP and vision introduces several challenges, such as:
- Handling ambiguity in visual and textual data
- Extracting meaningful information from unstructured data
- Training large-scale models to process both text and images
- Creating efficient methods for cross-modal information retrieval
- Addressing privacy concerns in analyzing visual and textual content
How can NLP and vision be used for content moderation?
NLP and vision can aid in content moderation by automatically flagging and filtering inappropriate or harmful visual and textual content. By analyzing text and image data, NLP algorithms can detect hate speech, nudity, violence, and other types of content violations. This can help in maintaining safe and respectful online environments.
What is the role of machine learning in NLP and vision?
Machine learning plays a crucial role in NLP and vision tasks. It involves training models that can learn from data and make predictions or perform various tasks. In the context of NLP and vision, machine learning algorithms are used to train models capable of understanding and interpreting language and visual content from the input data.
How can I get started with NLP and vision?
To get started with NLP and vision, you can:
- Learn programming languages such as Python and libraries like TensorFlow or PyTorch
- Study machine learning and deep learning concepts
- Explore NLP and computer vision literature and research papers
- Practice with open-source NLP and vision datasets and code repositories
- Participate in online courses or tutorials on NLP and vision
What are some popular NLP and vision research projects?
There are several noteworthy research projects in the field of NLP and vision. Some examples include:
- Google’s Multimodal Transformer for multimodal tasks
- Facebook’s DenseCap for generating dense image captions
- OpenAI’s CLIP for cross-modal understanding
- Microsoft Research’s Deep Image Captioning for generating image descriptions
- Stanford’s Visual Question Answering dataset and challenge (VQA)
What are the ethical considerations in NLP and vision applications?
As with any technology, NLP and vision applications raise ethical considerations. Some key considerations are:
- Privacy and security concerns related to analyzing personal visual and textual content
- Biases in algorithms that may lead to unfair or discriminatory outcomes
- Transparency in how NLP and vision systems make decisions
- Ensuring responsible and ethical use of NLP and vision technologies