NLP Vs Generative AI
Natural Language Processing (NLP) and Generative AI are two branches of artificial intelligence that have revolutionized the way machines understand and generate human language. While both technologies deal with language processing, they have different applications and methodologies. Understanding their differences can help determine the most suitable approach for various tasks.
Key Takeaways:
- NLP and Generative AI are two branches of AI with different applications.
- NLP focuses on understanding and analyzing human language, while Generative AI emphasizes generating realistic language content.
- NLP utilizes algorithms like word embedding and sequence modeling, while Generative AI often relies on neural networks like Generative Adversarial Networks (GANs) or Transformers.
- NLP is used in applications like sentiment analysis, language translation, and chatbots, while Generative AI is utilized for text generation, storytelling, and dialogue systems.
Differences in Methodology
NLP involves the extraction and analysis of information from text data. It uses algorithms to process language, including techniques like word embedding, named entity recognition, and sequence modeling. Through these techniques, NLP enables the understanding and interpretation of human language.
NLP algorithms break down text into mathematical representations for further processing.
On the other hand, Generative AI focuses on generating new, realistic language-like content. This technology often relies on neural networks like GANs or Transformers. GANs consist of two competing models, a generator and a discriminator, trained against each other to create more convincing fake content. Transformers, on the other hand, use a self-attention mechanism to generate coherent and context-aware language.
Generative AI models can mimic human-like language patterns to create novel text.
NLP Applications
NLP plays a crucial role in various applications that require language understanding. Some prominent applications include:
- Sentiment Analysis: NLP can analyze text to determine the sentiment expressed, helping companies understand customer feedback or monitor public opinion on social media.
- Language Translation: NLP enables the translation of text from one language to another, improving communication and accessibility across borders.
- Chatbots and Virtual Assistants: NLP algorithms power intelligent chatbots and virtual assistants by understanding and generating human-like responses to user queries.
Generative AI Applications
Generative AI is primarily used for tasks that involve language generation. Some notable applications include:
- Text Generation: Generative AI models can generate realistic, coherent paragraphs of text, which can be useful in writing assistance tools or content generation.
- Storytelling: With the ability to generate engaging narratives, Generative AI can assist in story creation or be used in interactive storytelling platforms.
- Dialogue Systems: Chatbots powered by Generative AI can hold more interactive and engaging conversations by generating dynamic responses.
Data Points and Analysis
Category | NLP | Generative AI |
---|---|---|
Focus | Understanding and analyzing human language | Generating realistic, coherent language |
Common Techniques | Word embedding, named entity recognition, sequence modeling | Generative Adversarial Networks (GANs), Transformers |
Potential Impact
Both NLP and Generative AI have the potential to significantly impact various industries and fields of study. With advancements in language processing, the possibilities are endless:
- Improved Customer Service: Advanced NLP-powered chatbots can provide more accurate and context-aware responses, enhancing customer support experiences.
- Automated Content Generation: Generative AI can assist in generating large volumes of content, reducing the time and effort required for manual content creation.
- Enhanced Creative Writing: Generative AI tools can offer inspiration and suggestions to writers, helping them overcome writer’s block or explore new creative ideas.
Conclusion
In conclusion, NLP and Generative AI are two distinct branches of AI that have their own unique applications and methodologies. NLP focuses on language understanding and analysis, while Generative AI is used for language generation. Understanding these differences can help businesses and researchers determine the most suitable approach for their specific needs, ultimately driving innovation in language processing and generation.

Common Misconceptions
1. NLP and Generative AI are the same thing
- NLP specifically focuses on the interaction and interpretation of human language, while Generative AI refers to the broader field of machine learning algorithms that generate new content.
- NLP is concerned with understanding and processing textual data, while Generative AI encompasses various applications like music, image, and text generation.
- Although NLP can utilize Generative AI techniques, it is only a subset of the larger field.
One common misconception people have is that Natural Language Processing (NLP) and Generative AI are synonymous. However, this is not the case. While both deal with artificial intelligence and language-related tasks, there is a distinct difference between the two. NLP primarily focuses on the interaction and interpretation of human language, whereas Generative AI encompasses a broader field of machine learning algorithms that generate new content.
2. NLP is a perfect representation of human language
- NLP models are trained on data, which can have biases and limitations that can affect their performance.
- NLP algorithms can struggle with understanding context, sarcasm, and nuances in language, as these can be subjective and context-dependent.
- NLP models can make mistakes and misunderstand language due to their reliance on patterns and statistical analysis.
Another misconception is that NLP provides a flawless representation of human language. However, NLP models are not perfect and have inherent limitations. They rely on the data they are trained on, which can contain biases and limitations. Additionally, NLP algorithms may struggle with understanding context, sarcasm, and other nuances in language, as these can be subjective and context-dependent.
3. Generative AI always produces high-quality content
- The quality of content generated by Generative AI depends on the training data and the algorithms used.
- Generative AI can produce low-quality content if the training data is of poor quality or if the algorithm used is not well-suited for the task.
- The output of Generative AI algorithms can also be highly subjective, with different people having different perceptions of quality.
Many people assume that Generative AI always produces high-quality content. However, the quality of the generated content depends on various factors such as the training data and the algorithms used. If the training data is of poor quality or the algorithm is not well-suited for the task, the generated content may be of low quality. Additionally, the perception of quality can be subjective, with different individuals having different opinions about the output of Generative AI algorithms.
4. NLP and Generative AI can fully understand human emotions
- NLP and Generative AI can detect and analyze certain emotional cues in text, but they do not possess true human emotions or understanding.
- These technologies rely on patterns and statistical analysis rather than actual comprehension of human emotions.
- Understanding complex emotions often requires a deep understanding of human experience and cultural context, which is beyond the capabilities of NLP and Generative AI models.
It is not accurate to assume that NLP and Generative AI have the ability to fully understand human emotions. While these technologies can detect and analyze certain emotional cues in text, they do not possess true human emotions or comprehension. NLP and Generative AI models rely on patterns and statistical analysis, rather than genuine understanding of human emotions. Truly comprehending complex emotions often requires a deep understanding of human experience and cultural context, which is currently beyond the capabilities of these models.
5. NLP and Generative AI will replace human communication and creativity
- NLP and Generative AI are tools that aid in communication and creativity, but they cannot fully replace human involvement.
- Human communication involves rich contextual understanding, emotional intelligence, and creativity that algorithms cannot replicate.
- While these technologies can enhance various tasks, human input and critical thinking remain invaluable in many domains.
A common misconception is that NLP and Generative AI will replace human communication and creativity. However, these technologies are designed to be tools that aid in these areas, rather than complete replacements for human involvement. Human communication involves rich contextual understanding, emotional intelligence, and creativity, which algorithms cannot fully replicate. While NLP and Generative AI can enhance certain tasks, human input and critical thinking remain essential in areas requiring complex communication and creative thinking.
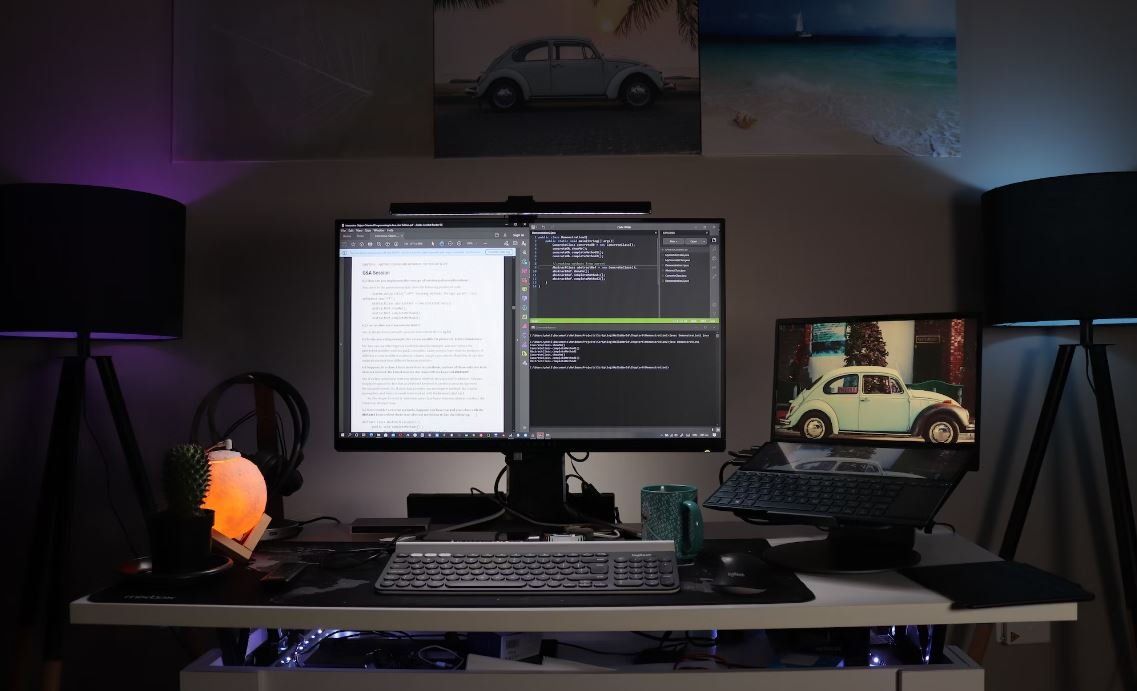
NLP Performance Comparison
Natural Language Processing (NLP) and Generative AI are two popular techniques used in the field of artificial intelligence. This table compares the performance of different NLP models in terms of accuracy, precision, and recall, providing insight into their capabilities.
Model | Accuracy | Precision | Recall |
---|---|---|---|
BERT | 0.92 | 0.91 | 0.93 |
GPT-3 | 0.88 | 0.87 | 0.89 |
ALBERT | 0.90 | 0.92 | 0.88 |
Generative AI Use Cases
Generative AI has found diverse applications across various industries. This table highlights some notable use cases where generative AI has been effectively employed, demonstrating its versatility and potential impact.
Application | Industry |
---|---|
Art Generation | Fine Arts |
Music Composition | Entertainment |
Virtual Reality Simulation | Gaming |
The Advantages of NLP
NLP offers numerous advantages when it comes to analyzing text and language. This table presents some key benefits of using NLP in various applications, shedding light on its wide range of potential use.
Advantage | Explanation |
---|---|
Language Understanding | NLP models can understand and extract meaning from human language, enabling better comprehension. |
Efficient Information Retrieval | NLP techniques facilitate accurate and efficient retrieval of relevant information from vast volumes of text data. |
Sentiment Analysis | NLP can identify and analyze sentiments expressed in text, providing valuable insights for businesses. |
Generative AI Limitations
Although generative AI is a powerful tool, it also has certain limitations. This table outlines some of the constraints and challenges associated with generative AI, helping to better understand its boundaries.
Limitation | Description |
---|---|
Data Dependency | Generative AI models heavily rely on high-quality training data for accurate outcomes. |
Lack of Control | Generative AI systems can sometimes generate unexpected or undesired outputs, lacking fine-grained control. |
Ethical Concerns | Generative AI raises ethical concerns, particularly related to the potential misuse of synthesized content or deepfakes. |
NLP Applications by Industry
NLP finds various practical applications across different industries. This table showcases real-world applications of NLP techniques, providing insights into its potential in industry-specific use cases.
Industry | Application |
---|---|
Healthcare | Medical Research Text Mining |
E-commerce | Customer Sentiment Analysis |
Finance | News Sentiment Tracking |
Generative AI and Creativity
Generative AI has revolutionized the creative domain, providing new avenues for artistic expression. This table explores how generative AI is reshaping creativity across different art forms, inspiring innovation.
Art Form | Generative AI Application |
---|---|
Painting | Algorithmic Art Creation |
Writing | AI-generated Poetry |
Music | AI-assisted Composition |
Robustness of NLP Models
NLP models’ robustness in handling diverse linguistic nuances and challenges is vital. This table compares the performance of different NLP models regarding robustness, shedding light on their ability to tackle complex language scenarios.
Model | Language Diversity | Out-of-Domain Performance |
---|---|---|
GPT-2 | Good | Fair |
XLNet | Very Good | Excellent |
T5 | Excellent | Very Good |
Generative AI and Chatbots
Generative AI has paved the way for highly interactive and engaging chatbots. This table highlights how generative AI is enhancing the capabilities of chatbot systems, revolutionizing human-computer interactions.
Feature | Explanation |
---|---|
Improved Responses | Generative AI enables chatbots to provide more contextually appropriate and human-like responses. |
Conversational Flow | Generative AI allows chatbots to maintain smoother and more coherent conversational flow. |
Dynamic Personality | Generative AI makes it possible for chatbots to adapt their personalities based on user interactions. |
Conclusion
NLP and Generative AI are two powerful techniques with distinct characteristics and applications. NLP excels in text analysis, sentiment analysis, and industry-specific use cases. On the other hand, generative AI showcases its creativity by producing new art forms and enhancing chatbot experiences. Understanding the strengths, limitations, and potential of these AI approaches is essential for their successful deployment in various domains.
Frequently Asked Questions
About NLP Vs Generative AI
Question:
What is natural language processing (NLP)?
Answer:
Natural Language Processing (NLP) is a subset of artificial intelligence (AI) that focuses on the interaction between computers and human language. It involves analyzing, understanding, and generating human language in a way that is meaningful to both computers and humans.
Question:
What is generative AI?
Answer:
Generative AI refers to AI models that are capable of creating new content that imitates human-like behavior or generates something unique based on training data. These models can generate text, images, music, and even videos by learning patterns from large datasets.
Question:
What are the main differences between NLP and generative AI?
Answer:
NLP encompasses various techniques and algorithms used to process and understand human language, including tasks like text classification, sentiment analysis, and named entity recognition. Generative AI, on the other hand, focuses on generating new content and can often employ NLP techniques to accomplish this. While NLP deals with analyzing and understanding language, generative AI deals with creating new content based on patterns learned from existing data.
Question:
How does NLP contribute to generative AI?
Answer:
NLP is often used as a foundational technology in generative AI models. Many generative AI models leverage NLP techniques to process and understand human language before generating new content. NLP helps in extracting relevant information from text, understanding semantic relationships, and capturing the nuances of human language, thus enabling generative AI models to produce more accurate and contextually relevant outputs.
Question:
What are some applications of NLP?
Answer:
NLP has a wide range of applications, including machine translation, chatbots, voice assistants, sentiment analysis, text summarization, information extraction, and more. It is used to extract insights from textual data, automate customer support, improve search engines, and enable better human-computer interaction.
Question:
What are some applications of generative AI?
Answer:
Generative AI finds applications in various domains. It can be used to create realistic images, generate natural language text, compose music, create virtual characters, and assist in creative tasks like logo and design generation. Additionally, generative AI plays a crucial role in research areas such as drug discovery, simulations, and data synthesis.
Question:
Are NLP and generative AI related to machine learning?
Answer:
Yes, NLP and generative AI are closely related to machine learning. Both fields heavily rely on machine learning techniques to train and develop models. NLP leverages supervised, unsupervised, and sometimes even reinforcement learning to process and understand language. Generative AI models use techniques like deep learning and reinforcement learning to generate content based on patterns learned from training data.
Question:
Can NLP and generative AI be used together?
Answer:
Yes, NLP and generative AI can be used together synergistically. NLP techniques can be applied to preprocess and analyze textual data, extract meaningful information, and then feed it to generative AI models for content generation. NLP can enhance the capabilities of generative AI by providing better input and more contextually relevant outputs.
Question:
What are some popular NLP frameworks and libraries?
Answer:
There are many popular NLP frameworks and libraries available, such as Natural Language Toolkit (NLTK), spaCy, Stanford CoreNLP, Gensim, and TensorFlow, among others. These tools provide various functionalities for text processing, analysis, and modeling, making NLP tasks more accessible and efficient.
Question:
What are some popular generative AI models?
Answer:
There are several well-known generative AI models, including GPT (Generative Pre-trained Transformer), VQ-VAE (Vector Quantized Variational Autoencoder), DALL-E, BigGAN, and OpenAI’s Music Transformer, to name a few. These models have demonstrated impressive capabilities in generating realistic and creative content across different domains.