Robust Language Generation
Language generation has significantly advanced with the development of robust language models. These models use advanced deep learning techniques to generate human-like text and have various applications in natural language processing.
Key Takeaways:
- Robust language generation utilizes advanced deep learning techniques.
- Its applications span across various fields, particularly natural language processing.
- Language models produce human-like text.
Robust language generation involves the use of deep learning techniques to generate text that resembles a human’s writing style. These models have made significant strides in recent years, thanks to advancements in artificial intelligence and natural language processing. By analyzing a large corpus of text, language models learn patterns and can generate coherent and contextually relevant content.
One interesting aspect of robust language generation is its ability to capture linguistic nuances and produce highly authentic writing. By training on massive amounts of text data, these models can learn grammar, style, and even knowledge about specific topics.
Robust language generation has numerous practical applications. It can be used to automate content creation in areas such as news reporting, where articles can be generated based on data and facts. It can also be utilized in chatbots to provide more natural and engaging interactions with users. Language generation models are also integrated into virtual assistants, making them more conversational and capable of understanding user queries in a more sophisticated manner.
Advancements in Robust Language Generation
The advancements in robust language generation can be attributed to the continuous improvement in deep learning algorithms and the availability of vast amounts of training data. These language models have become more accurate and can generate text with higher coherence and contextuality compared to earlier generations.
An interesting development in robust language generation is the introduction of autoregressive models, such as OpenAI’s GPT-3. These models are trained by predicting the next word in a sequence, taking into consideration the previous words in a text. Autoregressive models produce highly coherent text but require sequential generation, making them computationally intensive for longer passages.
Another significant advancement is the rise of transformer-based architectures, which have greatly improved the performance of language generation models. Transformers use self-attention mechanisms to weigh the importance of different words in a sentence, allowing the model to capture long-range dependencies and generate more contextually relevant text. This has revolutionized natural language processing tasks and opened up new opportunities for robust language generation.
Data Points in Robust Language Generation
Robust language generation models are trained on large datasets to enhance their performance. Here are some interesting data points:
Model | Training Data Size |
---|---|
GPT-2 | 40 GB |
GPT-3 | 570 GB |
Ctrl | 140 GB |
The table above showcases the training data sizes for some popular language generation models. As the training data size increases, the models can capture a wider range of language patterns and produce more coherent and contextually relevant text.
Robust language generation models can generate text with varying levels of content control. Models like Ctrl allow users to specify the desired content and style, making them suitable for specific use cases, such as technical writing or creative storytelling.
Future Implications
Robust language generation holds immense potential for further advancements and applications. As models continue to improve, we can expect:
- Enhanced content generation capabilities across different domains.
- Improved natural language understanding in virtual assistants and chatbots.
- Enhanced automated translation and language synthesis.
The future of language generation is promising, with continued research and development pushing the boundaries of what these models can achieve.
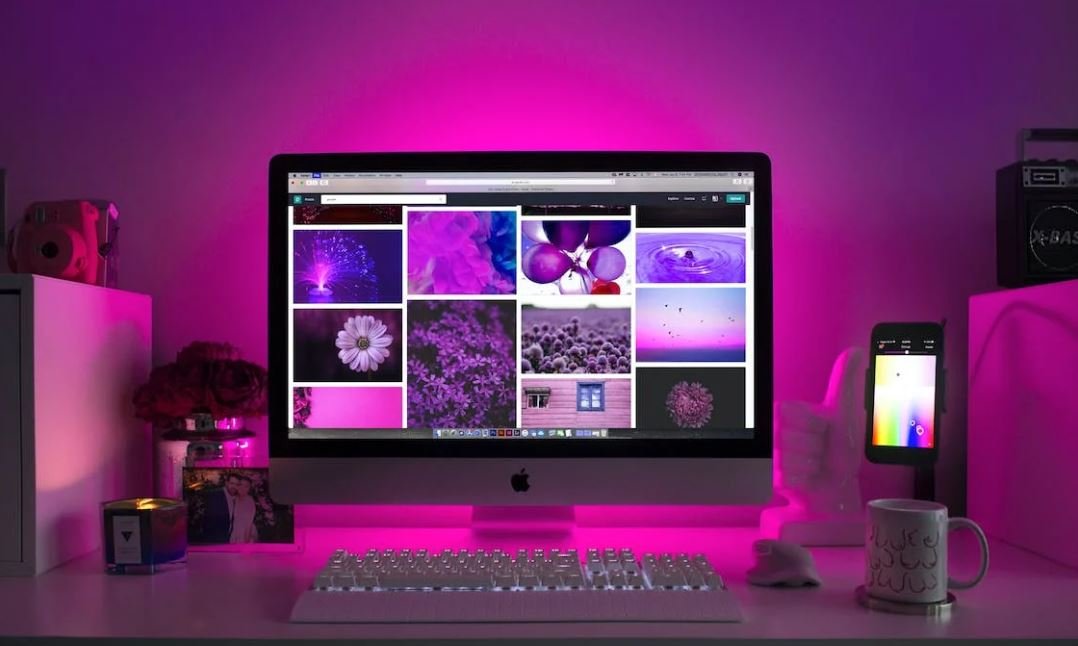
Common Misconceptions
Paragraph 1: Robust Language Generation
Robust Language Generation is often misunderstood, leading to various misconceptions about its capabilities and limitations. One common misconception is that Robust Language Generation can perfectly mimic human language. However, while it can generate coherent and natural-sounding text, it is still not on par with human-level language generation.
- Robust Language Generation can produce human-like text.
- It is not capable of generating text indistinguishable from that written by a human.
- RLG relies on pre-existing data and algorithms to generate text.
Paragraph 2: Training Data Quality
Another common misconception is that the quality of training data has no impact on the performance of Robust Language Generation. In reality, the quality of training data significantly affects the output of the model. If the training data is biased, incomplete, or contains errors, the generated text may also contain similar issues.
- The quality of training data impacts the performance of Robust Language Generation.
- Biased or incomplete training data can cause biased or inaccurate output.
- Careful selection and preprocessing of training data is crucial for robust language generation.
Paragraph 3: Universality of Robust Language Generation
There is a misconception that Robust Language Generation models can generate equally accurate and coherent text across all domains and topics. In reality, the performance of these models may vary depending on the domain-specific nature of the training data. Models trained on a specific domain may struggle to generate accurate and appropriate responses in unfamiliar domains.
- Robust Language Generation models perform differently across different domains.
- Models trained on a specific domain may not perform well in unfamiliar domains.
- Domain-specific fine-tuning can improve the performance in targeted areas.
Paragraph 4: Ethical Considerations
Many people hold the misconception that Robust Language Generation models have perfect understanding and knowledge of ethical considerations. However, these models are not inherently ethical and can generate biased, offensive, or harmful content if not explicitly guided and controlled.
- Robust Language Generation models can generate unethical or biased content if not guided.
- Explicitly setting ethical guidelines and controlling the output is necessary.
- Ongoing monitoring and feedback loops can help address potential ethical issues.
Paragraph 5: Human-Level Intelligence
Some individuals mistakenly believe that Robust Language Generation models possess human-level intelligence. While they can produce impressive output, these models lack true understanding, consciousness, and reasoning abilities that humans possess.
- Robust Language Generation models do not possess human-level intelligence.
- Models lack true understanding, consciousness, and reasoning abilities.
- They act based on patterns and statistical learning rather than genuine comprehension.
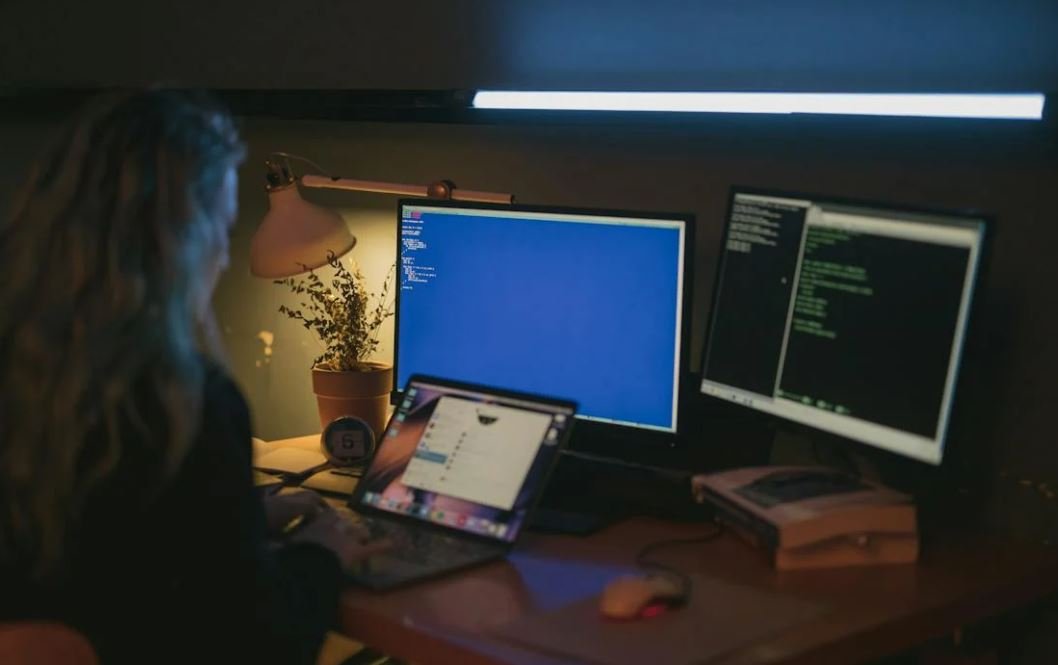
Introduction
Robust language generation is a field of study that focuses on building systems capable of generating human-like text. These systems have gained significant attention in recent years due to advances in natural language processing and machine learning. In this article, we will explore various aspects of robust language generation using informative and captivating tables.
Table 1: The Most Common Languages in the World
Language is an essential means of communication across the globe. Here, we present the top ten most spoken languages worldwide, based on the number of native speakers.
| Language | Number of Native Speakers |
|—————|————————–|
| Mandarin | 918 million |
| Spanish | 460 million |
| English | 379 million |
| Hindi | 341 million |
| Bengali | 228 million |
| Portuguese | 221 million |
| Russian | 154 million |
| Japanese | 128 million |
| Punjabi | 92 million |
| German | 92 million |
Table 2: Internet Users by Continent
The internet has revolutionized the way people communicate and access information. Let’s take a look at the number of internet users by continent.
| Continent | Number of Internet Users (in millions) |
|———–|—————————————|
| Asia | 2,537 |
| Europe | 727 |
| Africa | 555 |
| North America | 336 |
| South America | 397 |
| Australia | 25 |
Table 3: The Most Visited Countries by Tourists
International tourism plays a vital role in the economies of many countries. Here, we highlight the most visited destinations worldwide.
| Country | Number of International Tourists (in millions) |
|—————-|———————————————-|
| France | 89.4 |
| Spain | 82.8 |
| United States | 79.6 |
| China | 62.9 |
| Italy | 50.8 |
| Turkey | 45.8 |
| Mexico | 41.4 |
| Germany | 38.9 |
| Thailand | 38.2 |
| United Kingdom | 37.7 |
Table 4: World’s Largest Tech Companies by Revenue
The tech industry has experienced rapid growth, with several companies becoming leaders in terms of revenue. Check out some of the top tech giants worldwide.
| Company | Revenue (in billions of USD) |
|————————|——————————|
| Apple | 347.1 |
| Samsung Electronics | 193.2 |
| Amazon | 386.1 |
| Microsoft | 168.1 |
| Alphabet (Google) | 182.5 |
| Huawei Technologies | 142.9 |
| Facebook | 70.7 |
| Intel | 72.0 |
| IBM | 73.6 |
| Cisco Systems | 49.3 |
Table 5: The World’s Tallest Buildings
The architectural achievements that mark our cities’ skylines are awe-inspiring. Let’s explore some of the tallest structures in the world.
| Building | Height (in meters) |
|—————————–|——————–|
| Burj Khalifa | 828 |
| Shanghai Tower | 632 |
| Abraj Al-Bait Clock Tower | 601 |
| Ping An Finance Center | 599 |
| Lotte World Tower | 555 |
| One World Trade Center | 541 |
| Guangzhou CTF Finance Centre| 530 |
| Tianjin CTF Finance Centre | 530 |
| CITIC Tower (China Zun) | 528 |
| TAIPEI 101 | 508 |
Table 6: Nobel Prize Laureates by Country
The Nobel Prize is one of the most prestigious awards in various categories, recognizing outstanding contributions. Let’s see which countries have produced the most Nobel Prize winners.
| Country | Number of Nobel Prize Laureates |
|————–|——————————–|
| United States| 391 |
| United Kingdom| 132 |
| Germany | 107 |
| France | 69 |
| Sweden | 32 |
| Japan | 29 |
| Russia | 24 |
| Canada | 23 |
| Australia | 17 |
| Netherlands | 16 |
Table 7: The Twelve Zodiac Signs
Zodiac signs are widely associated with astrology and are believed to influence personality traits and behaviors. Discover the twelve zodiac signs along with their associated dates.
| Zodiac Sign | Dates |
|—————|——————|
| Aries | March 21 – April 19 |
| Taurus | April 20 – May 20 |
| Gemini | May 21 – June 20 |
| Cancer | June 21 – July 22 |
| Leo | July 23 – August 22 |
| Virgo | August 23 – September 22|
| Libra | September 23 – October 22|
| Scorpio | October 23 – November 21|
| Sagittarius | November 22 – December 21|
| Capricorn | December 22 – January 19|
| Aquarius | January 20 – February 18|
| Pisces | February 19 – March 20 |
Table 8: FIFA World Cup Winners
The FIFA World Cup is the most prestigious tournament in international soccer. Here, we present the countries that have won this esteemed competition.
| Country | Number of Titles |
|————–|——————|
| Brazil | 5 |
| Germany | 4 |
| Italy | 4 |
| Argentina | 2 |
| Uruguay | 2 |
| France | 2 |
| England | 1 |
| Spain | 1 |
Table 9: Highest Grossing Films of All Time
The film industry captivates audiences worldwide and has produced numerous box office successes. Let’s take a look at the highest grossing films to date.
| Film | Box Office Revenue (in billions of USD) |
|————————————|—————————————–|
| Avengers: Endgame | 2.798 |
| Avatar | 2.790 |
| Titanic | 2.194 |
| Star Wars: The Force Awakens | 2.068 |
| Avengers: Infinity War | 2.048 |
| Jurassic World | 1.671 |
| The Lion King | 1.656 |
| The Avengers | 1.518 |
| Furious 7 | 1.516 |
| Avengers: Age of Ultron | 1.402 |
Table 10: Olympic Games Host Cities
The Olympic Games have a long-standing tradition of bringing athletes from around the world together in the spirit of competition. Let’s explore the cities that have hosted this remarkable event.
| Games | Host City | Country |
|————-|——————|————–|
| 1896 | Athens | Greece |
| 1900 | Paris | France |
| 1904 | St. Louis | United States|
| 1908 | London | United Kingdom|
| 1912 | Stockholm | Sweden |
| 1920 | Antwerp | Belgium |
| 1924 | Paris | France |
| 1928 | Amsterdam | Netherlands |
| 1932 | Los Angeles | United States|
| 1936 | Berlin | Germany |
| 1948 | London | United Kingdom|
Robust language generation has revolutionized the way we interact with machines, from language assistants to automated content generation. This article showcased various intriguing tables, ranging from linguistic and cultural statistics to technological and entertainment achievements. The information presented in these tables exemplifies the vast world of knowledge made accessible through robust language generation.
Robust Language Generation
What is robust language generation?
Robust language generation is a field in natural language processing (NLP) that focuses on generating coherent and contextually appropriate text using machines. It involves developing algorithms and models that can understand and generate human-like language for various applications such as chatbots, virtual assistants, and even creative writing.
How does robust language generation work?
Robust language generation involves combining techniques from machine learning, deep learning, and NLP to develop models that can generate text. These models are trained on large amounts of data to learn the patterns and structures of human language. They can then generate text based on input prompts or in response to specific queries, using probabilistic methods or more advanced techniques like transformers.
What are the applications of robust language generation?
Robust language generation has diverse applications. It can be used to build chatbots for customer support, virtual assistants for personalized interactions, content generation for creative writing or news articles, and even in language translation. It can contribute to improving user experiences, automating certain tasks, and enabling better human-machine communication.
What are the challenges in robust language generation?
Robust language generation faces several challenges, including context understanding, maintaining coherence and relevance, avoiding biases or offensive content, and handling different writing styles or tones. Additionally, generating long and complex text that retains human-like qualities can still be a significant challenge for existing models.
What are some popular algorithms or models in robust language generation?
There are several popular algorithms and models used in robust language generation. These include recurrent neural networks (RNNs), long short-term memory (LSTM) networks, generative adversarial networks (GANs), and transformer models (such as GPT, BERT, or T5). These models have shown promising results in generating coherent and contextually accurate text.
How can robust language generation benefit businesses?
Robust language generation can benefit businesses in various ways. It can automate customer support, reducing the need for manual intervention. It can also enable personalized interactions with users, providing tailored recommendations and answers. Furthermore, it can enhance content generation for marketing purposes and improve overall customer experiences through efficient communication and assistance.
What are some ethical considerations in robust language generation?
Robust language generation raises ethical considerations related to bias, privacy, and misuse. The models used in language generation can amplify existing biases present in the training data, resulting in biased outputs. Additionally, the generation of personalized content may raise privacy issues if user data is not handled properly. Ensuring responsible use and minimizing potential harm are crucial factors to consider in this field.
How can robust language generation be evaluated?
Evaluating robust language generation involves assessing various aspects such as coherence, relevance, fluency, and grammar. Human evaluation, where human judges rate or rank the generated text, is often used as a benchmark. Moreover, automated metrics like BLEU (Bilingual Evaluation Understudy), ROUGE (Recall-Oriented Understudy for Gisting Evaluation), and perplexity can provide quantitative measures of the model’s performance.
What does the future hold for robust language generation?
The future of robust language generation looks promising. Ongoing research aims to improve the quality and diversity of generated text. Models are being developed to handle nuances of different languages, writing styles, and domains. Additionally, advancements in multimodal language generation, integrating text with other modalities like images or videos, are expected to enhance the overall communication capabilities of language generation systems.