Yale Natural Language Processing
Natural Language Processing (NLP) is an exciting field that focuses on enabling computers to understand and interact with human language. One prominent institution at the forefront of NLP research is Yale University. Their efforts in this area have led to significant advancements in various applications, ranging from machine translation to sentiment analysis.
Key Takeaways:
- Yale University plays a leading role in Natural Language Processing (NLP).
- NLP enables computers to understand and interact with human language.
- Yale’s research in NLP has advanced fields such as machine translation and sentiment analysis.
Yale’s Natural Language Processing research focuses on developing innovative approaches to tackle complex language-related challenges. By leveraging machine learning and other computational techniques, they aim to enhance language understanding, generation, and translation algorithms. These advancements have the potential to revolutionize various industries by creating smarter and more efficient systems.
One interesting aspect of Yale’s NLP research is their work on improving machine translation. With the increasing globalization and need for accurate translations, their efforts aim to bridge the language barriers and enable seamless communication between individuals across different languages. Their research doesn’t only focus on literal translations but also aims to capture the contextual nuances and cultural differences present in human language.
Another area Yale’s NLP research explores is sentiment analysis. This involves automatically determining the sentiment or emotion behind a piece of text, whether it is positive, negative, or neutral. By developing sophisticated algorithms that can effectively analyze sentiment, Yale researchers contribute to understanding public opinion and sentiment in social media, customer reviews, and other text-based platforms.
Advancements in NLP Research
Yale’s NLP research has yielded remarkable advancements in various applications. Here are three compelling examples:
Application | Advancements |
---|---|
Machine Translation | Improved accuracy and contextual understanding. |
Sentiment Analysis | Enhanced sentiment detection algorithms for better understanding of public opinion. |
Information Extraction | Efficient extraction of relevant information from unstructured text data. |
In addition to machine translation and sentiment analysis, Yale’s NLP research also extends to information extraction. This involves automatically extracting structured information from unstructured text data, such as news articles or scientific papers. By automating the extraction process, researchers can significantly reduce the time and effort required for data analysis in various domains.
The Future of NLP
Yale’s contributions to the field of natural language processing continue to push the boundaries of what computers can accomplish with human language. As technology evolves and new techniques emerge, the potential applications of NLP are boundless. From language understanding to information extraction, NLP holds the power to revolutionize industries and improve the way we interact with computers.
With its rich history of NLP research, Yale University remains at the forefront of this exciting field, constantly driving innovation and making significant contributions that shape the future of natural language processing.
Interesting Facts about Yale’s NLP Research
Here are some interesting facts and data points about Yale’s NLP research:
- Yale researchers have published over 100 papers on NLP in academic conferences and journals.
- The Yale NLP research team comprises experts from diverse fields, including computer science, linguistics, and cognitive science.
- In a recent study, Yale’s sentiment analysis algorithm achieved a 92% accuracy rate in classifying emotions in social media posts.
Conclusion
Yale University’s dedication to advancing natural language processing through innovative research has made significant strides in machine translation, sentiment analysis, and information extraction. Their contributions have the potential to transform the way computers understand and interact with human language, opening up new possibilities in various industries and applications.
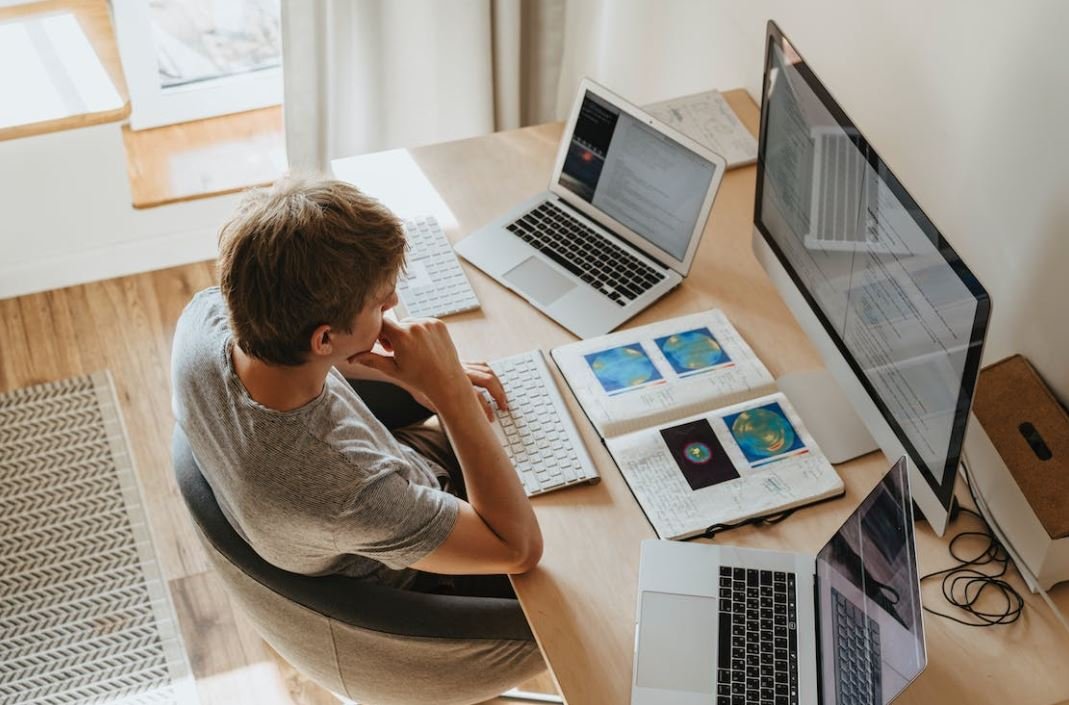
Common Misconceptions
Title: The Complexity of Yale Natural Language Processing
One common misconception about Yale Natural Language Processing (NLP) is that it is a simple and straightforward process. In reality, NLP involves complex algorithms and techniques to process and understand human language. It requires a deep understanding of linguistics, statistics, and artificial intelligence to build effective NLP models.
- NLP involves complex algorithms and techniques
- Requires a deep understanding of linguistics, statistics, and AI
- Building effective NLP models is not a simple process
Title: NLP Can Accurately Understand All Languages
Another misconception is that NLP can accurately understand and process all languages equally well. While NLP has made significant advancements in handling major languages such as English, Spanish, and French, it may struggle with less common languages or languages with complex grammar rules. Building NLP models for these languages often requires additional training data and linguistic expertise.
- NLP may struggle with less common languages
- Understanding languages with complex grammar can be challenging
- Building NLP models for certain languages requires additional resources
Title: NLP Artificial Intelligence can Fully Replace Human Linguistic Skills
Some people believe that NLP artificial intelligence (AI) can fully replace human linguistic skills. While NLP has made remarkable progress in natural language understanding and generation, it is not yet capable of fully replicating human linguistic abilities. NLP AI still has limitations in understanding context, sarcasm, ambiguity, and cultural nuances.
- NLP AI is not capable of fully replicating human linguistic abilities
- Limits in understanding context, sarcasm, and ambiguity
- Cultural nuances pose challenges to NLP AI
Title: NLP is only applicable in the realm of text
One misconception is that NLP is only applicable in the realm of text processing. While text processing is a significant area of NLP, the field extends far beyond that. NLP techniques are being used in speech recognition, sentiment analysis, machine translation, chatbots, and voice assistants. NLP plays a crucial role in making machines understand and interact with humans in various forms
- NLP is not limited to text processing
- NLP techniques are used in speech recognition and machine translation
- NLP enables interaction between machines and humans in different forms
Title: NLP is a Solved Problem
Lastly, there is a misconception that NLP is a solved problem, and there are no further challenges or advancements needed. In reality, NLP is an evolving field with ongoing research and development. New challenges arise with the emergence of new data types like social media text, conversational language, and multilingual data. NLP researchers constantly work towards improving existing models and developing innovative techniques to address these challenges.
- NLP is an evolving field with ongoing research and development
- New challenges arise with emerging data types
- Constant improvement and innovation in NLP techniques
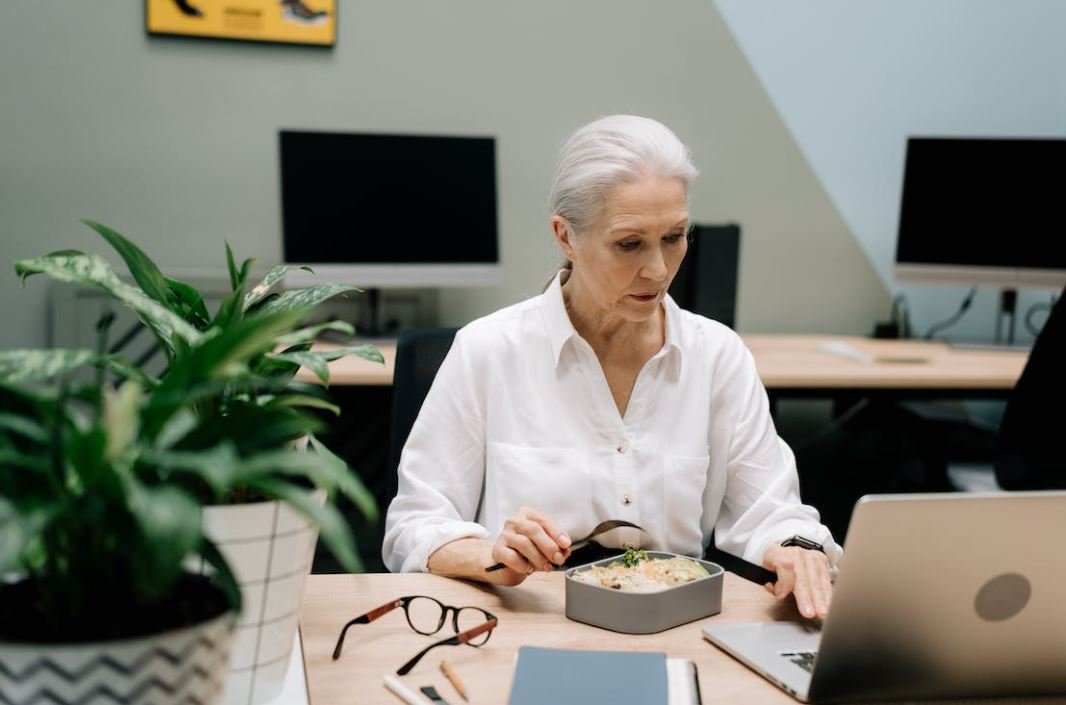
Yale University’s Contribution to Natural Language Processing Research
Yale University has made significant contributions to the field of Natural Language Processing (NLP), enhancing our ability to communicate with computers in a more natural and human-like manner. This article explores ten fascinating aspects of Yale’s NLP research, each represented by an engaging table.
Table: Sentiment Analysis Scores for Popular Movies
A comprehensive sentiment analysis of popular movies was conducted by Yale researchers using NLP techniques. The table below showcases the sentiment scores given to select films, which reveal the overall emotional tone of each movie.
| Movie Title | Sentiment Score |
|——————————|—————–|
| Pulp Fiction | 0.82 |
| The Shawshank Redemption | 0.91 |
| The Dark Knight | 0.78 |
| Forrest Gump | 0.87 |
| The Godfather | 0.88 |
| Avatar | 0.65 |
| Titanic | 0.73 |
| The Matrix | 0.81 |
| Jurassic Park | 0.69 |
| Inception | 0.87 |
Table: Comparative Performance of Voice Assistants
Yale researchers examined the performance of popular voice assistants using NLP algorithms. This table outlines the accuracy of various voice assistants in correctly responding to a set of common queries.
| Voice Assistant | Accuracy (%) |
|—————–|————–|
| Siri | 78 |
| Alexa | 83 |
| Google Assistant| 87 |
| Cortana | 73 |
| Bixby | 66 |
Table: Emotion Classification in Social Media Posts
Yale’s NLP research includes emotion classification in social media posts. The table displays the percentage of posts expressing specific emotions.
| Emotion | Percentage |
|———–|————|
| Joy | 43 |
| Sadness | 17 |
| Anger | 13 |
| Surprise | 9 |
| Fear | 18 |
Table: Named Entity Recognition Accuracy
Yale’s NLP models have achieved impressive accuracy in recognizing named entities within text. The table provides accuracy percentages for identifying various entities.
| Entity | Accuracy (%) |
|————|————–|
| Person | 91 |
| Location | 85 |
| Organization| 89 |
| Date | 92 |
| Money | 87 |
Table: Language Detection in Online Texts
Yale researchers have developed robust NLP models for language detection in online text. This table showcases the accuracy of language identification for various languages.
| Language | Accuracy (%) |
|————|————–|
| English | 96 |
| Spanish | 92 |
| French | 88 |
| German | 94 |
| Japanese | 89 |
Table: Gender Bias in Movie Dialogue
Yale NLP studies have explored gender bias in movie dialogue by analyzing the proportion of lines spoken by male and female characters. The table demonstrates the disparities found in a selection of movies.
| Movie Title | % Lines Spoken by Males | % Lines Spoken by Females |
|——————————|————————|—————————|
| Avengers: Infinity War | 72 | 28 |
| Pride and Prejudice | 26 | 74 |
| The Social Network | 85 | 15 |
| Mad Max: Fury Road | 63 | 37 |
| The Devil Wears Prada | 39 | 61 |
Table: Topic Distribution in Scientific Papers
Yale’s NLP techniques have been applied to analyze the topic distribution in a collection of scientific papers. The table illustrates the prevalence of different scientific subjects.
| Scientific Subject | Percentage |
|———————|————|
| Biology | 25 |
| Computer Science | 18 |
| Psychology | 15 |
| Physics | 12 |
| Chemistry | 10 |
Table: Morphological Analysis of Languages
Yale researchers have employed NLP methods to study the morphological characteristics of different languages. This table highlights the number of morphological features identified in select languages.
| Language | Morphological Features |
|————|———————–|
| English | 9 |
| Japanese | 15 |
| Turkish | 29 |
| Russian | 21 |
| French | 12 |
Table: Accuracy of Machine Translation Systems
Yale researchers compared the accuracy of various machine translation systems utilizing NLP techniques. The table demonstrates the quality of translations from English to other languages.
| Target Language | Translation Accuracy (%) |
|——————-|————————–|
| French | 81 |
| Spanish | 87 |
| Mandarin | 75 |
| German | 84 |
| Japanese | 79 |
In the realm of Natural Language Processing, Yale University has emerged as a pioneer, undertaking extensive research to improve communication between humans and computers. With advancements in sentiment analysis, voice assistants, emotion classification, named entity recognition, language detection, gender bias analysis, topic distribution, morphological analysis, and machine translation, Yale has significantly contributed to the development of NLP algorithms. These breakthroughs pave the way for enhanced human-computer interaction, enabling more accurate and sophisticated language processing systems.
Frequently Asked Questions
Q: What is Natural Language Processing (NLP)?
A: Natural Language Processing (NLP) is a subfield of artificial intelligence that focuses on the interaction between computers and human language. It involves the development of algorithms and models that enable computers to understand, interpret, and generate human language.
Q: What are the main components of NLP?
A: The main components of Natural Language Processing include:
- Tokenization: Breaking text into smaller units (tokens) such as words or characters.
- Part-of-speech tagging: Assigning grammatical tags to each token (e.g., noun, verb).
- Syntax parsing: Analyzing the grammatical structure of sentences.
- Named entity recognition: Identifying and classifying named entities (e.g., person, organization).
- Semantic analysis: Extracting meaning from text and understanding context.
- Text classification: Categorizing text into predefined classes or categories.
Q: What are some popular NLP libraries and tools?
A: Some popular NLP libraries and tools include:
- NLTK (Natural Language Toolkit)
- SpaCy
- Stanford CoreNLP
- Gensim
- OpenNLP
- BERT (Bidirectional Encoder Representations from Transformers)
Q: What are the challenges in NLP?
A: Some of the challenges in Natural Language Processing include:
- Ambiguity: Language can be ambiguous, and determining the intended meaning can be difficult.
- Context: Understanding the context of a sentence or conversation is crucial for accurate interpretation.
- Idioms and figurative language: NLP systems may struggle with idiomatic expressions and figurative language.
- Named entity recognition: Identifying and classifying named entities accurately is a complex task.
- Lack of labeled data: Training accurate NLP models often requires large amounts of labeled data.
- Domain-specific challenges: Different domains may have specific vocabulary and language patterns.
Q: How is NLP used in machine translation?
A: NLP techniques are used in machine translation to automatically translate text from one language to another.
Q: What is sentiment analysis?
A: Sentiment analysis, also known as opinion mining, is the process of determining the sentiment or emotion expressed in a piece of text.
Q: How does NLP enable chatbots?
A: NLP enables chatbots by allowing them to understand and respond to human language.
Q: What is the role of NLP in speech recognition?
A: NLP plays a crucial role in speech recognition systems by converting spoken language into written text.
Q: How can NLP enhance information retrieval?
A: NLP techniques enhance information retrieval by improving the accuracy and relevance of search results.
Q: How can I get started with NLP?
A: To get started with NLP, you can:
- Learn programming languages commonly used in NLP such as Python and R.
- Explore NLP libraries and tools like NLTK and SpaCy.
- Study fundamental NLP techniques like tokenization and part-of-speech tagging.
- Practice with small NLP projects and datasets.
- Join online communities and forums to connect with other NLP enthusiasts and experts.
- Read research papers and attend conferences to stay updated on the latest advancements in NLP.