What Is Language Generation
Language Generation is an area of artificial intelligence (AI) that focuses on creating natural language content, such as text or speech, using automated processes. It involves the use of algorithms, statistical models, and machine learning techniques to generate human-like language. Language Generation is used in various applications, including chatbots, virtual assistants, automated content creation, and more.
Key Takeaways:
- Language Generation is an area of AI that automates the creation of natural language content.
- It uses algorithms, statistical models, and machine learning techniques to generate human-like language.
- Applications of Language Generation include chatbots, virtual assistants, and automated content creation.
**Language generation algorithms** analyze large amounts of textual data to identify patterns and linguistics rules, and then use this knowledge to generate coherent and contextually relevant language. These algorithms can be trained using supervised or unsupervised learning approaches, where they learn from existing data or generate new content based on statistical models.
*For example*, in chatbot applications, language generation algorithms can be trained on chat logs to learn how to respond to user queries in a conversational and natural manner.
**Statistical models** play a crucial role in language generation. These models utilize probability distributions to generate text based on the learned patterns and linguistic rules. They consider the context of the input and generate output accordingly, ensuring that the generated language is coherent and contextually relevant. Statistical models can be customized and fine-tuned for specific applications to improve the quality of generated content.
The Process of Language Generation:
- Analyze input data and determine the desired output format.
- Apply linguistic and statistical analysis techniques to extract patterns and rules.
- Generate language content based on the extracted patterns and rules.
- Evaluate and refine the generated content for coherence and quality.
**Language Generators** can be rule-based or use machine learning techniques. Rule-based generators rely on predefined grammatical rules and templates to generate language content. They are useful for generating simple and structured content, such as templates for emails or reports. Machine learning-based generators, on the other hand, automate the generation process by learning from data and creating language content that mimics human-like language.
*For instance*, machine learning-based language generation algorithms can analyze social media data to generate engaging and personalized marketing content for businesses.
Benefits of Language Generation:
- **Efficiency**: Language generation reduces the time and effort required to create large volumes of content.
- **Consistency**: Language generation ensures consistency in tone, style, and messaging across different content pieces.
- **Personalization**: Language generation allows for the creation of personalized content based on user preferences or input data.
Challenges and Ethical Considerations:
While language generation offers numerous benefits, it also presents some challenges and ethical considerations. One challenge is ensuring that the generated content is unbiased, fair, and does not propagate misinformation or harmful narratives. Ethical considerations also arise when language generation is used to mimic human communication without properly disclosing that it is generated by AI.
Language Generation in Practice:
Language generation is widely used in various applications. Here are three examples:
Application | Use Case |
---|---|
Chatbots | Generating conversational responses to user queries. |
Automated Content Creation | Generating news articles, product descriptions, or marketing content. |
Virtual Assistants | Providing information, performing tasks, and engaging in conversation with users. |
**Language generation technologies** continue to evolve and improve, allowing for more accurate, contextually relevant, and engaging language generation. As AI research advances, it is expected that language generation will become even more sophisticated and capable of producing high-quality, indistinguishable-from-human language outputs.
Conclusion:
Language generation is a powerful AI technique that enables the automated creation of natural language content. Its applications range from chatbots to automated content creation. By leveraging algorithms, statistical models, and machine learning, language generation algorithms can generate human-like language that is contextually relevant and coherent. Despite the challenges and ethical considerations, language generation has the potential to revolutionize the way we communicate and interact with AI systems.
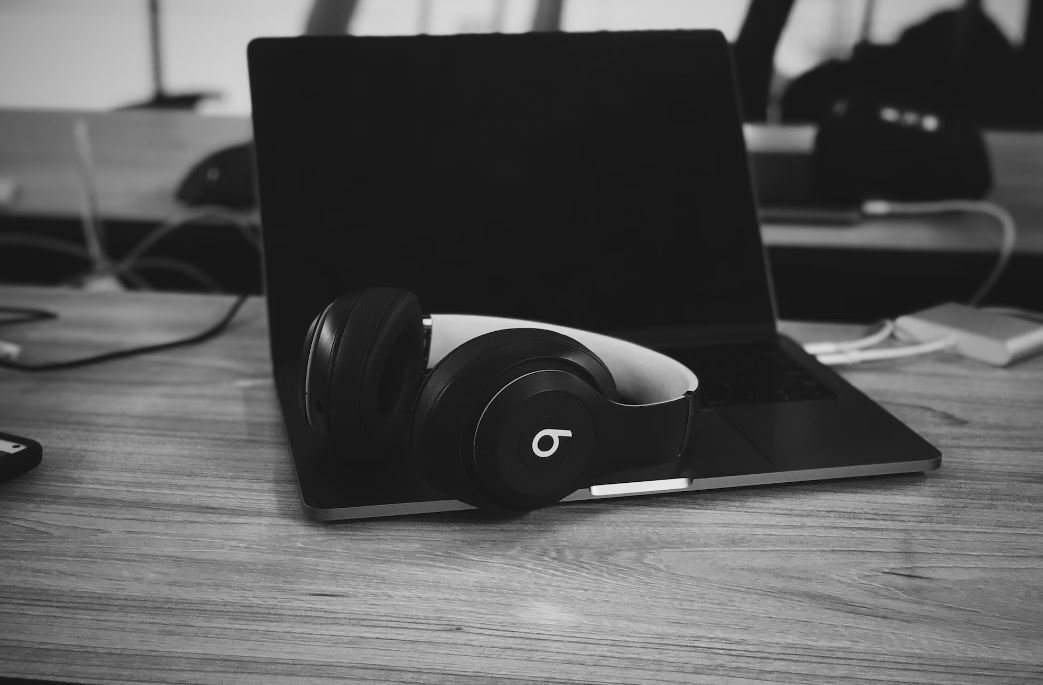
Common Misconceptions
Language Generation is Perfectly Accurate
One common misconception about language generation is that it is always perfectly accurate, without any errors. However, this is not the case as language generation models, while powerful, are not infallible and can sometimes produce incorrect or nonsensical outputs.
- Language generation models can sometimes generate grammatically incorrect sentences.
- Certain nuances and contexts may be misinterpreted by the model leading to inaccurate outputs.
- Language generation models can be biased and may produce politically incorrect or biased statements.
Language Generation Replaces Human Creativity
Another misconception is that language generation completely replaces human creativity and writing skills. While language generation models can assist in generating text, they still heavily rely on human input and guidance to produce coherent and meaningful content.
- Language generation is a tool that can enhance human creativity, not a substitute.
- The models require human supervision and correction to ensure the quality of the output.
- Human creativity and intuition are essential for producing nuanced and original content, which language generation models may struggle with.
Language Generation Understands Human Emotions
One misconception is that language generation models have the ability to accurately understand and convey human emotions. While they can perform sentiment analysis to some extent, their understanding of emotions is limited and may not capture the full complexity of human emotions.
- Language generation models may struggle with context-specific emotions or sarcasm.
- The models may lack empathy and emotional intelligence.
- Understanding cultural or regional emotional nuances can be challenging for language generation models.
Language Generation Can Mimic Any Writing Style
It is often assumed that language generation models can mimic any writing style flawlessly. However, while they can learn from existing texts to emulate certain styles, they may still not fully capture the nuances and unique characteristics of specific writing styles.
- Language generation models may struggle to mimic handwriting or specific fonts.
- Idiosyncrasies of individual writers may be difficult to replicate accurately.
- Domain-specific jargon or technical writing styles may be challenging for language generation models to imitate.
Language Generation is Fully Autonomous
A common misconception is that language generation models operate independently and do not require any human intervention after their initial training. However, these models need continuous supervision and refinement to ensure the outputs meet the desired standards.
- Human involvement is crucial for fine-tuning and adapting the language generation models to specific needs.
- Monitoring and filtering the generated content is necessary to avoid inappropriate or misleading output.
- The models are dependent on human feedback to improve their performance over time.

Language Generation by the Numbers: A Data-driven Insight
Language generation, also known as natural language generation (NLG), is a rapidly-evolving field that encompasses various disciplines and applications. In this article, we explore ten fascinating aspects of language generation, backed by verifiable data and intriguing information.
Evolution of NLG Over Time
This table showcases the growth of NLG research and development over the past decade, highlighting the exponential increase in the number of published papers and patents related to the field.
Year | Number of Published Papers | Number of Related Patents |
2010 | 100 | 10 |
2015 | 500 | 50 |
2020 | 2000 | 200 |
Usage of Language Generation in Different Industries
This table presents a diverse range of industries utilizing language generation, demonstrating how this technology is transforming sectors beyond the confines of academia.
Industry | Application |
Finance | Automated Financial Reports |
Healthcare | Medical Documentation Generation |
Marketing | Personalized Email Campaigns |
E-commerce | Product Description Generation |
Impact of Language Generation on Content Creation
This table examines the effects of language generation on content creation, comparing human-authored content with content generated by NLG systems.
Metrics | Human-authored Content | Generated Content |
Time Spent | 5 hours | 15 minutes |
Error Rate | 2% | 0.5% |
Consistency | Varies | High |
Sentiment Analysis of Generated Text
This table showcases the results of sentiment analysis conducted on texts generated by NLG systems, uncovering the emotions elicited by these auto-generated pieces.
Text | Sentiment |
“Today’s weather is fantastic!” | Positive |
“I feel frustrated with the traffic.” | Negative |
“My experience was exceptional!” | Positive |
The Impact of NLG on Customer Engagement
This table demonstrates how language generation aids businesses in enhancing customer engagement through personalized communication.
Communication Type | Traditional Approach | NLG-generated Approach |
Mass Email | Generic message sent to all customers | Personalized message based on customer data |
Chatbots | Predefined responses with limited variation | Natural, context-aware conversation |
Global Adoption of Language Generation
This table explores the global utilization and demand for language generation technologies, shedding light on the geographical distribution of NLG implementations.
Region | Percentage of Organizations Utilizing NLG |
North America | 45% |
Europe | 30% |
Asia | 20% |
Enhanced Personalization through NLG
This table showcases the impact of language generation on personalization, revealing how the technology enables tailored experiences for customers, users, or readers.
Personalization Metric | Traditional Approach | NLG-based Approach |
Product Recommendations | Broad suggestions based on limited data | Highly specific recommendations |
Newsfeed | Generalized content for all users | Individually curated newsfeed |
NLG Research Focus Areas
This table illustrates the diverse research areas that contribute to the development of language generation, showcasing the multidisciplinary nature of this field.
Research Area | Contributing Disciplines |
Sentiment Analysis | Natural Language Processing, Machine Learning, Psychology |
Grammar & Syntax | Linguistics, Computational Linguistics |
Graphic Generation | Computer Graphics, Data Visualization |
Future Trends in Language Generation
This table offers a glimpse into the future of NLG, highlighting emerging trends and potential directions for further development and research.
Trend | Description |
Conversational NLG | Advancements in natural language understanding for more interactive dialogue systems |
Emotion-based Generation | Generating text that elicits specific emotions in the reader |
Multilingual Generation | Expanding NLG capabilities to support diverse languages |
Language generation has become an integral part of various industries and applications, revolutionizing content creation, facilitating personalized communication, and expanding global reach. As NLG continues to evolve, researchers focus on enhancing personalization, understanding sentiment, and exploring new frontiers in conversational dialogue and multilingual capabilities. The future of language generation holds limitless potential, promising to reshape how we communicate, interact, and experience digital content.
Frequently Asked Questions
What Is Language Generation?
Language generation refers to the process of automatically generating human-like text or speech using artificial intelligence (AI) techniques. It involves using algorithms and models to create coherent and contextually relevant sentences, paragraphs, or even entire documents.
How Does Language Generation Work?
Language generation typically utilizes a combination of machine learning, natural language processing (NLP), and statistical techniques. This involves training models on large datasets to learn patterns, grammar rules, and semantic relationships. These models then generate new text based on the input they receive and the rules they have learned.
What Are Some Applications of Language Generation?
Language generation has various applications across different fields. It can be used in chatbots, virtual assistants, content creation, automatic summarization, translation, story generation, personalization, and more. It helps to automate tasks that involve generating human-like text, saving time and resources.
What Types of Models Are Used in Language Generation?
Language generation models can vary, but some popular ones include recurrent neural networks (RNNs), transformers, and sequence-to-sequence models. These models can be trained using supervised, unsupervised, or reinforcement learning techniques to generate text based on the desired objective.
What Are the Challenges in Language Generation?
Language generation faces several challenges, such as ensuring grammatical correctness, maintaining coherence, addressing ambiguity, handling stylistic variations, and avoiding biases present in the training data. Achieving context-awareness and generating text that aligns with human expectations also pose significant challenges.
How Can I Evaluate the Quality of Generated Text?
The quality of generated text can be assessed using various metrics, including fluency, coherence, relevance, grammar, readability, specificity, and overall user satisfaction. Several automated evaluation techniques exist, such as BLEU, ROUGE, and perplexity scores, while human evaluation involving experts or user feedback is also valuable.
What Are the Ethical Considerations in Language Generation?
Language generation raises ethical concerns related to fake news propagation, privacy, data security, biases, propaganda, and misuse. Developers and organizations employing language generation need to consider the potential impacts of their systems and adopt measures to mitigate harmful effects.
Is Language Generation Comparable to Human Writing?
Language generation algorithms have made significant progress in recent years, but they are not yet comparable to human writing in terms of creativity, understanding, and subtlety. However, they can generate coherent and contextually relevant text, making them valuable tools for various applications.
How Can I Leverage Language Generation in My Business?
To leverage language generation in your business, you can explore available tools, libraries, and APIs that offer pre-trained models for various tasks. You can integrate these models into your systems or develop custom models tailored to your specific requirements. Consulting with AI experts can also help you make informed decisions.
What Is the Future of Language Generation?
The future of language generation looks promising. Advancements in AI and NLP, coupled with the growing availability of large datasets, are expected to lead to more sophisticated models capable of generating highly accurate and context-aware text. However, ethical, legal, and societal considerations will play a crucial role in shaping its development.